Enterprises are fighting a dual mandate of operating inside a tight IT budget envelope while at the same time transforming their organization into an AI-first company. Navigating macroeconomic headwinds while driving innovation is an exciting challenge for IT decision makers. To deliver the goods, technology leaders are stealing from other budgets to fund AI, finding quick wins with fairly unspectacular use cases and in some situations swinging for the fences with ambitious AI training initiatives. These more sophisticated efforts are designed to either drive revenue from things like better advertising outcomes or solve complex human problems such as new drug discovery, cancer research, autonomous driving, etc. The reality is, sixteen months into the generative AI awakening, there’s lots of hype and tons of experimentation happening, but success in enterprise AI is far from assured.
In this week’s Breaking Analysis we dig deep into the numbers and look at the macro spending climate then drill into specific spending patterns around generative AI. We’ll look at how budgets are being funded, how Gen AI ROI expectations are changing, common use cases and LLM adoption. And we’ll close by asking the somewhat controversial question – is it cheaper to do AI in the cloud or on-prem?
The Macro Continues to Confound
First let’s look at how the sentiment on IT spending has changed in just a few short months. The chart below shows the results of twelve quarters of sampling ITDMs’ forecast of annual enterprise tech spending. The N’s of these surveys consistently range between 1,500 and 1,800 with a very high overlap (around 75%) of repeat respondents. As we exited COVID and the Fed started tightening, spending growth expectations kept decelerating and finally bottomed when the Fed stopped tightening last summer.
Rob Williams, SVP of investor relations at Dell commented on this data saying it probably tracks the two year treasury yield and he’s absolutely right – it’s basically inversely proportional to that metric meaning the rise in 2 year yields corresponds to a deceleration in IT spend expectations.
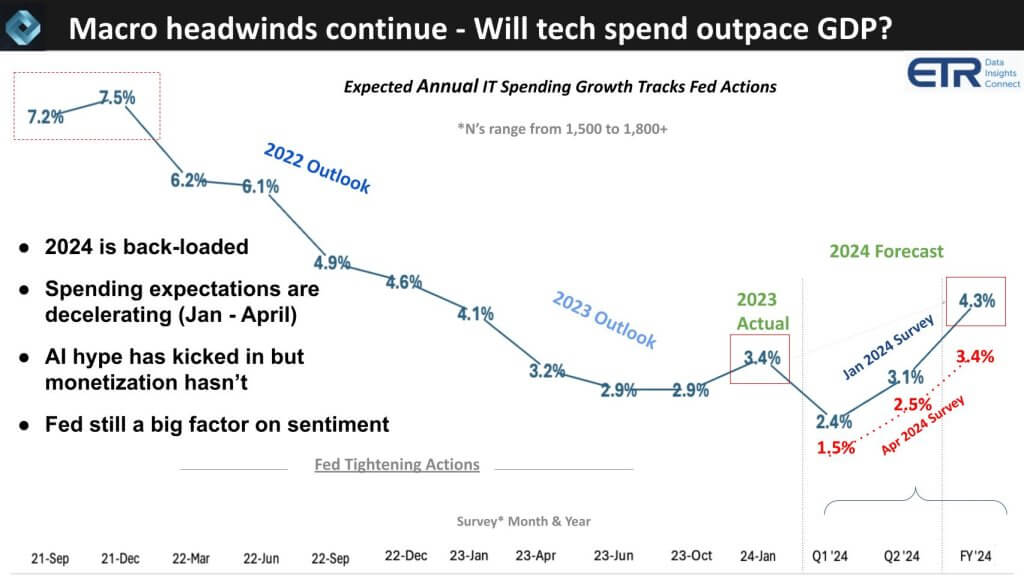
The notable points here are:
- IT spending grew about 3.4% last year and in the January ’24 survey the expectation was an accelerated growth rate to 4.3%.
- As we cautioned at the time the quarterly growth expectations were back loaded.
- Sure enough, as Williams surmised, when you track the recent two year movement it’s popped up again as the higher for longer theme reared its head. As you see in the red above, the expectations have dropped from 4.3% growth to 3.4% with Q1 and Q2 forecasts decelerating as well.
