At last week’s AI Agent Builder Summit, hosted by Scott Hebner of theCUBE Research, the hype around “agentic AI” – autonomous software agents that can orchestrate complex tasks – was on full display. Demos and keynotes painted a bold vision of AI-driven business processes. But scratch beneath the surface, and a sobering reality emerges– Most enterprises are far from ready to reap the benefits of fully autonomous agents. Much work needs to be done to realize the full promise of agentic systems, including organizational alignment to create a true data culture, cleaning up data silos, harmonizing that data, assigning data ownership, data product thinking, getting governance and security right, choosing technology partners, rationalizing SaaS and on-prem applications portfolios, and of course change management to get all this done. Starting the journey toward an agentic enterprise is an exciting imperative, but in our view will take the better part of a decade to realize its full promise.
In this Breaking Analysis, we put the summit’s optimism into context, examine why enterprise adoption of agentic AI is on a slower trajectory than the hype would imply, and update our “yellow brick road” to agentic – i.e. the stepping stones required to get to the promised land of agentic automation.
Executive Summary – TL;DR (The Five Headlines)
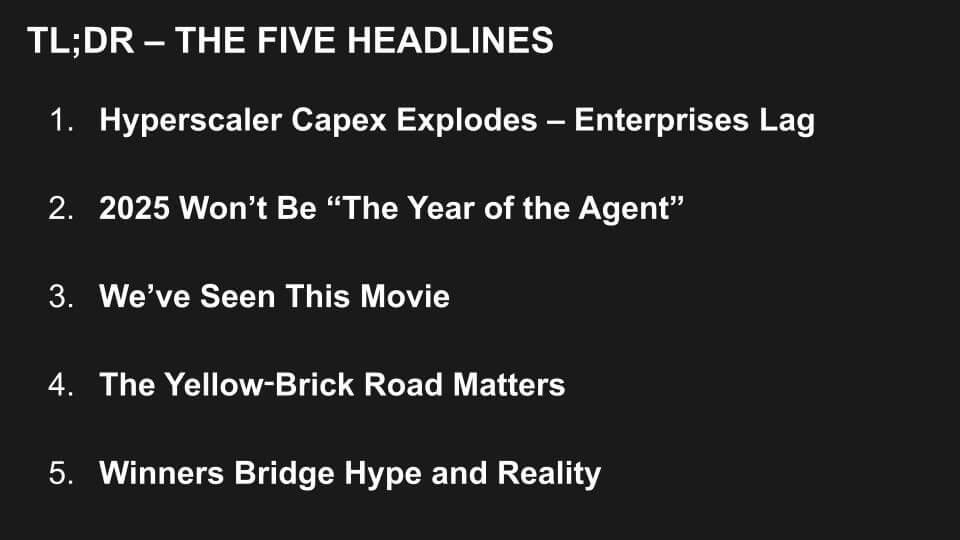
- Hyperscaler Capex Explodes – Enterprises Lag: Cloud giants are pouring unprecedented capital into AI infrastructure, but most enterprises are far behind in building comparable capabilities.
- 2025 Won’t Be “The Year of the Agent”: Despite the buzz around autonomous AI agents, enterprises aren’t ready for wide deployment of “agentic AI” at scale in 2025 – fundamental groundwork is still missing.
- We’ve Seen This Movie: The hype around AI agents echoes past tech waves (e.g. big data) where enthusiasm outpaced reality. Eventually value emerged, but only for a narrow set of firms that had the talent to pull it off. And even then, for most organizations, the promise wasn’t fulfilled, even after years of maturation and hard lessons on data and integration.
- The Yellow‑Brick Road Matters: Reaching true agentic AI is a journey with critical stepping stones. Companies must follow a structured path (a “yellow brick road” of data foundations, integration, and governance) rather than expecting instant magic from AI.
- Winners Bridge Hype and Reality: The organizations that will win in the agentic AI era are those that embrace the vision and invest in practical steps. These winners methodically close the gap between AI hype and enterprise reality, while others fall victim to unrealistic expectations.
