This week we spent a day in NYC reviewing AWS’ AI strategy and progress with several AWS execs including Matt Wood, VP of AI at the company. We came away with a better understanding of AWS’ AI approach beyond what was laid out at re:Invent 2023. We also met separately with a senior technology leader at a large financial institution to gauge customer alignment with AWS’ narrative. While stories from both camps left us with a positive impression, the survey data shows OpenAI and Microsoft continue to hold the AI momentum lead, a position the pair usurped from AWS, which historically was first to market with cloud innovations. AWS’ strategy to take back the lead involves a multi-pronged approach within its three layer stack of infrastructure, AI tooling and up the stack applications.
In this Breaking Analysis we review the takeaways from our AI field trip to New York City. We’ll share survey data from ETR on Gen AI adoption and key barriers. We also place these in context to the recent scathing review of Microsoft’s security practices by the government’s Cyber Safety Review Board and we’ll share our view of AWS’ AI opportunities and challenges going forward.
Power Law of Gen AI Plays Out
Early last year, theCUBE Research published the Power Law of Gen AI shown below. The basic concept is that while some industries have a handful of dominant leaders and a long tail of bit players, we see the Gen AI curve differently. On the chart above we show model size on the vertical axis and domain specificity on the horizontal plane. And while a few giants like the hyperscalers will dominate the training space, a large number of use cases are emerging and will continue to do so with greater industry specialization. Furthermore, open source models and well-funded third parties will pull the torso up to the right as shown in the red line. And they will support the premise of domain specific models by helping customers balance model size, complexity, cost and best fit.
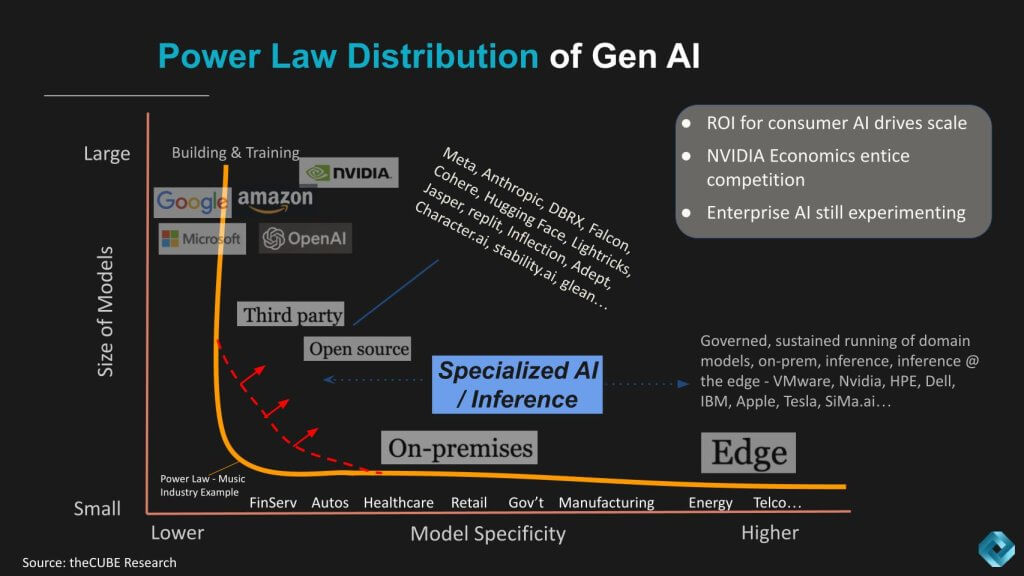
AWS’ Generative AI Stack
We weren’t able to obtain the deck Matt Wood shared with us so we’ll revert to an annotated version (by us) of a slide Adam Selipsky showed at re:Invent last year.
