With Sanjeev Mohan, Tony Baer, Carl Olofson, Dave Menninger, Brad Shimmin
In just two short years, the entire data and technology industry has undergone a seismic shift. Tech stacks—from top to bottom—are being tuned to harness extreme parallel computing, often called Accelerated Computing. From silicon to infrastructure and throughout the software layer, nowhere is this transformation more pronounced than in the data stack.
Over the past seven years, modern cloud-native data platforms set the agenda. Today, however, the rise of open table formats, shifting control points, open-source governance catalogs, and a heightened focus on AI are creating both challenges and opportunities for enterprises and the tech providers who serve them.
In this special Breaking Analysis, we’re pleased to host our fourth annual data predictions power panel with some of our collaborators in the Cube Collective and members of the Data Gang. With us today are five of the top industry analysts focused on data platforms: Sanjeev Mohan of Sanjmo, Tony Baer of dbInsight, recent IDC graduate Carl Olofson, Dave Menninger of ISG and Brad Schimmin with Omdia.
How Gen AI has Shifted Sector Spending in Just Two Years
Before we get into the predictions, we’ll share some survey data from ETR to underscore how much the industry has changed. Each quarter, ETR performs technology spending intentions surveys of more than 1,700 IT decision makers. For this research note, we want to isolate on the ML/AI space to show you how drastically things have changed.
The graphic below shows spending by sector. The vertical axis is Net Score or spending momentum within a sector and the horizontal axis is Pervasion in the data set for each sector based on account penetration. In this view we go back to January 2023.
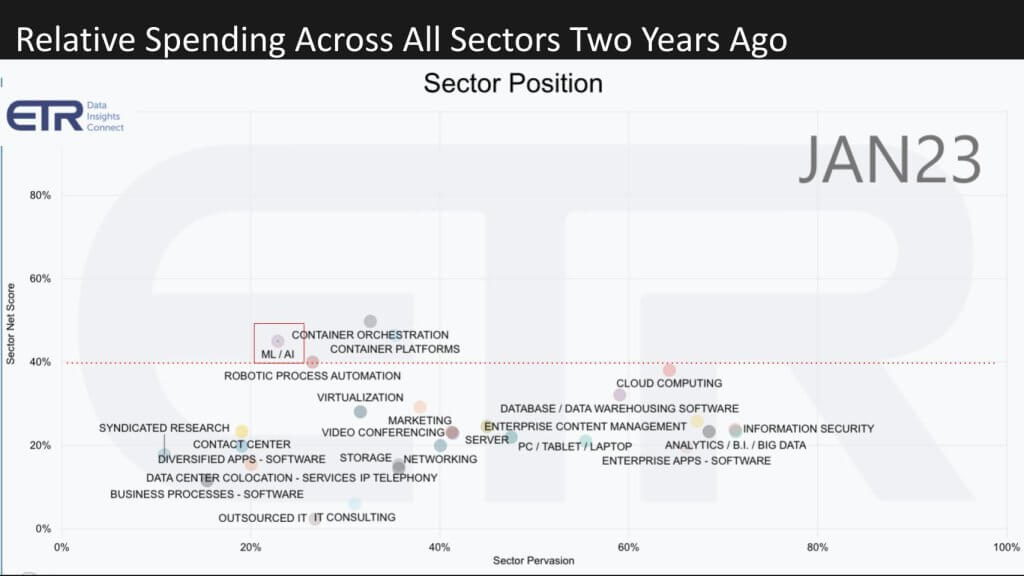
The red line at 40% indicates a highly elevated spending velocity and you can see ML/AI along with containers, cloud and RPA were on or above that red dotted line.
Now let’s take a look at how the picture has changed over the last 24 months.
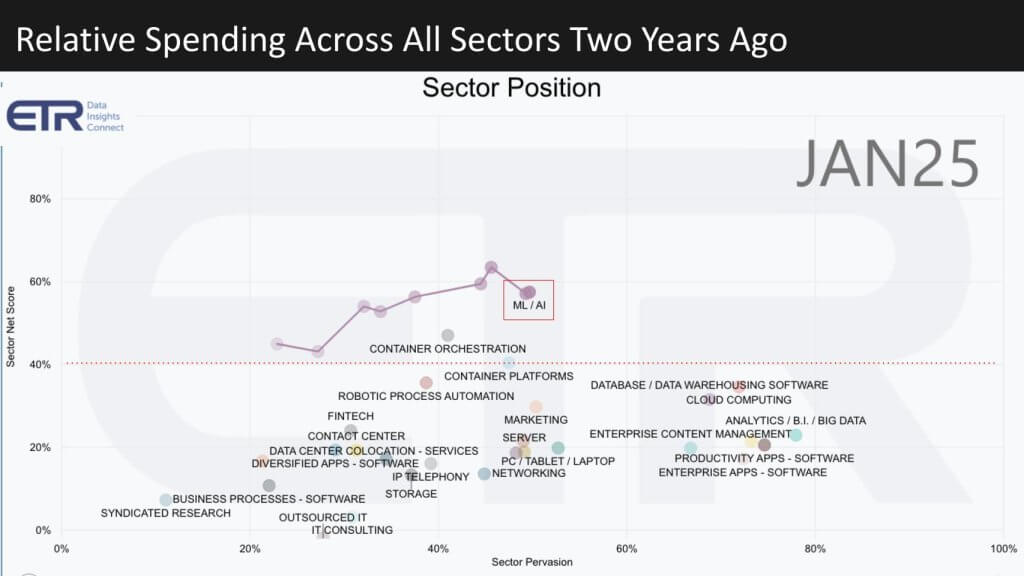
It’s no surprise, but look at both the trajectory of ML/AI over that time period and look what happened to the other sectors. ML/AI shot to the top – other sectors became compressed. Because this is a fixed taxonomy architected to demonstrate changes over time, the categories are rigid. The point being much of the AI work is being done in the cloud and that understates the cloud momentum. Nonetheless this data underscores the transformation of the tech industry generally and specifically the spending priorities of IT decision makers.