Data Center Supercycle
We often talk about the data center supercycle. People don’t like that word, but of course, we coined the term supercloud. We love super, supercycle, super cloud, super chips. It’s all fun. But the fact is the data center supercycle showed itself in earnest last year as shown below ( (i.e. data center spending on power, cooling, compute, storage, networking, core infrastructure).
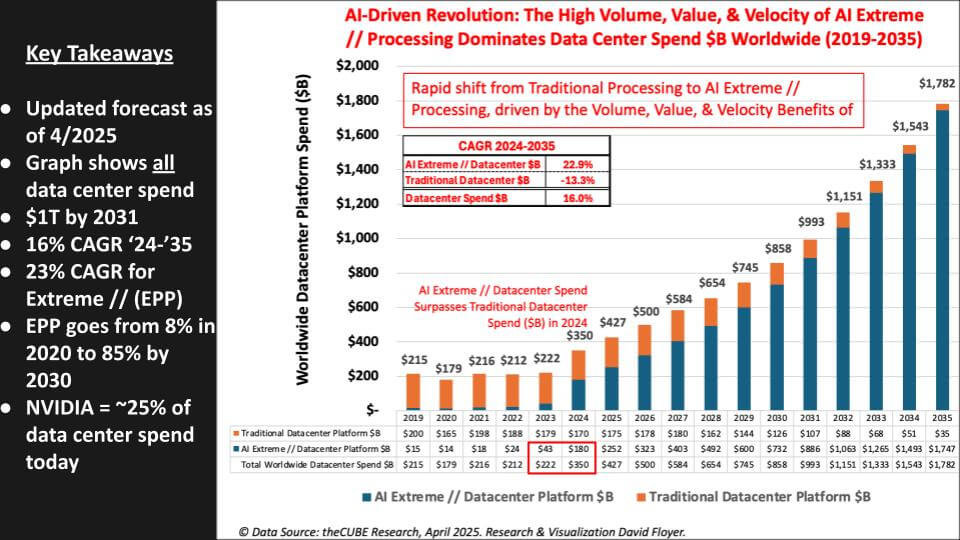
Hyperscalers are driving a rapid shift in data center spending from traditional systems (organge) to AI-focused “extreme parallel” infrastructure (blue). Our latest forecast shows total worldwide data center platform spend approaching $1 trillion around 2031, growing ~16% CAGR. The AI-specific portion (blue) is expanding at ~23% CAGR, effectively taking over the data center by 2030. Traditional (non-AI) data center spend is shrinking (–13% CAGR). In 2024, AI-oriented investments surpassed traditional IT infrastructure spend for the first time. By 2030, about 85% of all data center dollars will be going toward AI/“extreme” processing. NVIDIA alone accounts for roughly 25% of data center spend today by our estimates – an astonishing figure that highlights how much of current infrastructure investment is tied to AI acceleration.
What was perpetually a roughly $200 billion business growing in the low to mid-single digits, exploded in 2024 to $350B (see the highlights in red). That represents a 58% growth rate in a single year, which is going to continue at an accelerated pace for a decade. Now, think about that. The market is growing from around 200 billion to a trillion in just over a decade. Data center spend goes from that low-single digits to a CAGR of 16% over that period, and the AI portion of that grows in the mid- 20s. Meanwhile, infrastructure, to support traditional workloads, i.e. X86, declined steadily. You can see that in the brown bars.
So let’s step back.
Macro View: Hype vs. Reality in Enterprise AI
The tech world is buzzing about “agentic AI” – systems that can autonomously execute tasks, learn, and adapt. Startups and VCs hype these AI agents as the next revolution in productivity. Hyperscale cloud providers (the Amazons, Microsofts, and Googles of the world) are certainly convinced– they are doubling down on massive capital expenditures to build out AI supercomputing infrastructure. In contrast, traditional enterprises are taking a more cautious stance. While cloud giants’ spending on AI infrastructure is exploding, most enterprises lag in adopting these capabilities, constrained by practical realities of budgets, skills, and legacy systems. In short, there’s a widening gap between the hype of what AI agents promise and the reality of what enterprises can feasibly implement today.