We would expect IT spending to grow at least 1-2 points faster than GDP. With the new CPI numbers this past week we could see GDP growth expectations approach these tech spending forecasts. With the AI hype, you would like to see tech spending maintain a healthy growth rate above GDP. The reality is while AI hype is in full swing, AI monetization isn’t and so the Fed remains a factor in determining sentiment and that is rippling into IT budgets.
Many Gen AI Projects are Funded by Shifting Budgets
Let’s look at how is AI being funded in many organizations.
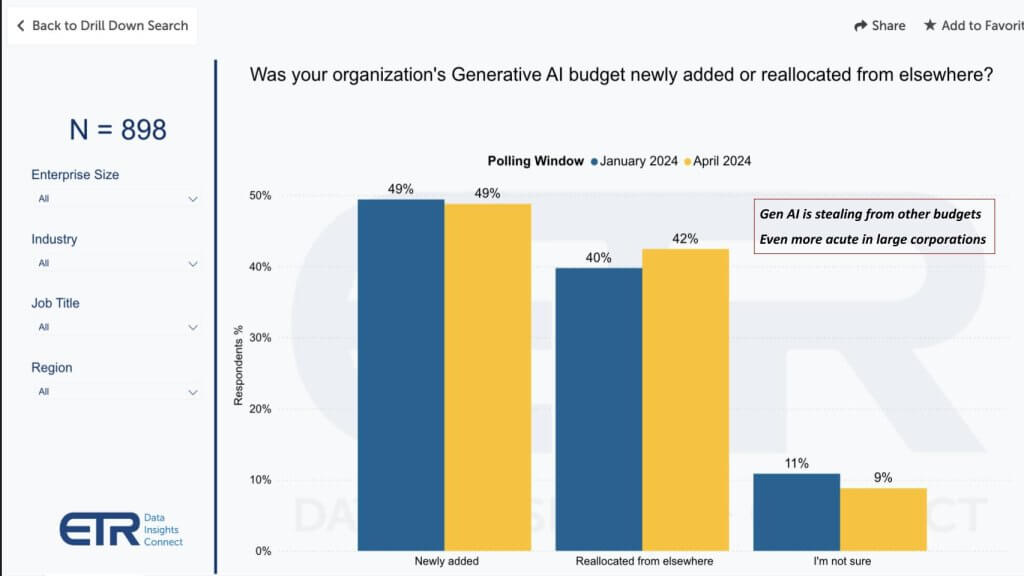
As we’ve indicated in previous episodes, this data above shows us that 42% of customers in a survey N of 898 say they’re stealing from other budgets to fund Gen AI projects. When we dig into that data, money is coming from business apps, non-IT departments, productivity apps that Gen AI could disrupt, other AI spend like legacy machine learning, analytics and not surprisingly, legacy RPA.
Large Companies More Likely to Shift Allocations
Remember this data represents percent of customers not budget amounts. But evaluating larger customers in the Global 2000 as a proxy for big spenders, the figure increases from 42% to 50% of customers stealing from other budgets. Moreover, the percent of customers allocating new funding in this cohort drops to 40% versus 49% in the broader survey.