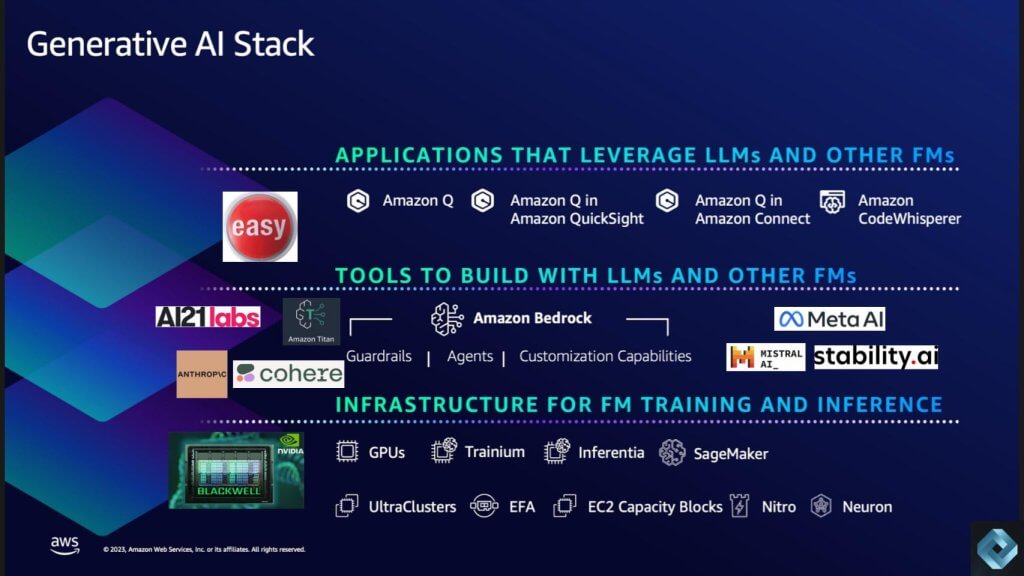
The diagram above depicts AWS’ three layered Gen AI stack comprising core infrastructure for training foundation models and doing cost effective inference. Building on top of that layer is Bedrock, a managed service providing access to tools that leverage LLMs and at the top of the stack, Q, Amazon’s effort to simplify the adoption of Gen AI with what are essentially Amazon’s version of copilots.
Infrastructure at the Core
Let’s talk about some of the key takeaways from each layer of the stack. First at the bottom layer there are three main areas of focus: 1) AWS’ history in ML and AI, particularly with SageMaker; 2) Its custom silicon expertise and 3) Compute optionality with roughly 400 instances. We’ll take these in order.
Amazon emphasized that it has been doing AI for a long time with SageMaker. SageMaker, while widely adopted and powerful, is also complex. Getting the most out of SageMaker requires an understanding of complex ML workflows, choosing the right compute instance, integration into pipelines/ IT processes and other non-trivial operations. A large proportion of AI use cases can be addressed by SageMaker. AWS in our view has an opportunity to simplify the process of using SageMaker by applying Gen AI as an orchestration layer to widen the adoption of its traditional ML tools.
In silicon, AWS has a long history developing custom chips with Graviton, Trainium and Inferentia. AWS offers so many EC2 options that can be confusing, but these options allow customers to optimize instances for workload best fit. AWS of course offers GPUs from NVIDIA and claims it was the first to ship H100’s and it will be the first to market with Blackwell, NVIDIA’s superchip.
AWS’ strategy at the core infrastructure layer is supported by key building blocks like Nitro and Elastic Fabric Adapter (EFA), to support a wide range of XPU options with security designed in from the start.
Bedrock and Foundation Model Optionality
Moving up the stack to the second block, this is where much of the attention is placed because it’s the layer that competes with OpenAI. Most of the industry was unprepared for the ChatGPT moment. AWS was no exception in our view. While it had Titan, its internal foundation model, it made the decision that offering multiple models was a better approach. A skeptical view might be this is a case of “if you can’t fix it, feature it,” however AWS’ history is to both partner and compete. Snowflake v. Redshift is a classic example where AWS serves customers and profits from the adoption of both.
Amazon Bedrock is the managed service platform by which customers access multiple foundation models and tools to ensure trusted AI. We’ve superimposed on Adam’s chart above several foundation models that AWS offers including AI21labs’ Jurassic, Amazon’s own Titan model, Anthropic Claude, perhaps the most important of the group given AWS’ $4B investment in the company. We also added Cohere, Meta’s Llama, Mistral AI with several options including its mixture of experts (MoE) model and its Mistral Large flagship and finally stability.ai’s Stable Diffusion model. And we would expect to see more models in the future including possible DBRX. As well, Amazon will be evolving its own FMs. Last November you may recall a story broke about Amazon’s Olympus which is reportedly a 2 trillion parameter model headed up by the former leader of Amazon Alexa, reporting directly to Andy Jassy.