We’ve seen this pattern before. From the dot-com era to cloud computing and big data analytics, new technologies often spark feverish excitement and bold predictions. In the case of cloud, mobile and social, the transformative impact did come – but somewhat later than initially expected, after the technology matured and organizations did the unglamorous work to make it enterprise-ready. Big data however never really lived up to its expectations.
Agentic AI is following a similar script– Enormous long-term potential, but with an adoption curve that will be more gradual than the current frenzy suggests. In 2025, for instance, we will not see broad adoption of AI agents run the enterprise on autopilot. Rather, it’s the year when forward-thinking companies lay down bricks on the yellow road toward that eventual destination. The question is will agentic end up living up to the hype. We think it will for those companies that do the hard work.
Hyperscaler Capex Explodes – Enterprises Lag
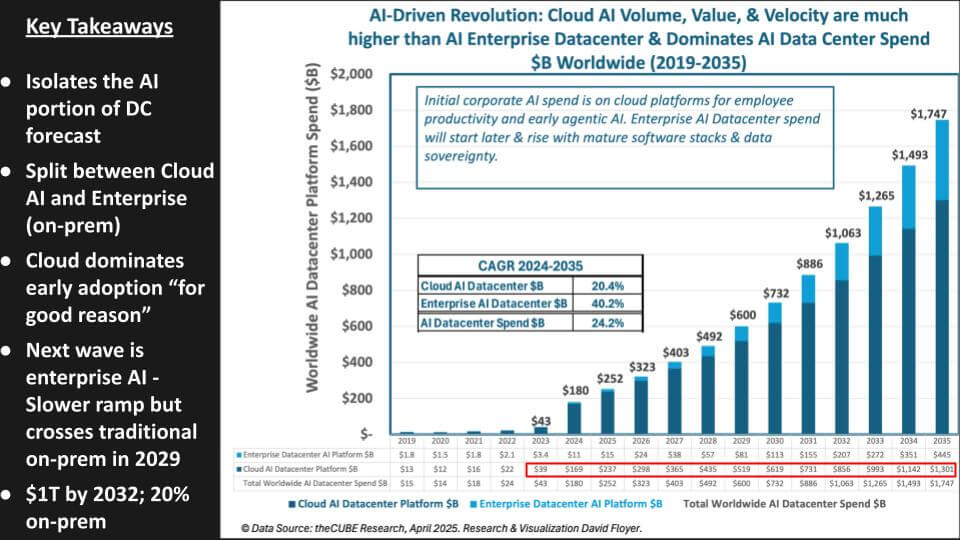
The above chart underscores how aggressively hyperscalers are betting on AI. The bars carve out the AI portion of the previous chart (i.e. AI-centric compute, largely GPU-accelerated systems and specialized silicon). The dark blue bars represent the portion of the market supported by hyperscalers (including Neo Clous), whereas the light blue represents enterprise on-prem (including colocation) activity. In practical terms, this means the cloud titans are retooling their data centers to be AI-first. They’re investing billions in GPUs, custom AI chips, high-speed networks, liquid cooling and expanded facilities to handle the volume, value and velocity of AI workloads. This hyperscaler-fueled buildout is driving overall tech CapEx to new heights.
While cloud providers race ahead, enterprise IT organizations lag behind. Few enterprises can match the hyperscalers’ level of investment or expertise in AI infrastructure. Most corporate data centers still run primarily traditional workloads on CPU-centric systems in air cooled data centers. Enterprise spending on AI gear, though growing, is a fraction of hyperscalers’ outlays. The result is a widening capability gap. Large cloud firms are amassing modern AI capacity (which enterprise customers then rent as cloud services), whereas enterprises’ own environments are not nearly as AI-ready. In effect, the cloud is where AI innovation happens first, leaving on-premises enterprise environments playing catch-up.
The implications are twofold. First, enterprises will lean heavily on cloud platforms in the near term for AI experimentation and deployments– they have little choice, given the cost and complexity of building in-house AI infrastructure. Second, the enterprise lag means we won’t see AI agents broadly operationalized in private data centers for several years. The infrastructure on which agentic AI thrives (massively parallel compute, specialized processors, AI-ready data pipelines) is still being built out in most enterprises. Until that foundation is in place, the grand visions of autonomous agents remain largely confined to cloud labs and pilot projects.