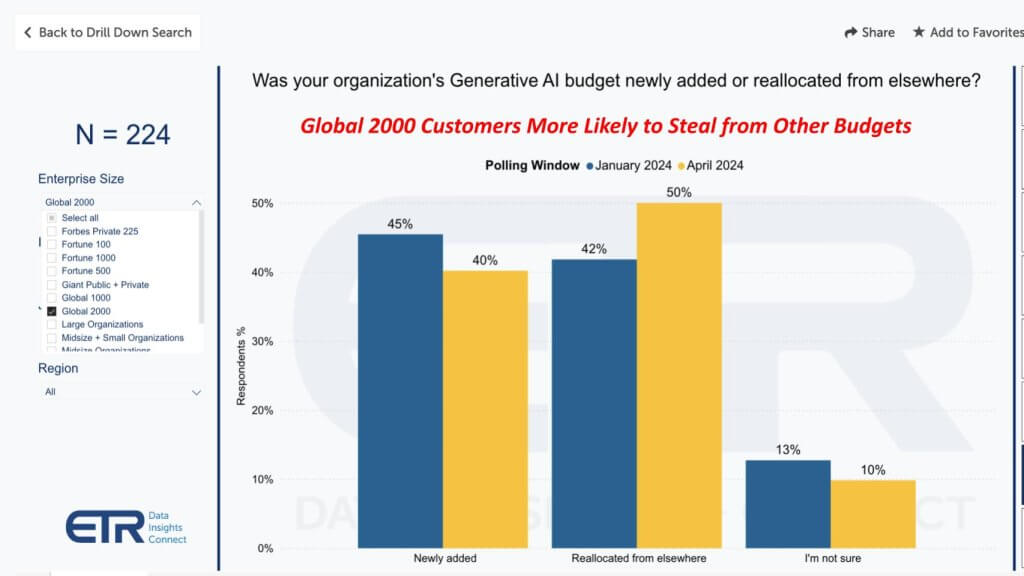
This data above represents results from 224 Global 2000 respondents so at more than 10% of the G2000 it’s a pretty representative set of respondents.
The point is… because of the macro climate and perhaps other factors we’ll discuss below, CFOs are not simply opening their checkbooks. Now anecdotally select CFOs tell us they are being very aggressive about AI spend – but we see that sentiment is much more narrowly applied. We’ll be interested to look back 5-7 years from now to see how those aggressive companies are faring.
ITDMs Becoming More Conservative on Gen AI ROI Timelines
ROI expectations are becoming somewhat less aggressive. People naturally listen to the hype and think this AI stuff is easy – which in many cases it is – but it’s not as easy to drive measurable results to the point where you can throw off enough cash to fund future investments – not yet anyway. So ROI expectations are shifting to the right as shown on this chart below.
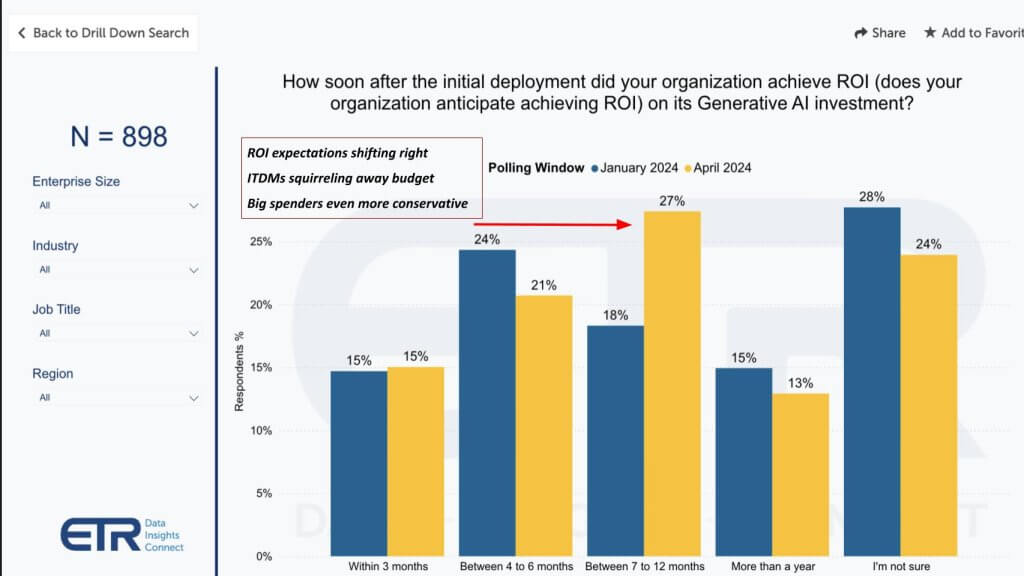
Meaning tech leaders are becoming less aggressive and signing up for a somewhat longer payback period. The norm is still inside of twelve months for mainstream projects but as you see there’s also still uncertainty on ROI timelines with 24% of customers citing they’re unsure. Those longer term ROI timelines we discussed earlier will be in the more than one year category representing 13% of customers.
The other thing we hear in conversations with customers is ITDMs squirreling away some of this budget as they try to determine where to place their bets. But as the call out notes the big spenders at larger companies are even more conservative as shown below.