Simplifying Gen AI Adoption with Applications
Finally the top layer is Q, an up the stack application layer designed to be the easy button with out of the box Gen AI for specific use cases. Examples today include Q for supply chain or Q for data with connectors to popular platforms like Slack and ServiceNow. Essentially think of Q as a set of Gen AI assistants that AWS is building for customers that don’t want to build their own. AWS doesn’t use the term ‘copilots’ in its marketing as that is a term Microsoft has popularized, but basically that’s how we look at Q.
Wide Gen AI Adoption – Microsoft & Open AI Dominant
The chart below shows data from the very latest ETR technology spending intentions survey of more than 1800 accounts. We got permission from ETR to publish this ahead of their Webinar for private clients. The vertical axis is spending momentum or Net Score on a platform. The horizontal axis is presence in the data set measured by the overlap within those 1,800+ accounts. The red line at 40% indicates a highly elevated spend velocity. The table insert in the bottom right shows how the dots are plotted – Net Score by N in the survey.
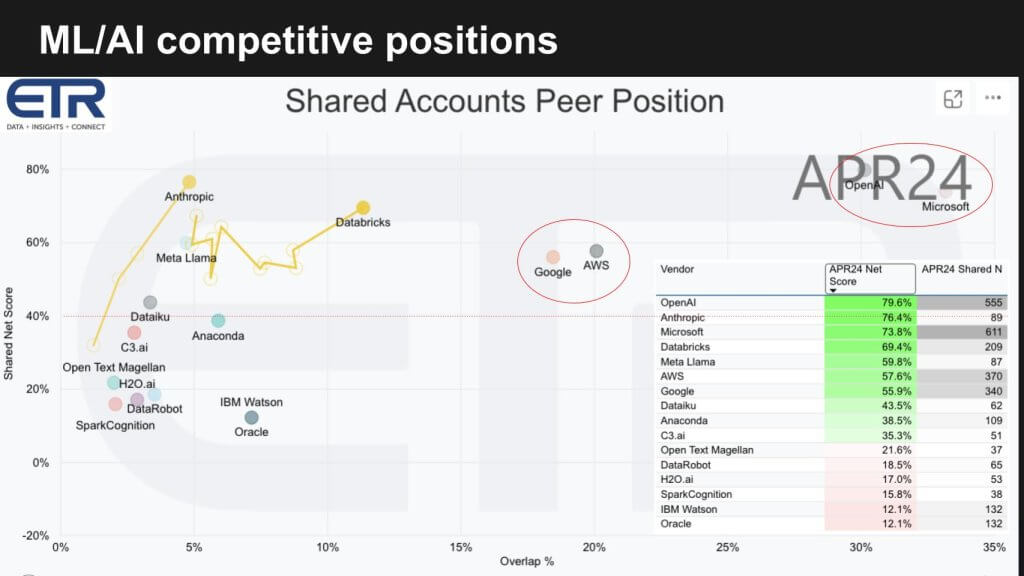
Point 1 – OpenAI and Microsoft are off the charts in terms of account penetration. Open AI has the #1 Net Score at nearly 80% and Microsoft leads with 611 responses.
Point 2 – AWS is primarily represented in the survey by SageMaker. AWS and Google within the AI sector are much closer than they are in the overall cloud segment. AWS is far ahead of Google when we show cloud account data but Google appears to be closing the gap. Data on Bedrock is not currently available in the ETR data set. Both AWS and Google have strong Net scores and a very solid presence in the data set but the compression between these two names is notable.
Point 3 – Look at the moves both Anthropic and Databricks are making in the ML/AI survey. Anthropic in particular with a net score rivaling that of OpenAI, albeit with a much much smaller N. But that is AWS’ most important LLM partner. Databricks as well is moving up and to the right. Our understanding is that ETR will be adding Snowflake in this sector. Snowflake you may recall essentially containerizes NVIDIA’s AI stack as one of their main plays in AI so it will be interesting to see how they fare in the days ahead.