2025 Won’t Be “The Year of the Agent”
If you listen to the hype, you’d think fully autonomous AI assistants are poised to take over office work in 2025. We’ve heard talk of AI agents scheduling meetings, managing workflows, responding to customers autonomously – essentially, 2025 heralding the arrival of “self-driving business processes.” The reality on the ground in enterprises is far more sober. Yes, interest in generative AI and agents is sky-high, and many organizations are experimenting. But when it comes to real production use of agentic AI, most enterprises are just not there yet. The basic ingredients needed to deploy reliable AI agents at scale – high-quality unified data, deeply integrated systems, strong governance – are missing in many cases. As a result, 2025 will be a year of learning and laying groundwork, not a year where AI agents run rampant across the enterprise.
One clear indicator of the maturity gap is how companies are using AI today. A recent Enterprise Technology Research (ETR) survey of IT decision-makers reveals that most organizations are currently engaging with large language models (LLMs) in a very limited way – primarily via consumption of third-party tools and APIs, rather than building their own advanced AI solutions.
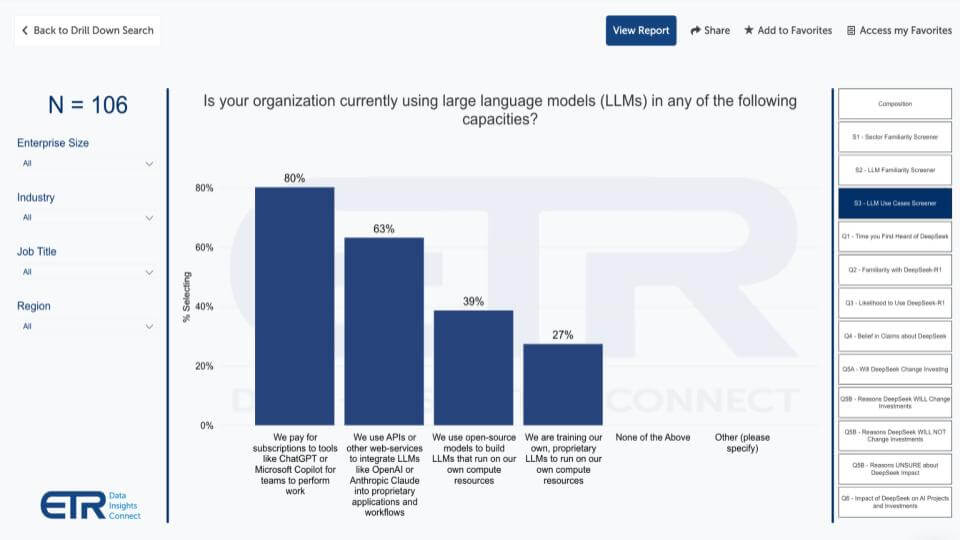
Enterprise adoption of AI today skews toward light consumption rather than deep production integration. In an ETR survey (N=106), 80% of organizations said they “pay for subscriptions to tools like ChatGPT or Microsoft Copilot” to let their teams experiment or perform work. 63% are tapping cloud AI APIs (e.g. OpenAI, Anthropic) to integrate LLM capabilities into some workflows. Meanwhile, only 39% are using open-source models on their own infrastructure, and a mere 27% are training proprietary LLMs in-house. In other words, the vast majority are consuming AI (often via cloud services), whereas far fewer are creating or operating their own AI at scale.
This data paints a clear picture– enterprises remain in the early, exploratory phase with generative AI. Most are content to leverage SaaS offerings or API services for AI capabilities – things like using ChatGPT for copywriting, or plugging an OpenAI API into a customer support app. These are relatively easy on-ramps to get value from AI quickly, but they’re a far cry from having fully autonomous AI agents deeply woven into business processes. Only a minority of companies are building their own models or significantly customizing AI systems on their own infrastructure. Training a proprietary LLM, for example, requires enormous data readiness and ML Ops maturity that few firms have right now. The upshot: for most enterprises, AI adoption in 2025 means consuming pre-built AI services, not standing up their own agentic AI platforms.