Larger Customers Somewhat More Conservative
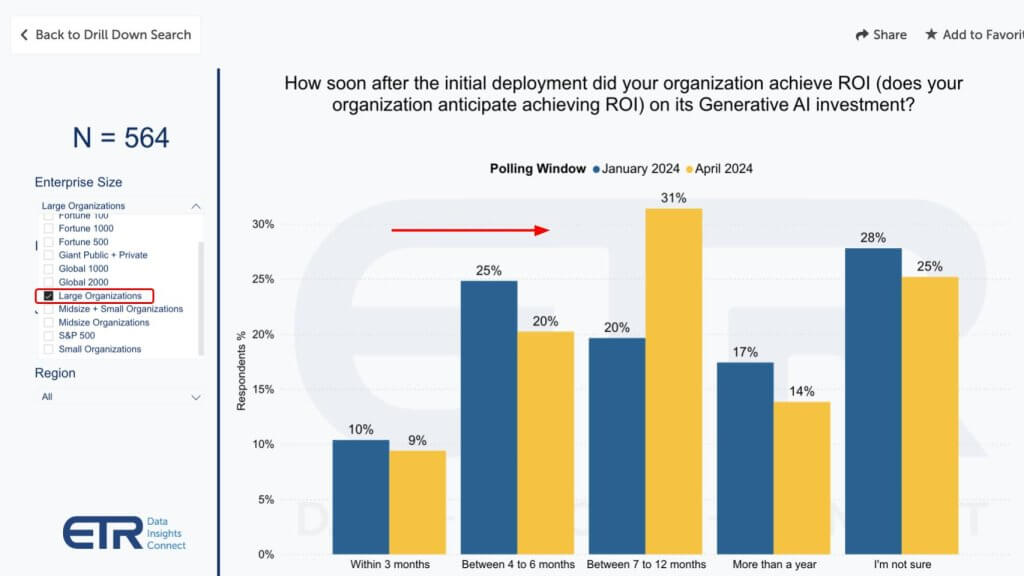
And why not? Why sign up for a short ROI when you’re still experimenting with Gen AI and basically applying ChatGPT-like use cases to your business.
How Enterprises are Deploying Gen AI for Business Use Cases
Below we show how Gen AI is being used in 1,844 customer accounts. The use cases are not remarkable by any means. It’s what you’d expect with basically ChatGPT-like examples. Specifically:
- Text summarization is the most popular.
- Followed by customer chat support
- Then code generation and writing marketing copy.
- Some image editing and design which is becoming much more functional
- And a low single digits of “other” which likely includes some of those more complex use cases like the ones we mentioned up front – advertising to drive revenue and things like drug discovery.
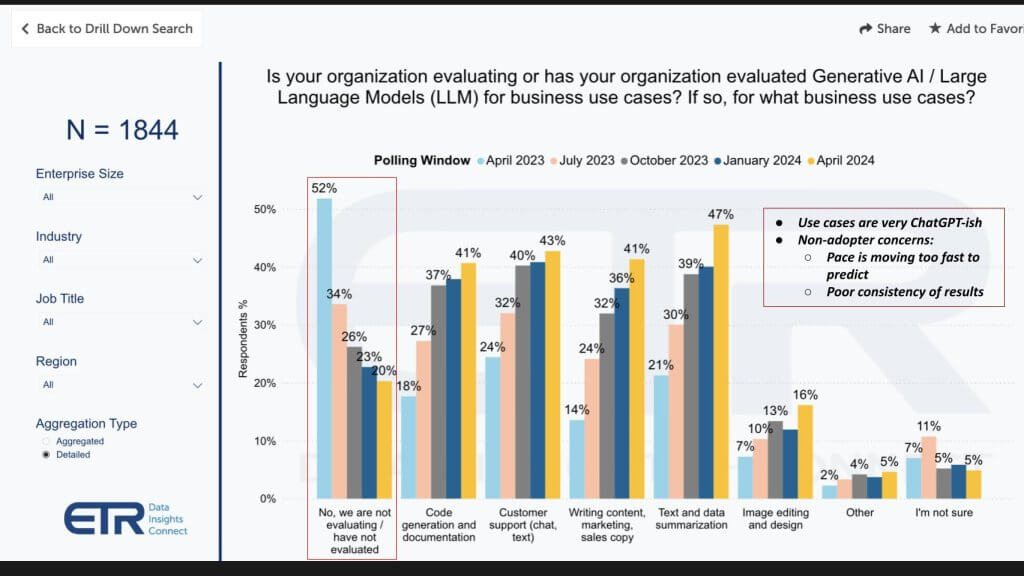
Those other cases are expensive. They are training intensive and require access to GPUs, which either you’re going to get from cloud providers, or alternative GPU clouds like Coreweave, Genesis or Lambda, etc. Or you’re going to buy GPUs and do the work on prem with GPU-powered servers from Dell, HPE, Lenovo, Supermicro or other ODMs. But for folks wanting to do serious training directly buying GPUs from NVIDIA, if you’re not willing to commit spending $10M, there’s a good chance you’re going to wait in line for over a year to get some.