Point 4 – In the January survey, Meta’s Llama was ahead of both Anthropic and Databricks on the vertical axis and it’s interesting to note the degree to which they’ve swapped positions…we’ll see if that trendline continues.
AI Tool Diversity Enables the Best Strategic Fit
We obtained the chart below after we recorded our video but wanted to share this data because it provides a more detailed and granular view than the previous chart. It breaks down the Net Score methodology. Remember, Net Score is a measure of spending velocity on a platform. It measures the percent of customers in a survey that are: 1) Adopting a platform as new; 2) Increasing spend by 6% or more; 3) Spending flat at +/- 5%; 4) Decreasing spend by 6% or worse; and 5) Churning. Net Score is calculated by subtracting 4+5 from 1+2 and it reflects the net percent of customers spending more on a platform.
Below we show the data for each of the ML/AI tools in the ETR survey.
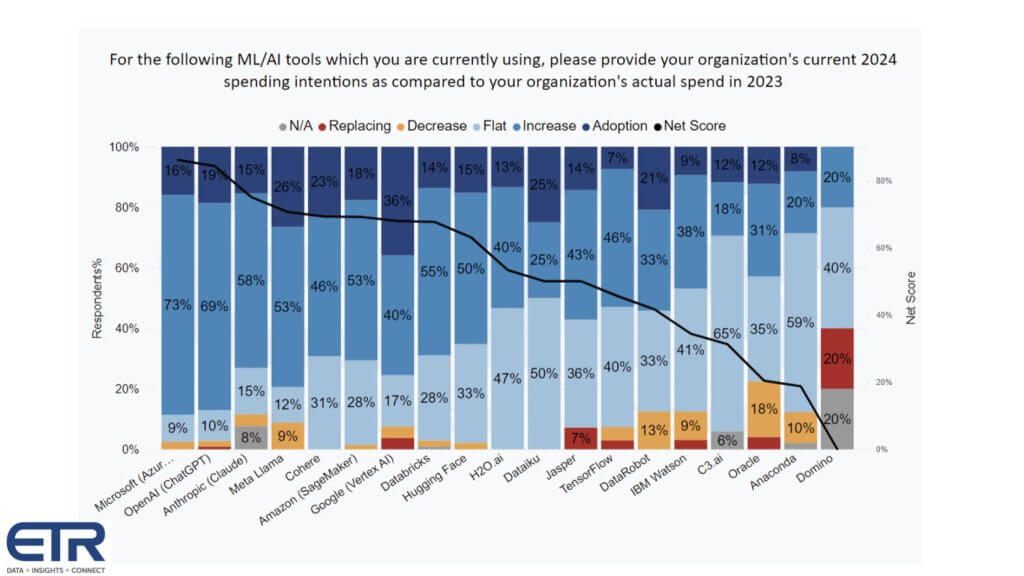
The following points are noteworthy:
- A Net Score of 40% or greater is considered highly elevated.
- The dominant scores of Microsoft and OpenAI are notable given the large N’s we showed in the previous chart.
- Anthropic’s momentum is also impressive but its presence in the survey (Ns) is 1/6th that of OpenAI.
- We don’t currently have data for Amazon Bedrock but it’s likely much of the Anthropic adoption is through AWS.
- The top tools show virtually no churn with the tiny exception of OpenAI and Google Vertex.
- The same is true for spending decreases with the exception of Llama, which is showing small portion of customers spending less.
- The top 9 tools all show the percent of customers spending more is greater than those spending flat and spending less combined – a sign of an immature market with lots of momentum.
There is much discussion in the industry regarding the eventual commoditization of LLMs. We’re still formulating our opinion on this and gathering data but anecdotal discussions with customers suggests they see value in optionality and diversity and our view is as long as innovation and ‘leapfrogging’ continue, foundation models may consolidate but commoditization is of lower probability.
Gen AI Adoption is Rapid but Risks Remain
Let’s look at some other ETR data and dig into some of the challenges associated with bringing Gen AI into production. In a March survey of almost 1,400 IT decision makers, nearly 70% said their firms have put some form of Gen AI into production. The chart below shows the 431 that have not gone into production and asks them why.