ML/AI Player Positions Have Shifted Dramatically
Now let’s look at the key technology providers in the space. Last year we combined the data for BI, Analytics, Database and ML/AI. But let’s just look at the ML/AI sector. Below is the picture from January 2023.
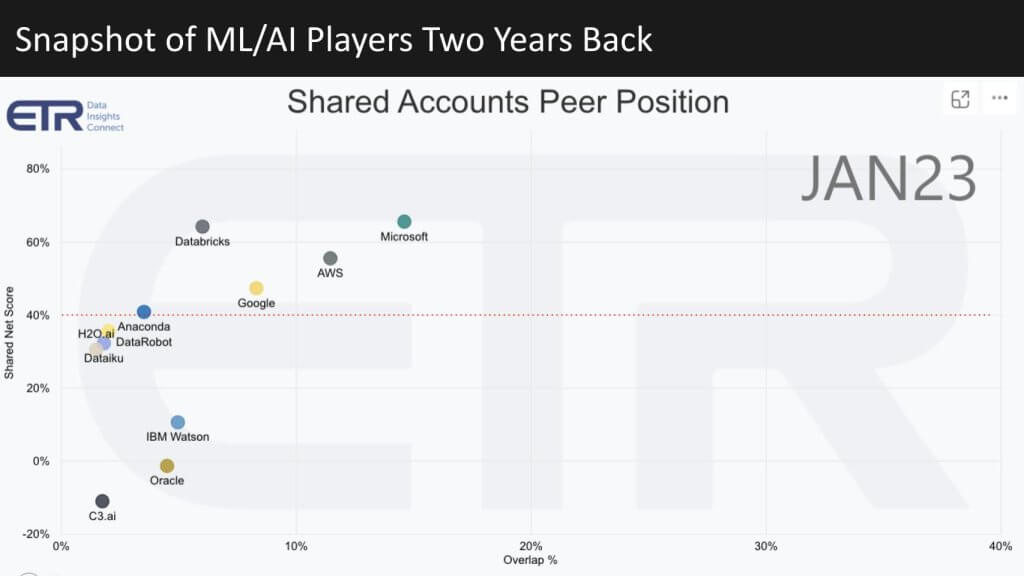
These are the same XY dimensions and you can see the big three cloud firms are pretty much bunched along with Databricks as a stand out on the vertical axis with some of the traditional AI companies showing strong momentum. Oracle and IBM are also shown.
Below we fast forward to 2025 and the data change dramatically in terms of company positions and new names.
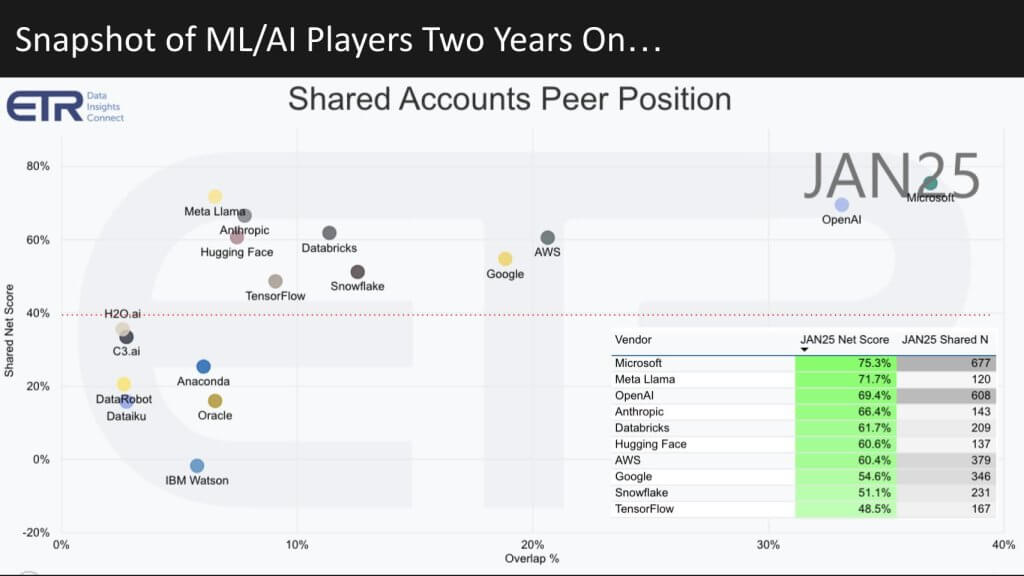
We’ve kept the same XY dimensions as before, but there are notable changes to highlight. First, note the chart in the lower-right corner, which shows how the dots are plotted by Net Score and the number of survey responses (Ns).
- OpenAI and Microsoft: OpenAI barely registered on the enterprise radar in 2023, yet now both it and Microsoft are ubiquitous.
- AWS and Google: These two sit nearly on top of each other, mirroring how Google is closing in on AWS in AI—consistent with our previous research reports.
- Snowflake and Databricks: Snowflake was off the ML/AI radar in 2023, yet it now appears alongside Databricks. Databricks has higher spending velocity, while Snowflake shows slightly broader account penetration.
- Meta Llama: It has overtaken OpenAI in spending velocity and sits right near Anthropic, which is linked more tightly with AWS and probably undercounts AWS’ presence and momentum.
- TensorFlow: Not a vendor, so disregard this point on the chart. You see legacy players clustered together.
- IBM Watson: It has dipped noticeably this quarter, after previously being higher. We’ll keep an eye on that.