Eighteen Percent of Respondents are not Pursuing Gen AI Initiatives
The other callout on this data is highlighted on the left in red. It shows the percent of customers who say they’re not evaluating Gen AI and LLMs. Surprised? So were we. And while the number has come down rapidly, in looking into this we’ve confirmed it’s true. It’s not necessarily that there’s no Gen AI happening at the company but there are a number of folks we’ve talked to that say it’s too complicated and moving too fast right now to pick winners so they’re waiting for the storm to subside and they’ll learn from others’ mistakes. The other thing we’ve heard is shown in the insert that folks just don’t trust the models right now because the results are unpredictable and it’s too risky.
To both of these we’d say at the very least you need to start thinking about your AI platform organization and architecture. AI is going to force changes to how you serve customers. The way you support your general purpose workloads of CRM, ERP and collaboration software today won’t necessarily directly translate to AI. We advise thinking about your AI platform and how to construct that. You’re likely a hybrid shop today so how do you evolve that into hybrid AI with a combination of cloud and on prem? And how are you re-thinking your data strategy to support AI by unifying metadata, rationalizing disparate data types with semantics and so forth.
Customers Have a Dizzying Set of AI Options
We agree, innovation is moving fast and there are many choices. It’s hard to keep up. Below is some data from ETR on AI tools adoption by vendor and platform.
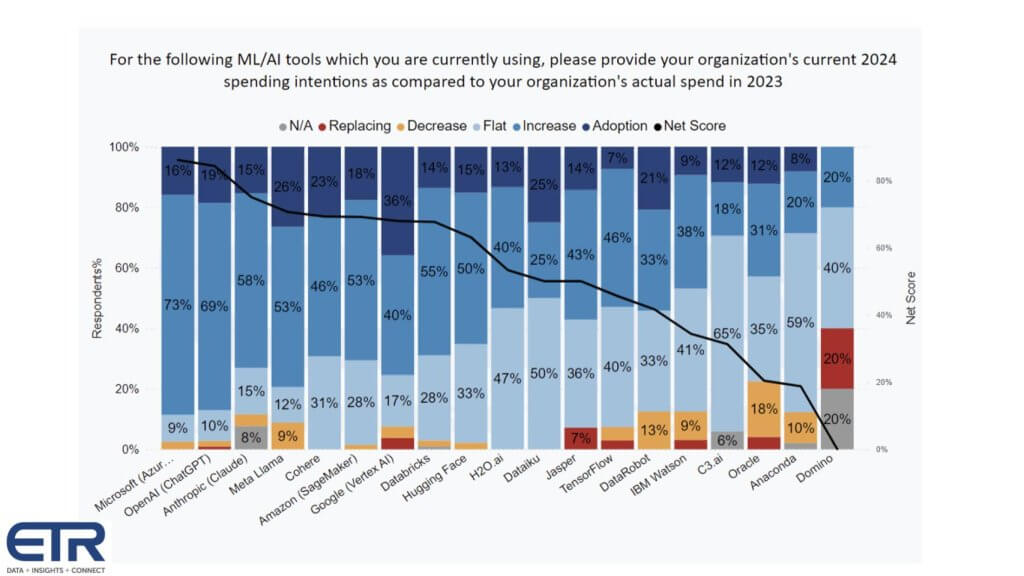
It shows for each AI vendor, the percent of customers in the latest survey: 1) Adopting the platform new – that’s the dark blue; 2) Investing more in the light blue; 3) Flat spend in the powder blue; 4) Decreasing spend in the orange; 5) And churning in the red. Subtract the orange and the red from the dark and lighter blues and you get Net Score which is that dark blue line. Note that anything above a 40% Net Score is considered exceedingly high.