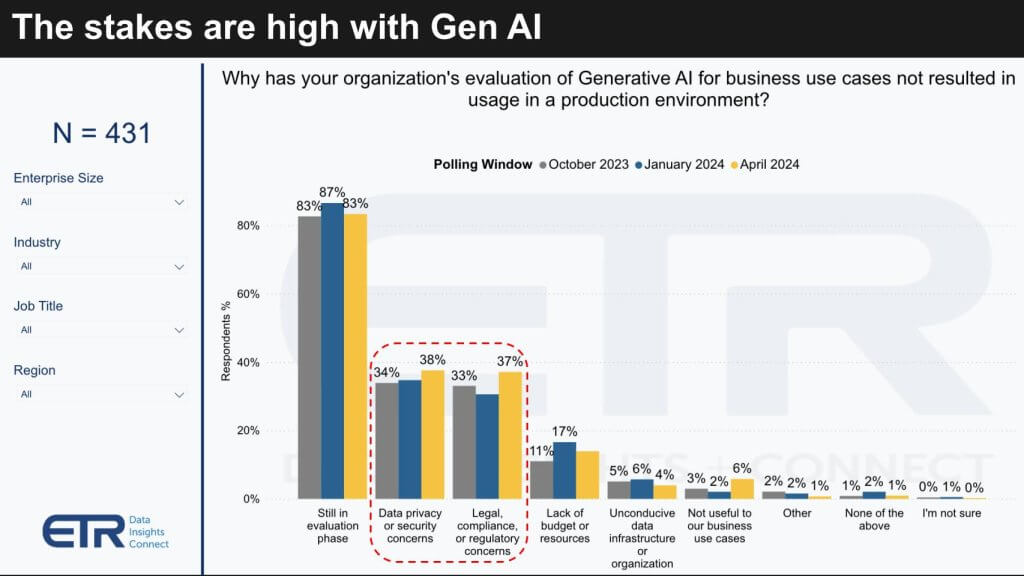
The number one reason is they’re still evaluating but the real tell is the degree to which data privacy, security, legal, regulatory and compliance concerns are barriers to adoption. This is no surprise but unlike the days of big data where many deployments went unchecked, most organizations today are being much more mindful with AI. But we believe customers have blindspots and are taking on risks that are not fully understood.
Microsoft’s Security Posture is a Major Customer Risk
Given the concerns about privacy and security one can’t help but reflect on the recent report initiated by the head of homeland security to investigate the hack on Microsoft one year ago that was traced to China. The breach compromised the accounts of key government officials, including the commerce secretary.
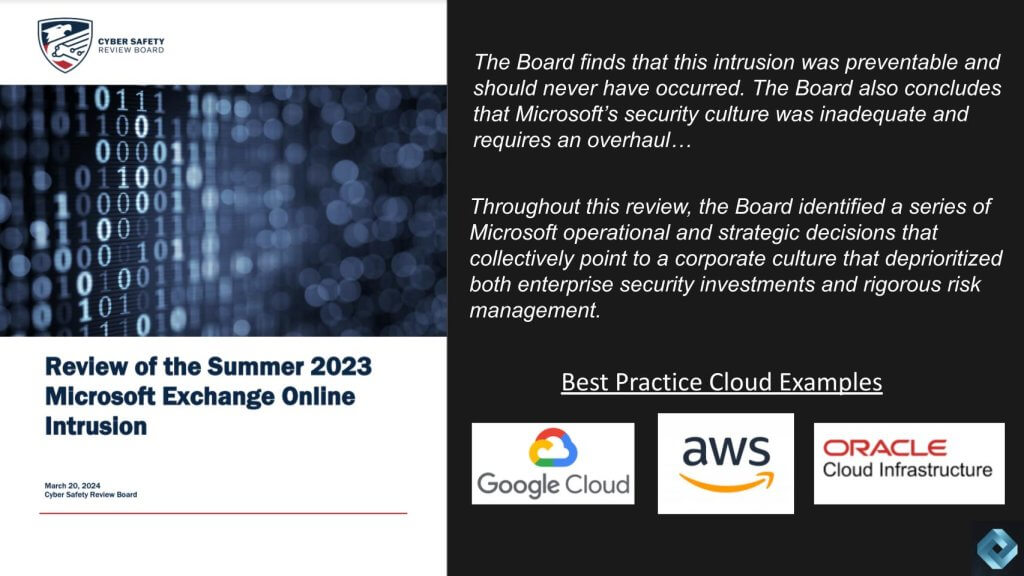
The government’s report absolutely eviscerated Microsoft for prioritizing feature development over security, using outdated security practices, failing to close known gaps and poorly communicating what happened, why it happened and how it will be addressed. This story was widely reported but it’s worth noting in the context of AI adoption. Here are a few key callouts from that report.