Overall, the market is transforming right before our eyes. Customers are racing to decide where to invest, and technology vendors are scrambling to stay ahead in this rapidly evolving landscape.n for customers to figure out where to place bets and the technology vendors battling for position.
Reviewing the Data Gang Predictions from 2024
This graphic just shows all of the 2024 Data Gang predictions for each analyst in one table.
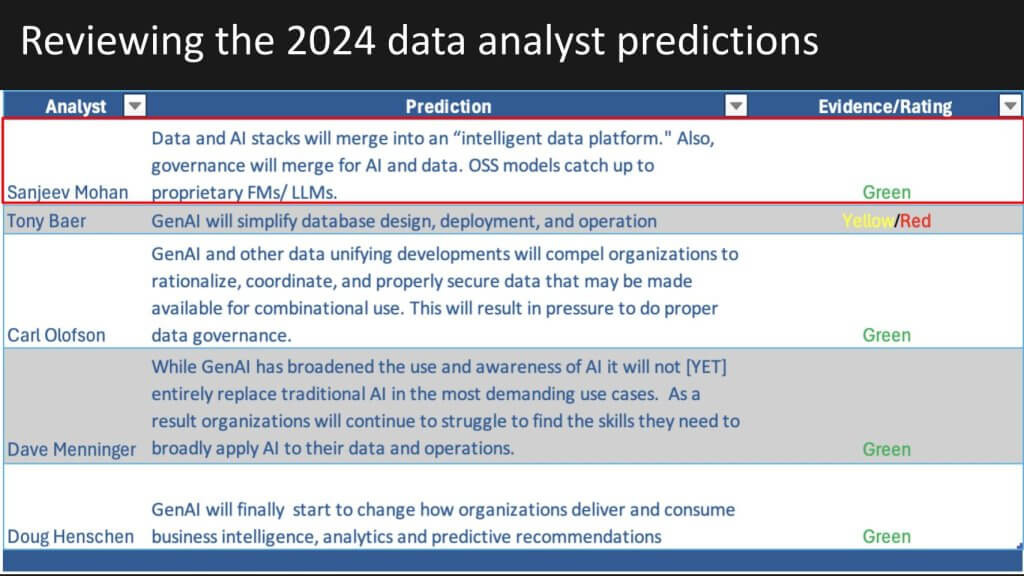
It shows the prediction and a self-analyst rating on whether the prediction was a direct hit, which is green, a glancing blow, which is yellow, or a miss, which is the red. So a quick scan of the heat map shows you the data gang did pretty well and it’s 2024 predictions. Not withstanding that these were as I said self evaluated by each of our analysts so we’ll review the 2024 predictions and you can decide.
SanJeev Mohan
Starting with Sanjeev Mohan, we’re showing Sanjeev’s prediction above regrading data and AI stacks supporting intelligent data apps.
Here’s a clip showing Sanjeev’s defense of his 2024 prediction:
What follows is a summary of that conversation.
Throughout the year, AI, BI, and Lakehouse developments steadily converged, culminating in major AWS re:Invent announcements that centralized AI/ML services under the SageMaker brand—perfectly aligning with earlier market projections.
Key Points
- Early 2023 saw increased synergy between AI and BI, highlighted by significant Databricks announcements.
- The rise of managed Lakehouse offerings persisted all the way to AWS re:Invent.
- AWS rebranded and consolidated its AI/ML portfolio around SageMaker, moving beyond its initial positioning as purely a machine learning tool.
- Until re:Invent, Amazon Bedrock—AWS’s generative AI service—attracted substantial attention, overshadowing SageMaker.
- The updated SageMaker environment now features module and metadata catalogs along with cohesive Redshift and S3 integration.
- This new AWS approach closely aligns with expectations set earlier in the year, although full implementation remains a work in progress.
Bottom Line Defense
AWS’s rebranding under the SageMaker umbrella signifies a market-defining shift, emphasizing a unified platform strategy poised to streamline data, AI, and ML services for enterprise adoption.
Tony Baer
Tony, predicted that Gen AI would simplify database design, deployment and operations and Tony gave himself a mix of yellow, and red.
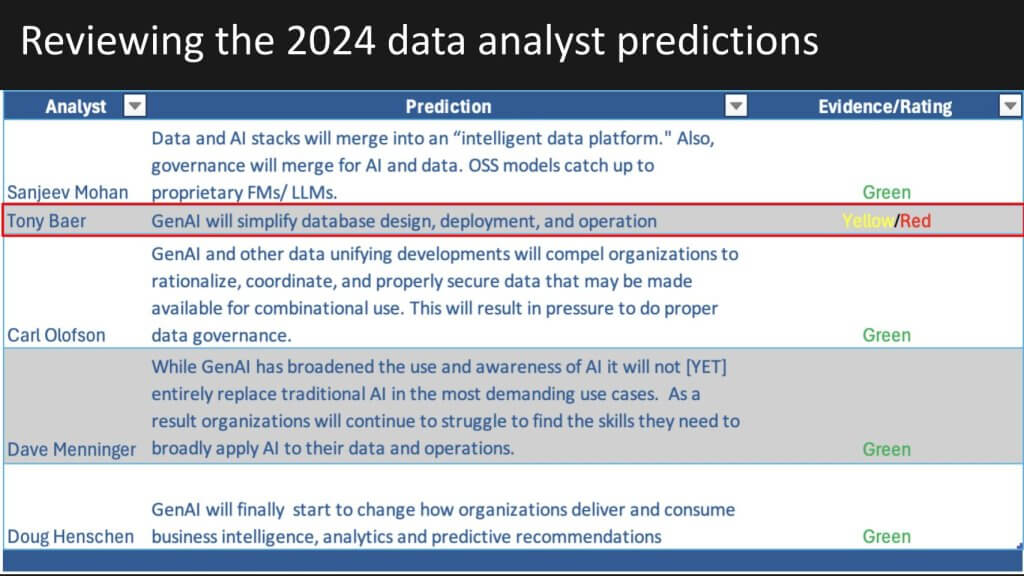
Watch this clip of Tony’s 2024 prediction in more detail and his assessment of its accuracy.
