Thirty months into the GenAI awakening, the jury is still out on how much enterprises are benefiting from investments in artificial intelligence. While the vast majority of customers continue to spend on AI, reported returns are no greater than, and frankly lag those, typically associated with historical IT initiatives like ERP, data warehousing and cloud computing. Let’s face it, the multi-hundred billion dollar annual CAPEX outlay from hyperscalers and sovereign nations is fueling the euphoria and essentially supporting blind faith in the AI movement. But ground truth returns from enterprise AI adoption remain opaque. This combined with geopolitical unrest, fluctuating public policy and GDP estimates in the low single digits, have buyers tempering expectations for tech spending relative to January of this year.
In this Breaking Analysis we review the latest data on enterprise AI adoption in the context of overall IT spending. We’ll also drill into some of the dynamics of AI usage and give you a small glimpse of some leading edge AI product research conducted by ETR.
Internal AI App/Dev is Grabbing Budget
We believe the latest cut of ETR’s AI Product Series, shown below, confirms a decisive budget shift toward building in-house AI applications. The survey, fielded to 600 IT decision makers who are actively developing and maintaining proprietary AI applications, finds that 83 percent expect to raise spend in 2025, with most projecting double-digit increases. Only 5 percent foresee any pullback. ETR notes that a companion January survey of broader “buy-and-build” AI programs delivered a similarly bullish read (75 percent net-positive), underscoring that whether enterprises are purchasing SaaS or crafting code themselves, AI App/Dev is a budget priority.
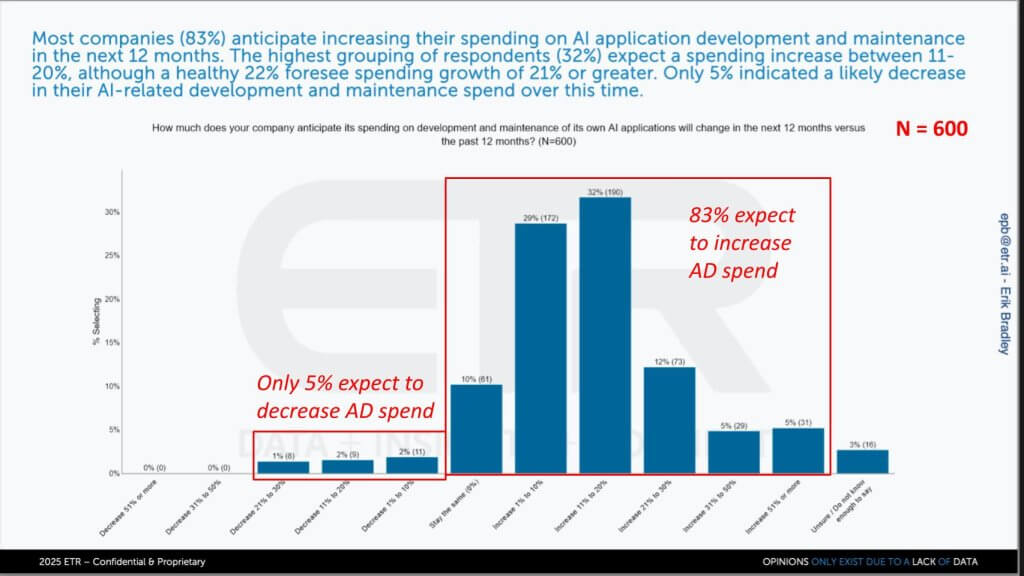
Key Findings
- Double-digit spending commitment: Our research indicates that the majority of respondents are materially expanding budgets to accelerate time-to-value for custom AI workloads.
- Consistency across buyer types: A prior, all-inclusive AI study showed nearly identical enthusiasm, signaling that demand is robust for both commercial SaaS and bespoke development paths.
- Early-cycle headroom: We believe these spending intentions represent the front end of a multi-year investment wave, given that most enterprises are still in proof-of-concept or early production stages.
- Low downside risk: With just 5 percent citing budget cuts, the data suggest AI application development is somewhat insulated from broader IT spending scrutiny. We recognize that can change with sentiment shifts.
- Cadence of validation: ETR’s regular pulse will allow us to track inflections quickly; our expectation is that the next read-out will sustain or even exceed today’s optimism; with the caveat that if ROI remains opaque (which we’ll discuss below) and public policy uncertainty remains, the sanguine sentiment could flip.
Nonetheless, in our opinion, the chart’s right-skewed distribution of responses illustrates a market that is still in acceleration mode and nowhere close to saturation. The implication is vendors supplying tooling, runtime platforms, and talent to in-house AI teams will likely see sustained tailwinds through 2026 and beyond.
Regulatory Crosswinds Haven’t Broken AI’s Momentum
The chart below paints a picture suggesting geopolitical tension and shifting policy frameworks are not derailing enterprise AI agendas. In ETR’s April 2025 macro survey of 507 leaders, half report they are either holding steady or accelerating AI programs in spite of tightening policies. Only 10 percent are tapping the brakes.