The Board finds that this intrusion was preventable and should never have occurred. The Board also concludes that Microsoft’s security culture was inadequate and requires an overhaul…
Throughout this review, the Board identified a series of Microsoft operational and strategic decisions that collectively point to a corporate culture that deprioritized both enterprise security investments and rigorous risk management.
The report also evaluated other cloud service providers and specifically called out Google, AWS and Oracle. The report gave specific best practice examples of how they approach security and left the reader believing that these firms have far better security in place than does Microsoft.
Why is this so relevant in the context of Gen AI? It’s because the cloud has become the first line of defense in cybersecurity. In cloud there’s a shared responsibility model that most customers understand and it appears that Microsoft is not living up to its end of the bargain.
If you’re a CEO, CIO, CISO, board member, P&L manager…and you’re a Microsoft shop, you’re relying on Microsoft to do its job. According to this report, Microsoft is failing you and putting your business at risk. This is especially concerning because of the ubiquity of Microsoft and its presence in virtually every market, and the astounding AI adoption data we shared above. Customers must begin to ask themselves if the convenience of doing business with Microsoft is exposing risks that need to be mitigated.
Satya Nadella, saved Microsoft from irrelevance when he took over from Steve Ballmer and initiated a cloud call to action. Based on this detailed report, Microsoft has violated the trust of its customers, many of whom are now putting their AI strategies in Microsoft’s hands. This is a a wake up call to business technology executives and if ignored, it could spell disaster for large swaths of customers.
Learnings From the AWS AI Briefing
Coming back to AWS… As you can see in the data, AWS is doing well, but if you believe AI is the new next thing – which we do – then: 1) the game has changed and 2) AWS has a lot of work to do.
So what are some of the themes we heard this week from AWS?
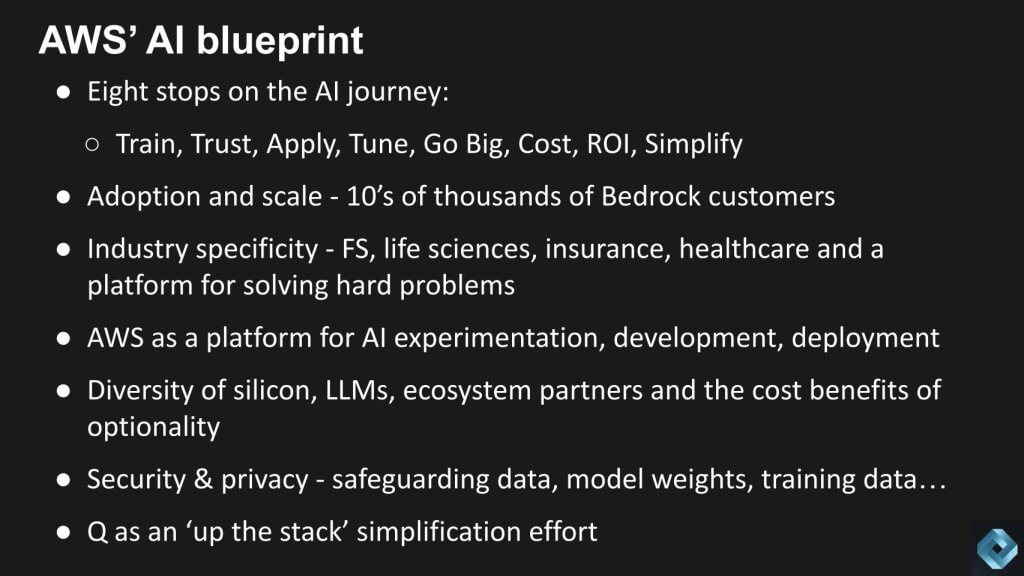
Matt Wood laid out an eight step journey they see from customer AI initiatives. These are not linear steps necessarily but they are key milestones and objectives that customers are initiating.