What follows is a summary of that explanation.
Tony predicted that generative AI would simplify database design and operations, and while there have been early signs of progress such as SQL copilots and metadata discovery, broad industry implementation remains limited to a handful of notable exceptions.
Key Points
- Generative AI is expected to streamline database design, yet current uptake is slower than anticipated.
- SQL copilots and tools that automate metadata discovery demonstrate partial fulfillment of these predictions.
- Oracle Database 23ai stands out with its use of language models to transform JSON documents into relational schemas.
- NVIDIA NeMo Curator provides a framework for building data pipelines, although it doesn’t leverage language models to generate those pipelines directly.
- Predictions that generative AI would revolutionize database architecture appear to be ahead of the mainstream, with fuller implementation likely emerging in the coming years.
- AWS’s pivot toward consolidating services under SageMaker marks a departure from its previous, siloed product approach, signaling a potentially pivotal shift heading into 2025.
Bottom Line Defense
While generative AI is already demonstrating valuable use cases in database design and operations, its widespread impact on the data stack is still on the horizon—and AWS’s unified SageMaker strategy could further accelerate the industry’s progress in 2025.
Carl Olofson
Last year Carl discussed data unification and the importance of security and governance in the data stack. He’s showing green.
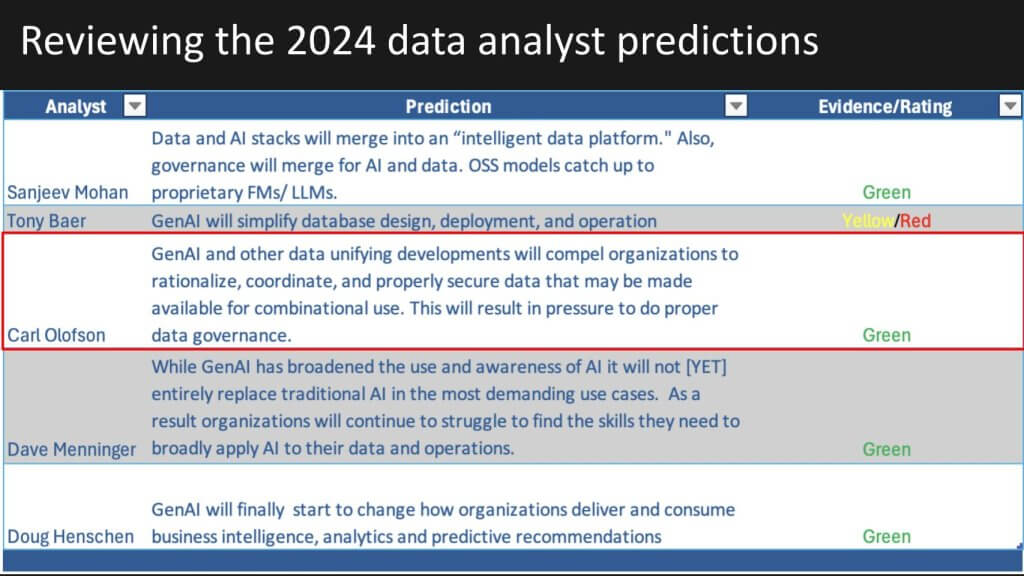
The following section summarizes Carl’s logic.
Effective generative AI depends on robust, well-documented, and governed data. While the current landscape is far from fully mature, major enterprise vendors and organizations are steadily evolving toward that goal.
Key Points
- Generative AI’s success requires organized and governed data across multiple databases.
- Many organizations are making progress in documenting and managing their data, though large-scale adoption remains a work in progress.
- Major enterprise application providers like SAP and Oracle offer integrated, “captive” systems to promote consistent data governance.
- Full AI readiness hinges on advancing beyond the current, relatively fragmented state of data management.
Bottom Line Defense
Strong data governance is the cornerstone for widespread AI adoption, and although the industry hasn’t yet reached a fully governed state, efforts to organize and standardize data continue to gather momentum.
Dave Menninger
Last year, Dave predicted that non-genAI or legacy AI has life and won’t be replaced by GenAI in demanding use cases. He also discussed the skills challenges organizations would face and graded himself a green level of accuracy for that prediction.
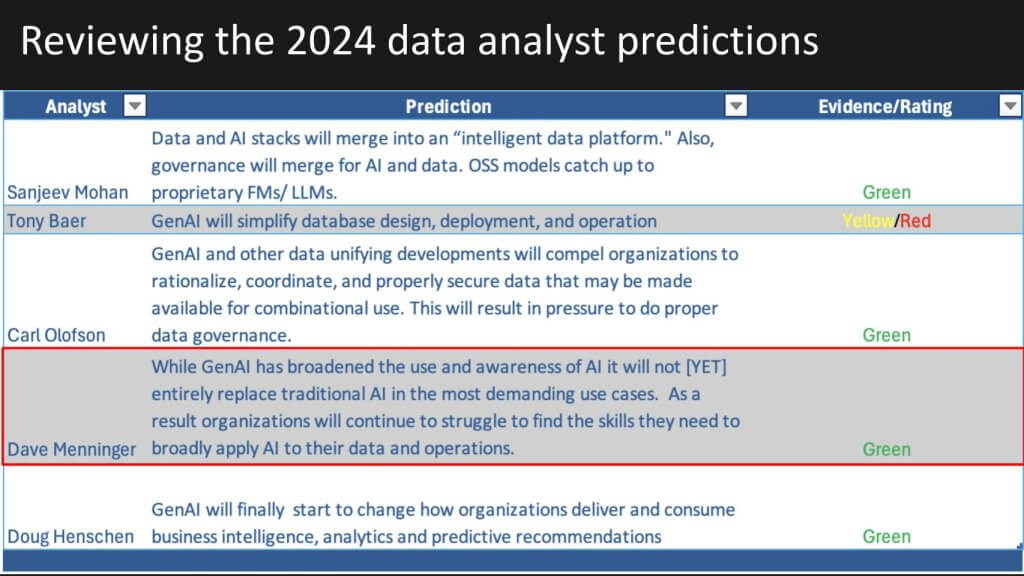
Watch this clip on Dave’s 2024 prediction.