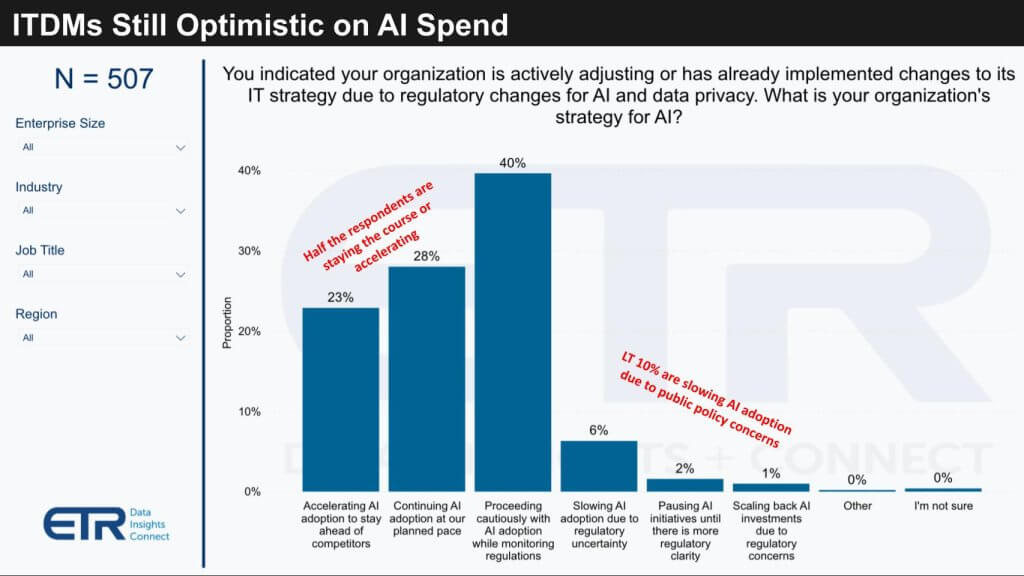
The horizontal bars above depict three cohorts: 1) Enterprises accelerating AI adoption; 2) Enterprises staying the course; and 3) Those slowing adoption. The middle band, labeled “proceeding cautiously,” is the largest at roughly 40 percent, while the deceleration slice is under 10%. The leftmost bar representing acceleration widens materially when the data is filtered for IT-telco respondents, jumping from 24 percent to 40 percent, with a corresponding lift in the “continuing” category to 26 percent. In our view, the data reinforces the idea that digitally intensive sectors are doubling down, not hedging.
Key Findings
- Resilient demand signals: The data indicates that regulatory uncertainty is viewed more as a governance hurdle than a spending deterrent.
- Sector bifurcation: IT-telco firms display nearly 70 percent positive momentum, suggesting competitive pressure is crowding out caution in tech-centric verticals.
- Healthy skepticism, not stasis: The 40 percent “proceed with caution” cohort reflects disciplined budget stewardship rather than retreat. We believe these organizations are actively piloting use cases while monitoring evolving compliance frameworks. We see this cohort however as similar to the swing vote in elections – if sentiment could change so could their spending patterns.
- Limited downside without a catalyst: With deceleration stuck at 10 percent, the probability of a broad AI investment freeze appears lower. Again, we’ve seen how changes in policy or market conditions can negatively affect budget allocations.
- Optionality remains: Undecided buyers can still tip positive; their budgets are allocated, but release is gated to clearer ROI metrics.
In our opinion, the chart’s asymmetry – i.e. mostly “green” with a narrow “red band” cutting, signals that AI has earned prominent status in 2025 budgets. The prudent middle will either migrate toward acceleration as regulatory guardrails solidify; or stand pat if uncertainty persists. We caution that spending is fickle and often ebbs and flows with economic sentiment.
ROI Lags the Hype, Revealing Immaturity of the AI Cycle
The chart below injects a cautionary note into the otherwise bullish narrative. Thirty months into the generative-AI wave, 27 percent of respondents acknowledge they have yet to see any tangible return on their AI spend. Fewer than 10 percent report an ROI north of 25 percent, the hurdle rate many CFOs use to green-light higher risk IT projects. Even more revealing is that over one-quarter admit they are not tracking ROI at all.