Step1 – Training. Let’s not spend too much time here because most customers are not doing hard core training. Rather they start with a pre-trained model from the likes of Anthropic or Mistral, etc. AWS did make the claim that most leading foundation models (other than Open AI’s) are predominantly trained on AWS. Anthropic is an obvious example but Adobe Firefly was another one that caught our attention based on last week’s Breaking Analysis.
Step 2 – IP Retention and Confidentiality. Perhaps the most important starting point. Despite that ETR data we just showed you, many folks have banned the use of OpenAI tools internally. But we know for a fact that developers for example find OpenAI tooling to be better for many use cases like code assistance. Fro example, we know devs whose company has banned the use of ChatGPT for coding but rather than use Code Whisperer (for example) they find OpenAI tooling so much better that they download the iPhone app and do it on their smartphone. This should be a concern for CISOs. Customer should be asking their AI provider if humans are reviewing results? What type of encryption is used? How is security built into managed services? How is training data protected? Can data be exfiltrated and if so how? How are accesses to data flows being fenced off from the outside world and even the cloud provider?
Step 3 – Applying AI. The goal here is to widely applying Gen AI to the entire business to drive productivity and efficiency. The reality is customer use cases are piling up. The ETR survey data tells us that 40% of customers are funding AI by stealing from other budgets. The backlog is growing and there’s lots of experimentation going on. Historically, AWS has been a great place to experiment but from the data, OpenAI and Microsoft are getting a lot of that business today. AWS’ contention is that other cloud providers are married to a limited number of models. We’re not convinced. Clearly Google wants to use its own models. Microsoft prioritizes OpenAI of course but it has added other models to its portfolio. This is one where only time will tell. In other words, does AWS have a sustainable advantage over other players with FM optionality or if it becomes an important criterion can others expand their partnerships further and neutralize any AWS advantage?
Step 4 – Consistency and Fine Tuning. Getting to consistent and fine tuned RAG models for example. Matt Wood talked about the “swiss cheese effect” that AWS is addressing. It’s a case where if a RAG has data it’s pretty good but where it doesn’t it’s like a hole in swiss cheese so the models will hallucinate. Filling those holes or avoiding them is something that AWS has worked on according to the company. And it is able to minimize poor quality outputs.
Step 5 – Solving Complex Problems. For example, getting deeper into industry problems in health care, financial services, drug discover and the like. Again these are not linear customer journeys, rather they are examples of initiatives AWS is helping customers address. Most customers today are not in the position to attack these hard problems but those industry leaders with deep pockets are in a position to do so and AWS wants to be their go to partner.
Step 6 – Lower, Predictable Costs. AWS didn’t call this cost optimization but that’s what this is. It’s an area where AWS touts its custom silicon. While competitors are now designing their own chips, as we’ve reported for years, AWS has a big head start in this regard from its Annapurna acquisition of 2015.
Step 7 – Common Successful Use Cases. The data tells us today that the most common use cases are document summarization, image creation, code assistance and basically the things we’re all doing with ChatGPT. This is relatively straightforward and if done so with protection and security it can yield fast ROI.
Step 8 – Simplification. Making AI easier for those that don’t have the resources or time to do it themselves. Amazon’s Q is designed to attack this initiative with out of the box Gen AI use case as we described earlier. We don’t have firm data on Q adoption at this point but are working on getting it.
Some other quick takeaways from the conversations:
- We met with industry experts at AWS in financial services and cross industry pros who shared numerous use cases in insurance, finance, media, health care, you name it. Right in line with the power law we discussed earlier.
- AWS is positioning itself as a platform to support scale and it has a strong track record in doing so.
- Bedrock adoption is very strong with 10’s of thousands of customers.