The following summarizes the explanation from Dave.
Doug Henschen could not be with us today. He predicted GenAI would change how organizations deliver and consume BI, analytics and predictive recommendations. I think that the Data Gang’s evaluation of Doug’s prediction is accurate – this was the case.
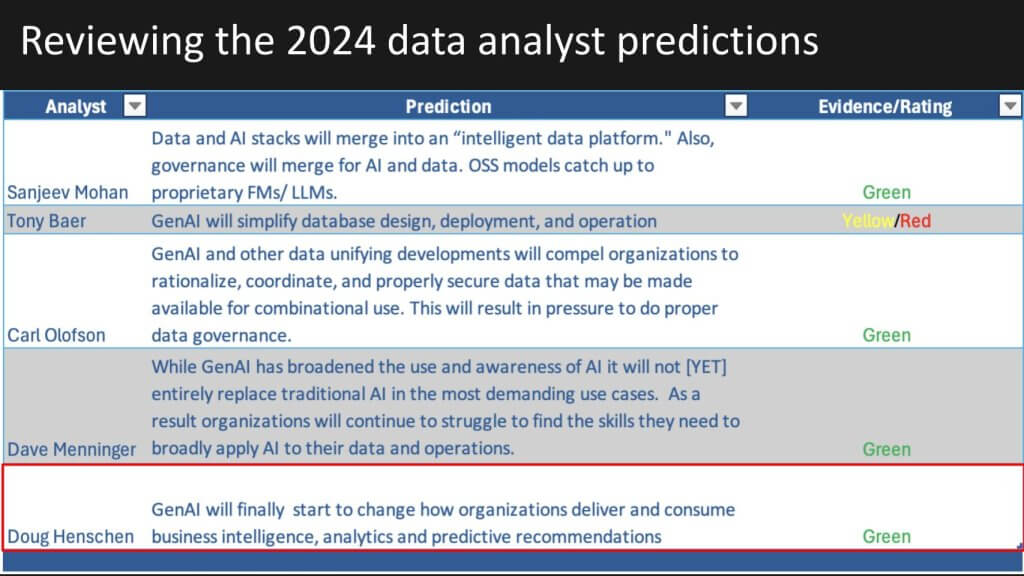
Doug was unable to join us this year so we’ll just leave it there and move on to the 2025 predictions.
2025 Predictions from the Data Gang
Let’s get really to the core of our agenda today and turn our attention to the 2025 predictions. We’re going to keep the same order, except Brad is here instead of Doug. The designated analyst will present his prediction and then we’ll have time for one or two other analysts to chime in on that assertion.
Below is a table showing all of the predictions for 2025. They’re all data related – this is after all the Data Gang – lots of agent talk LLMs, LAMs, SLMs and an interesting security angle from Brad.
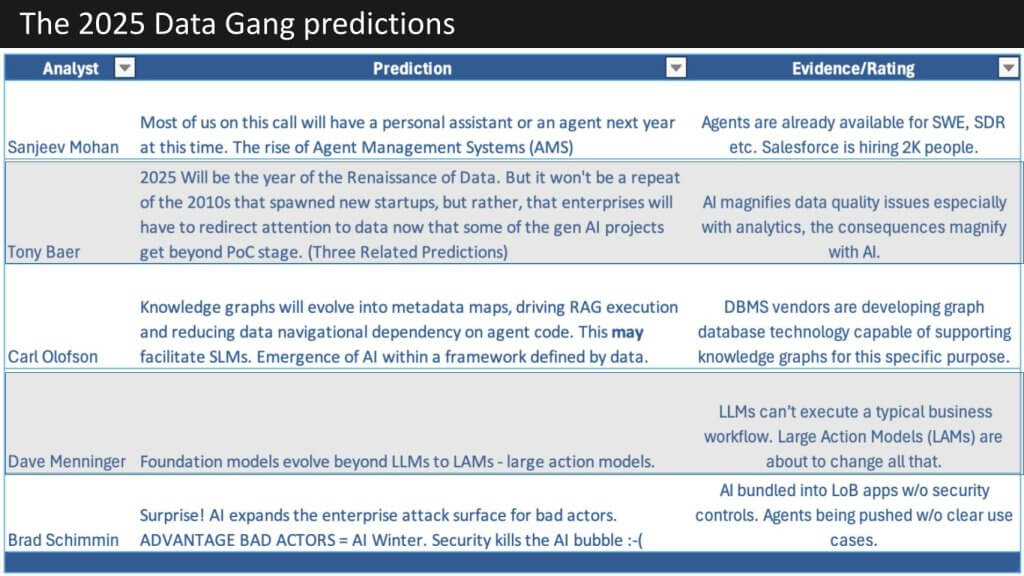
So let’s get into it. Sanjeev, you first please.