Why aren’t enterprises further along? There are three big gaps between the hype of agentic AI and the reality in enterprises today:
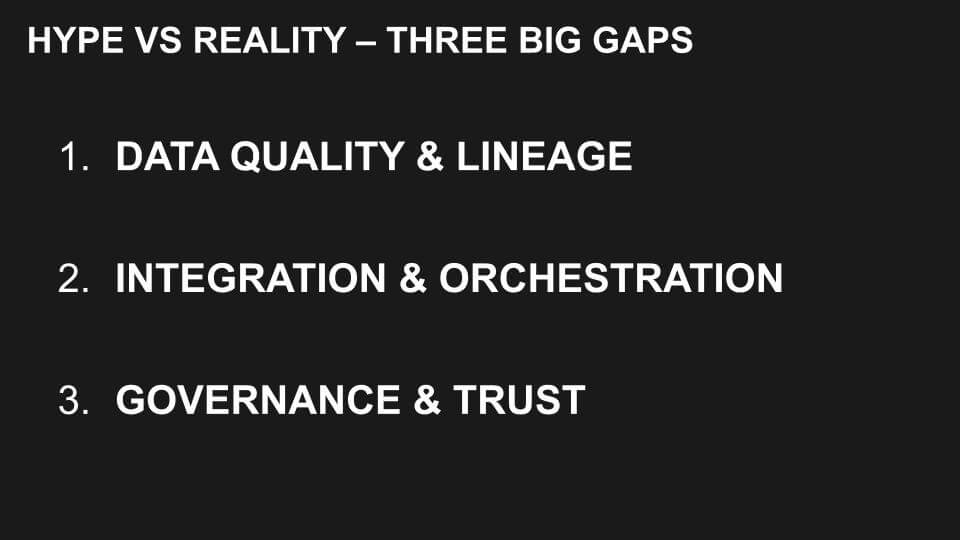
- Data Quality & Lineage: AI agents are only as good as the data feeding them. Most organizations’ data is not in great shape – it’s scattered, siloed, unclean, and lacking consistent lineage. Without a clean, unified data corpus (with known provenance), autonomous AI outputs can’t be trusted. Many firms are discovering that their data foundation needs serious work before they can unleash AI broadly.
- Integration & Orchestration: For an AI agent to be truly useful, it must plug into a multitude of enterprise systems, APIs, and workflows – often in real time. Achieving this integration and orchestration is incredibly challenging. Enterprises have complex, heterogeneous IT environments (ERP, CRM, custom apps, etc.). Today’s AI pilots often operate in isolation (a chatbot here, an RPA automation there). The heavy lifting to connect AI agents seamlessly into end-to-end business processes (so they can act across different tools and datasets) is still ahead of us.
- Governance & Trust: Enterprises have strict requirements around security, compliance, and oversight. An AI agent that unpredictably produces incorrect or biased results can do real damage. Right now, organizations lack robust governance frameworks for AI. Questions of accountability (who is responsible if the AI makes a bad decision?), transparency (can we explain why it acted a certain way?), and ethical use are unsettled. Until governance and trust mechanisms catch up, enterprises will rightly limit where autonomous agents are allowed to roam.
Because of these gaps, we believe predictions that “this is the year of the autonomous agent” in the enterprise are premature. 2025 will see lots of experimentation with generative AI, single copilots, in SaaS use cases (e.g. Salesforce) and maybe some narrow agent-like use cases, but broad deployment of agentic AI across the enterprise is still a few years out. Enterprise tech leaders we speak with are excited about the possibilities – automating customer service, IT operations, marketing campaigns, etc-– yet they also acknowledge the current limitations. The smart ones are not betting the farm on immediate AI magic. Instead, they’re formulating plans to methodically address data issues, integration plumbing, and governance first, so that they can safely take advantage of agentic AI when it’s truly ready for prime time.