What this data is telling us is:
- OpenAI and Microsoft are like iPhone and AT&T in the early days with a partnership that is getting a lot of attention and has momentum. With a Net Score of 71%, Microsoft’s AI customer momentum is among the highest in the survey. Meanwhile from the January 2022 to the April 2024 surveys, respondents citing they’re using Microsoft’s AI have grown by 3.5X (175–>611).
- Google is closing the gap on AWS. While both firms’ AI initiatives have continued strong momentum, the ETR survey data shows that in January 2022, AWS had 62% more customers using their AI and that gap has narrowed to under 10% in the April 2024 survey.
- In that same timeframe, Google’s N’s in survey have grown by 3.7X (93–>340) and AWS’ have grown by 2.5X (151–>370).
- Anthropic has made a big move up recently to surpass Llama in spending velocity.
- Cohere has strong momentum.
- Amazon SageMaker is still popular for a lot of AI applications where Gen AI is not well suited.
- Databricks legacy ML is doing well – DBRX is not in these numbers.
- Hugging Face is also popular.
- The pre-ChatGPT firms like H20 and others are evolving their portfolios to compete in the new market context.
IBM is interesting to us because for the first time in a long time we’re really excited about IBM’s AI business. The data is not as friendly here and we suspect it’s because IBM is still cycling through old Watson and ramping watsonx with corresponding services around data, governance and the like. But IBM is definitely in the game as is Oracle.
A Fast Moving Market Makes it Difficult for Customers
We sympathize with the complexity of the situation right now. As an example, Databricks announces DBRX touting benchmarks of an MoE model that beats Mixtral then Mistral counters with a new high water mark. And Amazon ups its investments in Anthropic but they’re building Olympus, an internal foundation model which they want to make better than Anthropic’s Claude. Then Microsoft does an aqu-hire of Inflection…so yeah it’s complicated.
But the way to prepare is to think about the architecture that serves your business and build your own system that fits your needs. That may mean focusing primarily on processes and people versus technology which is fine but there will be an underlying technology architecture that at some point you’ll have to leverage to compete so you want to be thinking about that now. And bringing in the expertise and partnerships to help you build it for your purposes.
Where to do AI – Cloud or On-Prem – It Depends…
Ok the last thing we’re going to talk about is cost of cloud versus on prem. And indirectly inferencing versus training. Everyone is debating this. The cloud platforms are moving fast and let’s face it, that’s where most of the action is with Microsoft pulling off the OpenAI coup, forcing both Google and AWS to respond with their internal “code reds.”
The GPU clouds we talked about earlier are popping up and raising lots of venture money while Meta is throwing its open source weight around with Llama. The hyperscalers are winning the CAPEX wars because they are swimming in cash and so they’re buying as many GPUs as they can. At the same time they’re building their own silicon.
But then at at GTC, Michael Dell getting a call out from Jensen that nobody is better at building end to end systems than Dell. Because Dell has by far the most comprehensive end to end portfolio of anyone from laptops to high end servers. But how do you think HPE, Lenovo and Supermicro feel about that? So HPE just announced that Jensen will be speaking at the Sphere with Antonio Neri at HPE Discover.
And so it goes…
The reason we bring this up is all this jockeying for position is adding to the confusion. The cloud players are saying come to us we have optionality, tools, innovation and scale. The alternative GPU clouds are saying that they have purpose-built services for the AI era that is more cost effective and a better strategic fit for AI. And the on-prem players say you’re going to spend a lot of money in the cloud, come to us.
So we’ve got a hold of some data that we found eye catching.