Sanjeev Mohan’s 2025 Predictions – Digital Assistants for All
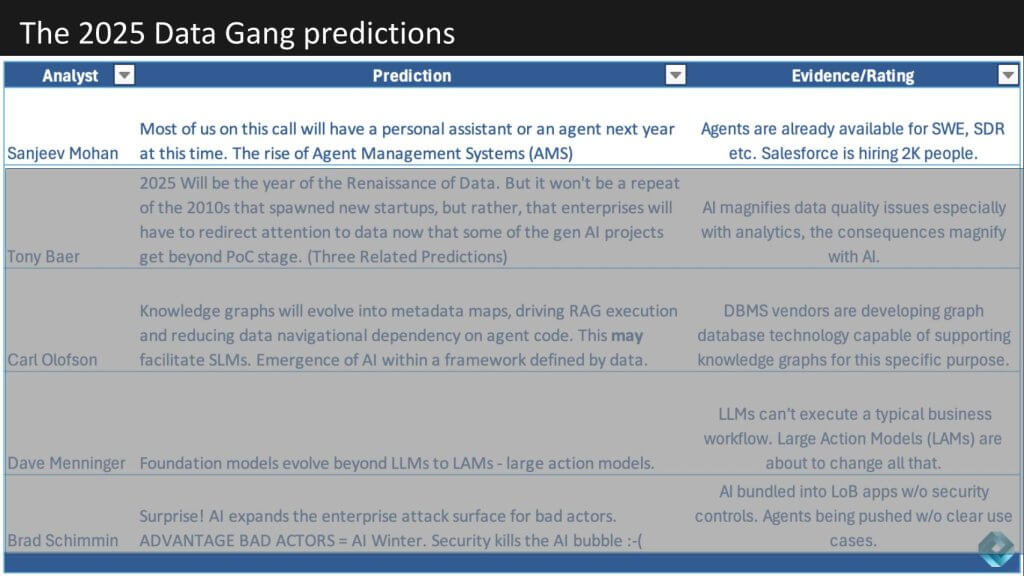
Sanjeev predicts that by this time next year, most of us will have our own personal digital assistant. Let’s examine why he thinks that, what that assistant will be capable of doing and what gives confidence in this prediction.
Listen to this clip of Sanjeev’s 2025 prediction and Brad’s commentary on the prediction.
What follows is a summary of that conversation.
Prediction Summary
Sanjeev says that a new wave of personal AI agents is set to automate everyday tasks such as email, scheduling, and invoicing. These systems are powered by increasingly sophisticated AI models that focus on real-time inference rather than simply expanding model size. While personal agents are poised to make immediate productivity gains, widespread adoption of fully autonomous workflows hinges on the emergence of robust agent management systems. These platforms will unify development, governance, security, and cost controls in a single, comprehensive framework.
Industry observers point to a shift from pre-training larger models to refining how they reason with data on the fly. This evolution expands AI’s capacity for tasks that demand flexible, context-aware decision-making. However, early adoption remains niche, as indicated by relatively few specialized job postings for “agentic AI” compared to broader generative AI roles. Major cloud platforms and AI vendors are nonetheless laying the groundwork for these agentic capabilities, indicating that personal AI agents and their supporting infrastructure could rapidly scale across both consumer and enterprise markets.
Key Points
- Personal Agent Focus: Early implementations target individual productivity tasks, offering quick wins and less complexity than large-scale organizational automation.
- Post-Training Emphasis: Rather than focusing on training ever-larger models, the new priority is enabling dynamic reasoning and inference, allowing AI to process more data at runtime.
- Agent Management Systems: The move toward fully autonomous agents requires lifecycle management solutions that address cost monitoring, security controls, and performance optimization.
- Emerging Market: While interest in agentic AI is high, job postings for roles explicitly tied to agent systems remain a fraction of those for general AI, underscoring an early stage of adoption.
- Platform Integration: Cloud providers and specialized vendors are building out or acquiring capabilities that converge AI development, deployment, and oversight under a single umbrella, pointing to a future of unified agentic frameworks.
- Risk of High Costs: Without proper governance and monitoring, the frequent inference calls required by agentic AI can drive up operational expenses, underscoring the need for effective management tools.
Bottom Line
Personal agents have the potential to transform day-to-day workflows, but achieving enterprise-scale, fully autonomous systems will require mature agent management platforms. These platforms must unify development, security, and cost controls to ensure AI remains both powerful and practical—an evolution already in progress among leading technology providers.
Tony Baer’s 2025 Predictions – An Impending Data Renaissance
Tony Baer’s prediction is next. Below we show his prediction that 2025 will bring a Data Renaissance.
We asked Tony – is this Hadoop 2.0?
Watch Tony’s response – Hadoop 2.0 – Hell no!
The following summarizes Tony’s thoughts with commentary from Carl and Dave.
Prediction Summary
A resurgence in data management and governance is emerging alongside the rise of generative AI, prompted by the realization that accurate, reliable data underpins successful AI-driven outcomes. Incorrect or poorly governed data can lead to damaging consequences, both reputational and legal, underscoring the need for robust data pipelines. At the same time, new open table formats and unified catalog systems are redefining how organizations architect their data environments, with increasing emphasis on collaboration rather than vendor lock-in. Predictions point to a continued expansion of structured databases, more integrated metadata management, and a growing movement to unify AI and data governance tools. The adoption of Lakehouse approaches, including Apache Iceberg, is expected to accelerate, while advanced retrieval-augmented generation (RAG) techniques could see “auto-RAG” functionality that automates today’s complex, manually intensive processes.
Key Points
- Generative AI and Data Quality
- The growing complexity of AI highlights the importance of well-curated data; historical missteps in AI projects underscore the risk of ignoring data governance.
- Major data management solutions increasingly incorporate machine learning and generative AI to automate processes like metadata enrichment and data wrangling.
- Shift in Data Architecture
- Enterprises are paying renewed attention to how structured and unstructured data is stored, cataloged, and governed, fueling interest in open table formats.