We’ve Seen This Movie
If all this sounds familiar, it’s because we’ve lived through similar hype-realism cycles in technology before. Think back to the early days of big data about a decade ago– Hadoop and NoSQL were the rage, and many proclaimed that data lakes would instantly make every company data-driven. What actually happened? Companies plunged in without preparing their data governance or figuring out integration, and many big data projects failed to deliver value. It took years of iteration (and new technologies like cloud data warehouses and lakehouses) to finally tame big data and extract real ROI. The lesson– new tech breakthroughs may eventually pay off, but usually later than the height of hype and often they don’t live up to the vendor promises.
We see agentic AI following a similar trajectory with similar risks. Early excitement and prototypes now; a bit of disappointment or backlash when results don’t immediately match the hype; then a gradual, step-by-step maturation; and eventually, widespread transformational use – but likely in the latter part of this decade. Understanding this pattern is important for setting expectations. Enterprises should temper the “AI agents will change everything overnight” narrative with a dose of realism– it’s going to be a long journey. But as with cloud, if you navigate that journey wisely, the destination can absolutely be worth it.
The Yellow‑Brick Road Matters (The Journey to Agentic AI)
To get from here to the promised land of pervasive agentic AI, enterprises must embark on a journey – a modern-day “yellow brick road” with no shortcuts. Just as Dorothy had to follow the Yellow Brick Road to reach the Emerald City in The Wizard of Oz, organizations have to traverse a sequence of steps to reach the Emerald City of AI-driven automation. Each step builds on the previous one, laying critical foundation for the next. In our view, this journey matters immensely – perhaps more than the end goal itself – because skipping steps will almost certainly lead to disappointment (or a trip back to square one).
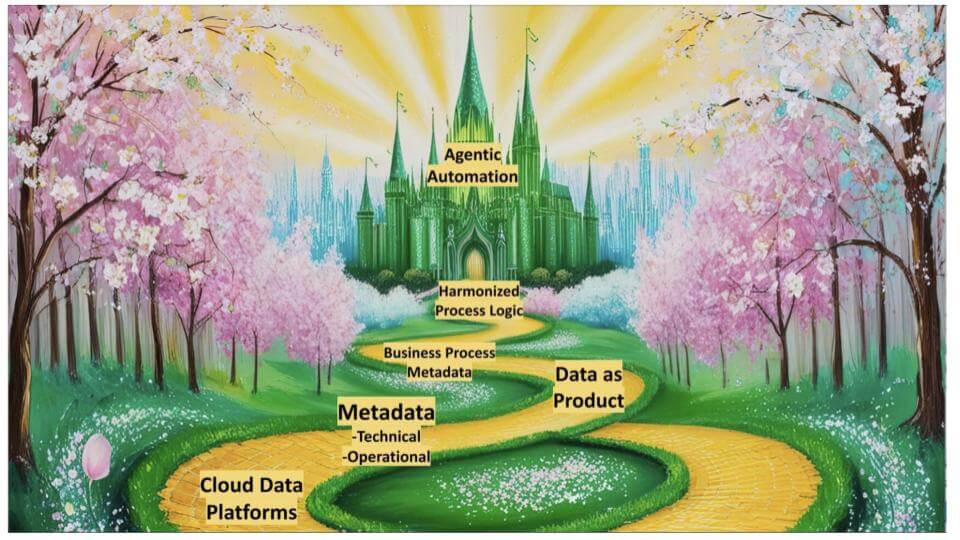
The metaphor of a “Yellow Brick Road” to agentic AI is shown here. Just as a strong road has stepping stones, a strong AI foundation has sequential layers. At the base, companies need modern data platforms (to aggregate and scale data). On top of that comes robust metadata (technical and operational) and treating data as a product, which together ensure data is well-understood and trustworthy. Further up, firms must map and align business process metadata and logic with that data (think of this as capturing process metadata and harmonizing workflows). Only with those pieces in place can true agentic automation – AI-driven processes with minimal human intervention – be achieved (the Emerald City at the road’s end with the Wizard behind the curtain).
Following this yellow brick road means building capabilities in a logical order. An organization that tries to deploy advanced AI agents without first cleaning up its data and processes is a shortcut that we don’t believe will get you to a desired state. By contrast, firms that progress step by step will find that each milestone makes the next one easier. For example, establishing a cloud-scale data platform – and similar on-prem / hybrid capability – along with solid data governance early on – will make it much simpler to integrate AI into business workflows later (because the data is ready, available and trusted). Adopting an “AI-ready” mindset for process design now (documenting processes, APIs, and metadata thoroughly) will pay dividends when you introduce AI agents who need to understand and interact with those processes.