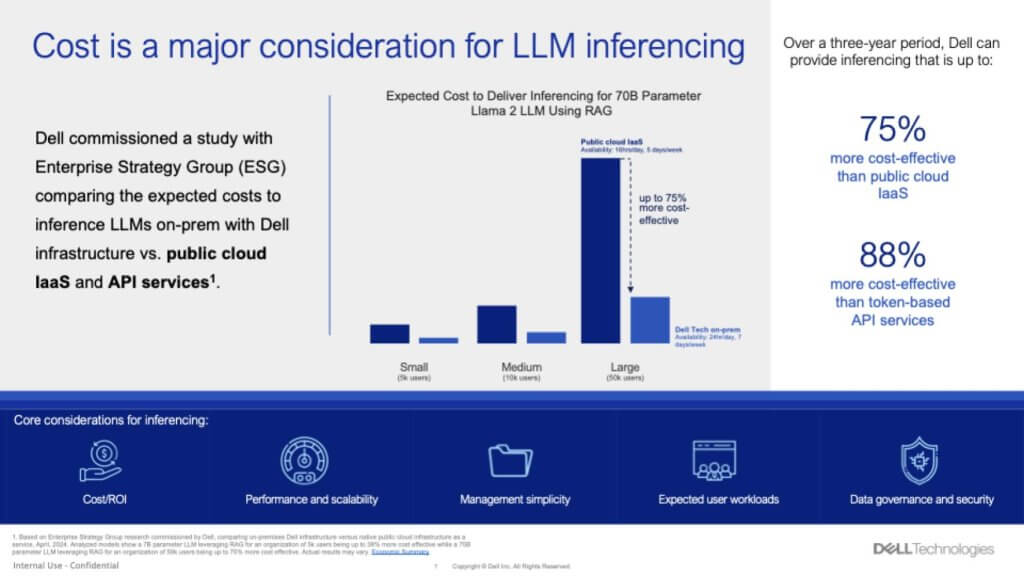
It’s data from a study commissioned by Dell and conducted by ESG. You have to be warned this was a paid for study and you know how these things are used in marketing. But the person who led the study, Aviv Kaufmann, is well respected and from what we’re told – not by Dell but others who know him – he would not compromise his ethics and has a strong engineering background.
Cutting to the chase – just to add more FUD into the conversation – this data shows that doing inference on prem for a 70B parameter Llama 2 LLM using RAG is far cheaper on-prem than in the cloud. And the two points in the call out are: 1) If you’re driving your RAG via token-based API services from the likes of OpenAI, you’re going to pay a price over doing your own RAG on-prem with open source models; and 2) RAG is not that hard to do. And doing RAG-based workloads with Dell powered GPU servers is more cost effective than using IaaS on AWS or other clouds.
Ping Pong Matches
Now we love the back and forth whether it’s benchmarketing or TCO wars. So the first thing AWS is going to point to is they would run this model differently using their custom chips. That is AWS would say “if you want lower cost inference you should use our custom inferentia chips.” Of course you can only get them in the AWS cloud. Dell doesn’t make its own chips. And the back and forth continues.
The point is this is one of those “it depends” moments. Cloud company A will tell you it’s much less expensive to do IT in the cloud than on prem with all that heavy lifting and the on-prem guys will say “that may have been true in 2010 but we’ve replicated the cloud operating model on-prem” and the debate goes on. It may very well be more expensive sometimes to do work in the cloud but oftentimes the developers in an organization are so in love with their cloud it’s worth it. Or the access to new services or innovations in the cloud are often better.
At the same time, cloud bills are sometimes very expensive and unpredictable. Moreover, the data you want to use for your AI may not be in the cloud so in all likelihood, while the cloud is and will likely continue to grow faster than on-prem, most customers are living in a hybrid IT environment and that will extend to hybrid AI.
But we’d like to speak with Aviv and learn more about this study so we’ll reach out to him and hope he’ll talk.
Things to Watch in AI Adoption
Let’s leave with a few thoughts on some of the things we’re paying attention to around enterprise AI adoption.