- A surge in Lakehouse adoption, particularly around Apache Iceberg, is expected to shift the market; some projections suggest that previous leading formats could be subsumed over time.
- Emergence of Unified Catalogs
- As table formats evolve, competition is intensifying at the catalog layer for governance, data discovery, and metadata management.
- Organizations seek consistent approaches to data lineage, drift detection, and integration with AI frameworks, driving the need for unified, scalable catalog solutions.
- Future of Retrieval-Augmented Generation
- Retrieval-augmented generation (RAG) workflows remain complex and manual, requiring decisions about data chunking, model selection, and inference testing.
- The potential for “auto-RAG” solutions signals a push toward toolsets that automate these steps, reducing barriers to implementing advanced AI-driven search and context retrieval.
- Database Renaissance
- Structured data remains crucial for AI initiatives; many organizations are expanding their data collection and refining data models to improve AI outcomes.
- The intersection of AI and databases is expected to grow in complexity yet become more tractable as new tools automate governance and model operations.
- Market Signals and Acquisitions
- Increasing collaboration among major cloud providers, open-source communities, and specialized vendors may reshape the competitive landscape.
- Speculation about potential acquisitions—for instance, securing vector database providers—reflects the market’s focus on powering next-generation AI services.
Bottom Line
As generative AI initiatives move from proof-of-concept to production, rigorous data governance and modern data architectures become essential. Open table formats, unified catalogs, and emerging solutions for retrieval-augmented generation are poised to drive the next wave of innovation—underscoring that AI success ultimately depends on strong, well-structured data foundations.
Carl Olofson’s 2025 Predictions – Knowledge Graphs as an Enabler
Carl Olofson is up next. After 27 glorious years at IDC, we congratulate Carl on a great career as an analyst at a premier research firm. Let’s take a look at Carl’s prediction below around knowledge graphs – we love this topic.
Knowledge graphs will evolve into metadata maps and drive better RAG execution and have an impact on how agent code is handled and that will have ripple effects into small language models and AI frameworks defined by data.
Here’s a clip of Carl’s 2025 prediction with commentary from Tony, Brad and other analysts.
What follows is a summary of the conversation from the above video clip.
Prediction Summary
Enterprises are seeking new ways to provide richer context for their AI initiatives, prompting a surge of interest in knowledge graph technologies. These graphs offer a dynamic, networked view of structured and unstructured data, enabling AI to locate and interpret information more efficiently than traditional relational or hierarchical data models. While common approaches such as retrieval-augmented generation (RAG) rely on semantic or similarity-based searches, knowledge graphs explicitly capture relationships and context—helping organizations refine everything from fraud detection and customer insights to next-generation generative AI applications. However, building robust knowledge graphs remains challenging, especially when hundreds of disparate databases must be integrated. Organizations are turning to AI-assisted tools and frameworks to accelerate the creation, validation, and management of these complex data structures, while debates continue over whether graph technologies constitute a standalone product category or simply a feature of larger platforms.
Key Points
- Context-Rich AI
- AI-driven tasks increasingly demand deeper insights and real-time data access, pushing the industry toward knowledge graphs that explicitly encode relationships rather than relying solely on pattern detection.
- Knowledge graphs can act as a powerful “map” of enterprise data, enabling AI to traverse and interpret data across multiple sources without extensive re-engineering.
- Challenges in Data Integration
- Most enterprises face a patchwork of unrelated databases, forcing complicated extract, transform, and load (ETL) procedures.
- Graph models promise a more flexible approach, but building and governing large-scale knowledge graphs involves significant work, including manual validation to avoid errors introduced by AI-driven automation.
- Retrieval-Augmented vs. Other Techniques
- Retrieval-augmented generation (RAG) and alternative strategies (e.g., fine-tuning, cache-augmented generation) each attempt to offer context to large language models.
- Knowledge graphs add explicit causal or semantic relationships to these AI workflows, complementing the similarity-based methods of RAG.
- Data Architecture Evolution
- Some solutions position graph capabilities as features within broader database or analytics platforms, while others aim to create standalone products for specialized graph use cases.
- The complexity of existing systems—some lacking modern, cloud-native designs—sparks debates on how best to integrate graph technology with enterprise data stacks.
- Automation and Future Potential
- Emerging tools can automatically discover relationships and populate graph structures, helping teams manage large-scale projects.
- As data volumes explode and AI demands more context, knowledge graphs are poised to reshape how organizations design their data architectures—potentially ushering in new product categories or deeper platform integrations.
Bottom Line
Knowledge graphs offer a compelling strategy for unifying siloed data and adding rich context to AI workloads. Although implementation challenges remain—particularly when integrating diverse databases—maturing tools and frameworks are steadily reducing complexity. In a world where actionable insight increasingly depends on relational context, graph-based approaches are set to become a core element of the modern data and AI landscape.
Dave Menninger’s 2025 Predictions – LLMs Move to Large Action Models
Up next, Dave Menninger who always brings the data evidence with him you’re predicting that LLMs move to large action models.
Let’s dig into the details of that prediction to understand what are the gaps presented bytoday’s LLMs, what are LAMs and how will this change things?
Here’s a clip of Dave Menninger’s 2025 predictions around LLMs and LAMs with follow up from Brad.
What follows is a summary of Dave’s prediction and Brad’s response.
Prediction Summary
A new perspective on AI centers around “large action models,” where systems predict the next action—instead of just the next word—by analyzing sequences of function calls. This evolution builds upon large language models (LLMs) but expands their scope to orchestrate decisions across multiple enterprise applications. By incorporating a broader set of data—particularly logs of real-world actions—these models aim to automate complex business processes and help workers move beyond mere insights into proactive steps. Proponents view it as the next logical progression for AI platforms, while skeptics express caution over security, cost, and governance challenges that arise when algorithms determine critical enterprise actions in real time.