In essence, the road to agentic AI is a maturity curve. You can’t jump from a basic automation today straight to an fully autonomous AI agent tomorrow. But you can plot your course and steadily move upward. We break this journey into five key stages – think of these as the major milestones or waypoints on the road. Below, we outline this five-step playbook that enterprise customers can follow to travel the path toward agentic AI.
Customer Playbook – The Big Five (Steps on the Road)
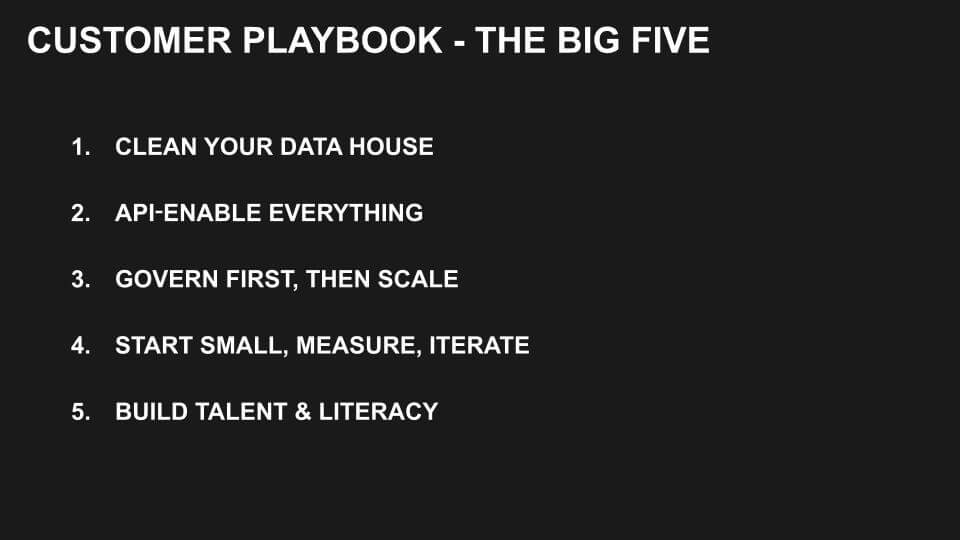
1. Clean Your Data House: Get your data in order before chasing AI magic. This means consolidating siloed data, cleaning and de-duplicating it, and establishing strong data lineage practices. Invest in data cataloging and a unified metadata model so you know what data you have and can trust its accuracy. By creating a single source of truth (or as close to it as possible), you set the foundation upon which AI agents can reason. This step addresses the fundamental data quality gap – without high-quality, well-understood data, any AI initiative will be built on sand.
2. API‑Enable Everything: Make your systems interoperable and accessible. Agentic AI thrives in a connected environment. Enterprises should expose core business functions and data through APIs and microservices. Modernize legacy systems with API layers or integration platforms. The goal is to turn your organization’s processes into a set of Lego blocks (services) that can be programmatically orchestrated. This way, when you introduce AI agents, they can easily plug into different systems to retrieve information or execute transactions. API-enablement goes hand-in-hand with a data mesh principles and builds on past service-oriented architectures, and it directly addresses the integration/orchestration gap. In short, ensure your AI can talk to everything it needs to.
3. Govern First, Then Scale: Establish AI governance, ethics, and security upfront. Don’t wait for a public fiasco to think about AI oversight. Set up an AI governance committee or include AI in your data governance frameworks now, even if your AI use is small. Define policies for responsible AI use, data privacy, and risk management. Implement model monitoring and validation processes to keep AI output in check. By building trust and guardrails early, you create an environment where scaling AI won’t result in chaos or compliance violations. This step is about instilling confidence – for both stakeholders and regulators – that as you do more with AI, you’re doing it safely and accountably. Only once these governance pillars are in place should you accelerate broad AI deployment. In other words, get your rules of the road set before speeding up.