- And the last three on the chart above we’ve touched on a bit – silicon and LLM diversity – ecosystem partners and companies like Adobe training on AWS with products like Firefly which we covered last week.
- Security, privacy and controls.
- Up the stack applications with Q. Our belief is Q is still a work in process. Packaged apps are not AWS’ wheelhouse but Q is a start and perhaps Gen AI makes it easier for them to enter upstream.
AWS AI Going Forward
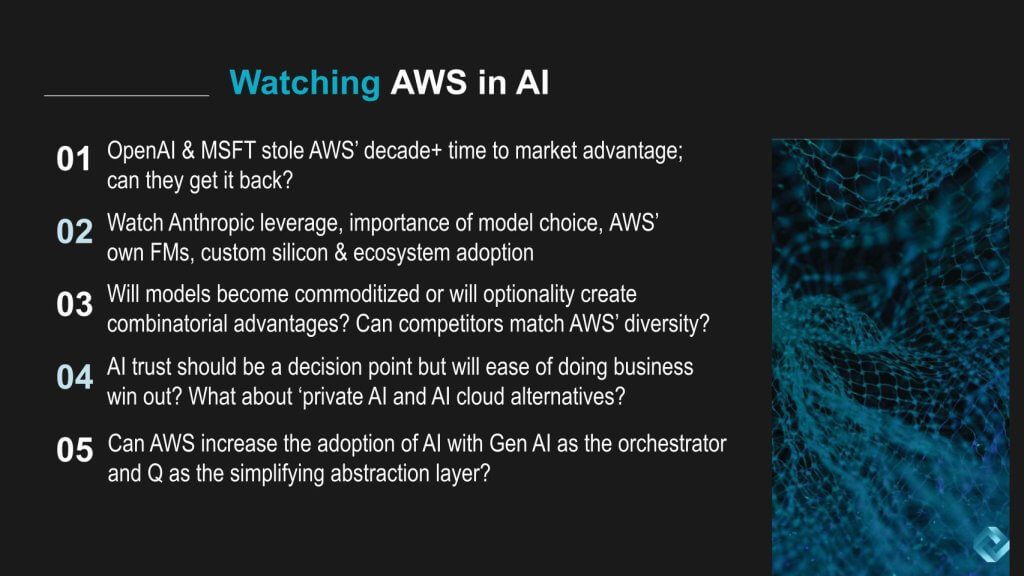
OpenAI & MSFT stole AWS’ decade+ time to market advantage; can they get it back? To do so they will try to replicate their internal innovation with ecosystem partners to offer customer choice and sell tools and infrastructure around them.
Watch for Anthropic leverage, even closing the loop back to silicon. In other words can the relationship with Anthropic make AWS’ custom chips better. And what about Olympus – watch for that capability from Amazon’s internal efforts this year. How will model choice play into AWS’ advantage and will it be sustainable?
Will models become commoditized or will optionality create combinatorial advantages? Can competitors match AWS’ diversity if optionality becomes an advantage?
AI trust should be a critical decision point but will ease of doing business win out? What about ‘private AI and AI cloud alternatives?
Speaking of GPU cloud alternatives our friends at VAST are doing very well in this space. At NVIDIA GTC we attended a lunch hosted by VAST with Genesis Cloud which was very informative. These firms are really taking off and positioning themselves as a purpose-built AI cloud to compete with the likes of AWS. So we asked VAST for a list of the top alternative clouds they’re working with, in addition to Genesis names like Core42, CoreWeave, Lambda and Nebula are raising tons of money and gaining traction. Perhaps they all won’t make it but some will to challenge the hyperscale leaders. How will that impact supply, demand and adoption dynamics.
Can AWS increase the adoption of AI with Gen AI as the orchestrator and Q as the simplifying abstraction layer? In other words can Gen AI accelerate AWS’ entry into the application space…or will its strategy continue to be enabling its customers to compete up the stack. Chances are the answer is ‘both.’
What do you think? Does AWS’ strategy resonate with you? Are you concerned about Microsoft’s security posture? Will it make you reconsider your IT bets? How important is model diversity to your business? Does it complicate things or allow you to optimize for the variety of use cases you have in your backlog?
Let us know.