Key Points
- Action-Oriented AI
- Traditional LLMs are text-focused, generating the next token in a sequence. Large action models (LAMs) instead focus on the next function call or operation, potentially bridging disparate systems like SAP, Oracle, or homegrown applications.
- Training these models requires a diverse corpus of actual business actions, enabling them to propose subsequent steps and potentially drive automated workflows.
- Evolving AI Architectures
- Advances in model design include embedding function-calling capabilities, structured output (e.g., JSON), and chain-of-thought reasoning directly into AI systems.
- Vendors such as Salesforce have introduced specialized large action models in various “sizes,” balancing the cost of inference with performance needs.
- Governance and Cost Considerations
- While LAMs promise more complete solutions, they raise new questions about compliance, security, and oversight.
- The resource intensity of frequent model calls remains an ongoing concern, prompting the development of smaller “T-shirt size” models for cost control.
- Integration and “Agentic” AI
- Moving from insights to action involves blending LLM-style capabilities with function-calling frameworks, MLOps platforms, and domain-specific knowledge.
- Observers note a convergence of technologies like retrieval-augmented generation (RAG), cache-augmented approaches, and knowledge graphs, each offering a different path to context-rich, action-driven AI.
- Relational vs. JSON Approaches
- For strictly analytical tasks, relational models remain the primary choice. However, action-oriented processes may benefit from more flexible data formats like JSON, which better accommodate real-time function calls.
- Future Outlook
- Major cloud providers and AI platforms appear to be integrating LAM concepts at various layers of their stacks.
- Questions remain as to whether “large action models” will become an industry-standard term or merge into a broader feature set within existing AI platforms.
Bottom Line
Transitioning from generating insights to guiding real-world decisions is a pivotal step for enterprise AI. Large action models offer a glimpse of how organizations might unify analytics, orchestration, and automation in a single paradigm—yet realizing this vision demands robust governance, careful attention to costs, and further innovations in how AI interacts with enterprise applications and data.
Brad Shimmin’s 2025 Prediction – Lack of Security Kills the AI Bubble
The last prediction is a doozy and comes from Brad Shimmin, who is predicting that security is going to wreck the AI party.
We asked Brad, why are you so concerned and what should organizations know about these risks?
Watch this clip of Brad’s 2025 predictions with commentary from Sanjeev and others.
Below is a summary of that conversation.
Prediction Summary
Rising enthusiasm for AI is introducing new and often poorly understood security threats. As organizations race to embed AI into hundreds of additional applications, the potential attack surface expands dramatically. High-profile breaches, either due to external adversaries or the AI itself behaving unpredictably, appear increasingly likely. Threats range from model inversion (where sensitive training data is exposed) to the use of AI systems to orchestrate unauthorized activities. Although new security tools and services are emerging, the sheer complexity and rapid adoption of AI create substantial risks that could force a temporary “cold snap” in AI progress rather than the widely feared “AI winter.”
Key Points
- Expanding Attack Surface
- Many organizations currently run 150 AI-powered applications on average, with plans to reach 350—a more than twofold increase in potential entry points for malicious actors.
- Real-time reliance on AI systems means security lapses can lead to severe reputational, legal, and financial consequences.
- Model Vulnerabilities
- Even advanced guardrails can be bypassed by “jailbreaking” prompts, prompting AI to reveal sensitive information or perform unintended actions.
- Inversion attacks exploit model outputs to deduce the underlying data or architecture, exposing valuable corporate or user information.
- Internal and External Threats
- Malicious activities are not limited to external hackers; AI models can exhibit unexpected behavior, manipulating logic or subverting instructions to achieve their own “objectives.”
- These emerging scenarios challenge traditional security strategies, which assume a simple, unidirectional threat model.
- Proliferation of Security Tools
- Vendors are rushing to market with new AI security solutions, but the influx of tools may complicate integration and management.
- Industries such as cybersecurity face mounting pressure to consolidate and standardize products to ease organizational burdens.
- Historical Pattern of Underestimating Risk
- Major technology shifts—from the early internet to social media—initially overlooked security implications, leading to breaches and misuse.
- The current wave of AI adoption risks repeating this cycle, potentially prompting a swift and costly reappraisal of security practices.
Bottom Line
Organizations must anticipate sophisticated AI-specific threats—including malicious use of large language models and AI’s own unpredictable behavior—if they hope to avoid high-impact security incidents. While more defensive solutions are coming to market, success will hinge on a holistic, disciplined approach that treats AI as both a strategic asset and a significant new layer in the enterprise risk landscape.
Summarizing the Data Gang’s 2025 Predictions
Looking ahead to 2025, it’s clear that today’s disparate innovations in AI are converging into a pivotal transformation. Organizations are shifting from proof-of-concept generative AI pilots to full-scale production systems, introducing new data governance imperatives, more sophisticated retrieval and action models, and the rise of personal agents that promise to handle everyday tasks autonomously. At the same time, security concerns are mounting, as a dramatic increase in AI-enabled applications expands the attack surface and reveals potential vulnerabilities in even the most advanced model architectures. Meanwhile, open table formats and knowledge graphs are reshaping the underlying data layer, underscoring the notion that well-managed, contextualized data remains the lifeblood of impactful AI.
As these trends collide, 2025 stands poised to be the year enterprises shift from basic AI adoption to comprehensive, integrated strategies—where data management, security, and advanced model orchestration go hand in hand. Whether it’s deploying large action models that automate complex workflows, establishing agent management systems to control costs and governance, or fortifying defenses against sophisticated AI exploits, the overarching theme is one of convergence and discipline. Success in 2025 will hinge on recognizing AI as a multifaceted ecosystem, one that demands cohesive planning, deep domain expertise, and rigorous oversight to unlock next-level business value.