4. Start Small, Measure, Iterate: Don’t attempt a big-bang AI transformation; begin with targeted projects. Identify low-risk, high-value pilot use cases for AI and agents. For example, automating a single workflow in IT support, or deploying a chatbot for a specific customer service tier. Keep the initial scope narrow, but do it end-to-end – ensure the project touches real data, real systems, and real users, so you can learn from the full process. Measure the outcomes (e.g. time saved, resolution rates, user satisfaction) and iterate based on feedback. This agile approach allows you to build experience and capability incrementally. Early wins will also help garner buy-in across the organization. By starting small, you avoid costly failures and learn what works in your context, then you can expand the circle gradually. Remember, the journey is iterative – each loop of learn-and-improve gets you closer to the ultimate vision.
5. Build Talent & Literacy: Invest in your people – both experts and the general workforce. The fancy term “agentic AI” won’t mean much if your teams lack the skills to implement and use it. Address the talent gap by training or hiring for key roles– i.e. Data engineers, ML engineers, prompt engineers, AI modelers, etc. Simultaneously, drive AI literacy among your broader employee base and leadership. Educate non-technical stakeholders about what AI can and cannot do, and how to interpret its outputs. Developing internal champions who understand the business and the technology will help bridge communication between IT and business units for AI projects. Culturally, encourage experimentation and re-skilling – the organizations that learn fastest will adapt fastest. In the end, AI is not a plug-and-play tool; it’s a capability that your organization must grow. Human talent is the soil in which that capability takes root.
Each of these five steps directly addresses the hype-vs-reality gaps we identified. By cleaning your data and API-enabling your environment, you tackle the data quality and integration challenges that currently hold back AI. By instituting governance and starting with measured pilots, you ensure trust and gradual progress, avoiding the reckless hype-driven rush that leads to failure. And by cultivating talent, you give your organization the skills to actually execute on the promise of agentic AI. Think of this playbook as laying down the bricks on your yellow brick road – with each brick in place, the path to the AI-enabled future becomes clearer and more achievable.
The Bottom Line– Bridging Hype and Reality
We believe that agentic AI has the potential to fundamentally change how businesses operate– driving 10X productivity for organizations. The idea of software agents autonomously handling complex tasks is no longer science fiction – it’s on the horizon. But the journey to get there will be long and challenging for enterprises. The bottom line is that success with agentic AI will come to those who bridge the gap between hype and reality. That means keeping one foot in the future and one foot in the present (run and grow the business) while you work on transforming your organization. We firmly believe you should embrace the vision– i.e. experiment with the latest AI innovations, imagine new business models they enable, and take some calculated risks. But you must equally invest in the unsexy work– i.e. Cleaning data, data integration, setting governance policies, and educating teams. This is the bridge that connects today’s reality to tomorrow’s possibilities.
The companies that do this – i.e. balance excitement with execution – will be the ultimate winners of the agentic AI era. They will be positioned to leap ahead of competitors when AI agents finally mature, because they’ll have the infrastructure and culture ready to support them. In contrast, those who either dismiss the trend as pure hype or dive in blindly without preparation will find themselves disappointed.
Our advice to enterprise leaders is to take the long view. Agentic AI is a long journey, not a quick trip. Use the current hype as motivation and momentum to get organizational buy-in, but set realistic expectations internally. Focus the narrative on what your team needs to do to make AI real (the playbook steps), not just on the shiny outcomes. By doing so, you create a culture that is excited about AI and grounded in executing it properly.
In summary, the road to agentic AI is indeed long, with many twists and turns. But with a pragmatic strategy, that road is navigable. The hype will eventually give way to solid enterprise gains for organizations that properly prepare. The Emerald City of automation awaits at the end of the yellow brick road, but those organizations who earn their way there and do the hard work will get to unlock its full value ahead of the competition. The time to start paving your road is now but do so with an understanding of what it will take to reach your destination.
What do you think? Is your organization ready for agentic AI? Are you having success in pockets (e.g. SaaS) and is that encouraging? Are you worried about AI “stovepipes?” How are you dealing with data silos and what’s your strategy for governing open data?
Let us know.