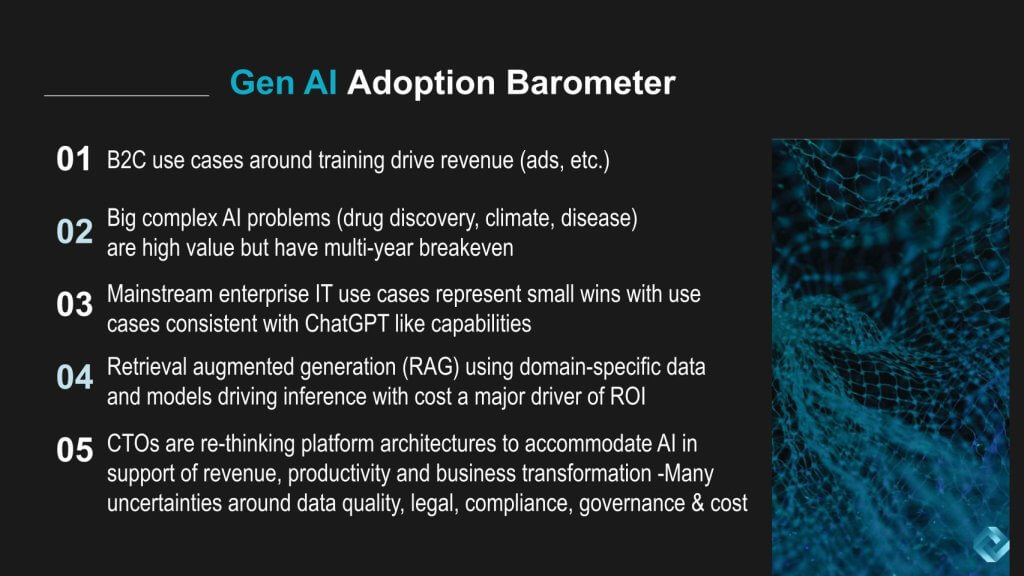
Despite all the confusion one thing is clear – the big money in AI right now is in superchips, big memories, fast interconnects and training. B2C use cases are no-brainers for AI because the bigger the AI cluster you can build the better AI and ad targeting you’ll have.
But those big complex problems in healthcare, climate, and the like have long investment horizons and will take time to pay back. They may not see ROI for a decade. They are training intensive so the demand for big GPUs is here to stay in our view. NVIDIA has a monopoly in super GPUs and will hold its serve for the better part of a decade in our opinion.
Mainstream enterprise use cases are focused on productivity and quick hits that are very ChatGPT-ish in nature. Document summarization, ideation, code generation, etc. These are nice but in and of themselves will not transform industries.
RAG is not that hard to do. And it allows domain-specific inferencing. There are lots of experiments going on with RAG today but the big money use cases are not easy to find. They’re fun. They’re cool. But cost is going to be a factor here. Low cost inferencing at the edge is going to be the dominant AI use case in our view. It won’t necessarily create monopolies but there will be a lot of it. And as we’ve said for years, it will be based on the Arm standard.
And finally, forward thinking CTOs and this emerging role of an AI architect are envisioning new platform strategies. Waiting for the storm to clear may not be the best approach with respect to architecture. There are some knowns like the type of workload patterns AI requires and how training and inference work may have unique requirements. And much of this is about governance, data quality and other corporate edicts. These decision points should be ongoing and iterative with platform, not product thinking.
Cost will come into play. It always does. Remember the math on ROI is really simple. Benefit divided by cost. So what happens when you drive the denominator toward zero? The result goes to infinity. But the size of the benefit matters too. So a 1000% ROI on a project with a $100 NPV isn’t nearly as interesting as a 12% IRR on a billion dollar net present value. But if it takes 10 years to get a payback…a lot can change in 10 years.
Confused about what do to with AI? You’re not alone, so think about the people and process effects and the dramatic change in business process that AI can bring. Then get going on architecture, platforms, iterative development and learnings from experiments. But don’t stick your head in the sand and hope to figure it out down the road or your company may be out of business.
What do you think? Does this data we shared reflect what’s happening at your organization? Do you have it all figured out or are you struggling to keep up? Where are you placing your AI bets and how are you funding them?
Let us know.
Image: zobaair