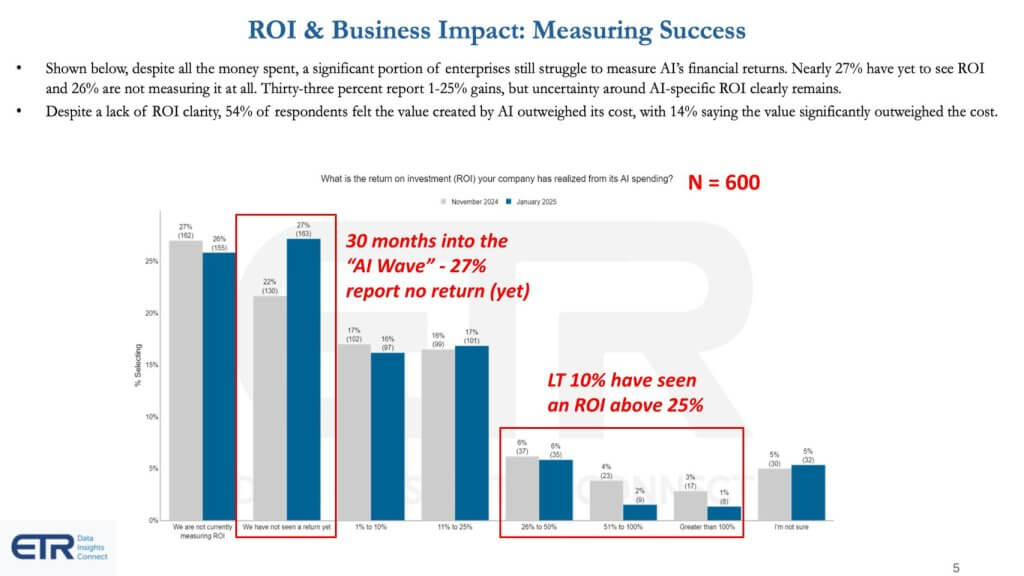
The bar segments above show several ROI bands ranging from Not tracking, Negative/Zero, 0–10 %, 10–25 %, and three bands “>25 %.” The largest cohort are either not measuring or have yet to cross break-even. The data underscores a skew toward unproven or unmeasured outcomes.
Key Findings
- Proof-of-concept phase: Despite vendor narratives to the contrary, our research indicates enterprises are still in experimentation mode, allocating funds to build, test, and learn rather than to harvest immediate returns.
- Budget re-allocation, not expansion: Qualitative feedback and other quantitative survey data suggests AI spending often cannibalizes line-of-business, sales, and marketing budgets, delaying formal ROI scrutiny.
- Deferred accountability: The 27 percent “not tracking” cohort highlights a mindset of “build now, justify later,” especially among firms that maintain their own codebases.
- ROI gap vs. hurdle rates: With sub-10 percent clearing a 25 percent ROI bar, we believe many projects could soon face CFO pressure to articulate value beyond efficiency anecdotes.
- Talent and OPEX offsets: In addition, we note that slower head-count growth is viewed internally as an implicit payback mechanism, perhaps further postponing rigorous financial scrutiny.
Once again we see an asymmetry in the data – i.e. a large cohort at “no return” while tapering sharply at “high return.” In our view this signals that AI remains an early-cycle investment story. The “proceed with caution” middle we highlighted earlier could flip positive once tangible benefits surface; conversely, it could sour if ROI continues to lag expectations or economic sentiment shifts. Either way, the next two survey waves will be pivotal in determining whether optimism converts into measurable economic value.
AI’s is not Immune to Cuts: CFOs will Trim Experimental Spend First
We believe the chart below offers an important reality check. When 219 C-suite executives were asked where they would pull dollars if macro conditions deteriorate further, AI initiatives rank as the second-most “very vulnerable” category—trailing only outsourced IT services and sitting behind security cloud, SaaS and core infrastructure.
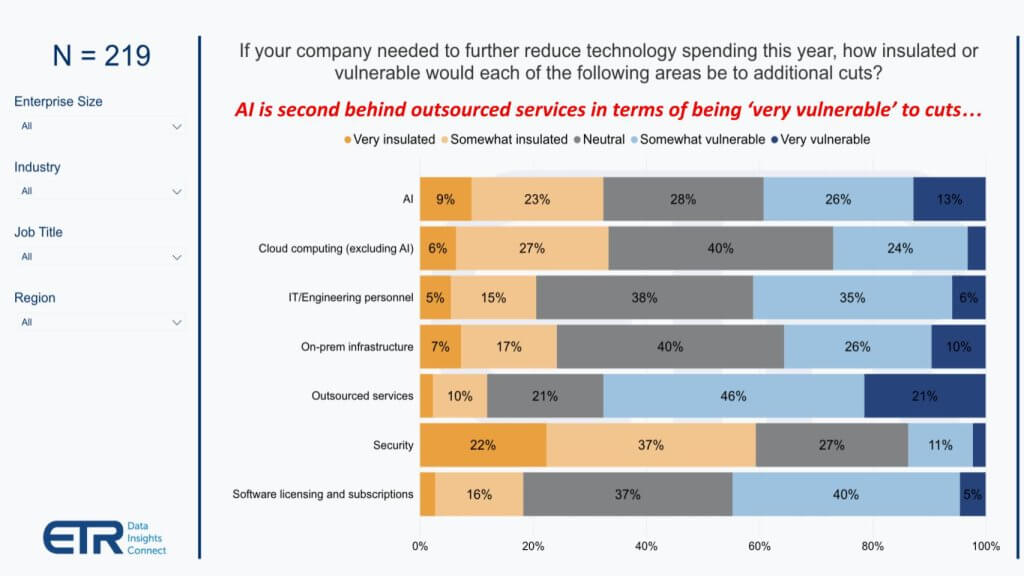
The horizontal bars above rank seven IT domains by share of executives evaluating the vulnerability of each segment to incremental cuts. Outsourced IT services tops the list, followed by AI projects. Security anchors the group with the smallest exposure, while cloud computing, SaaS and foundational infrastructure occupy the middle ground, indicating to us that they are somewhat more insulated.
Key Findings
Observation | Implication |
---|---|
One-third of C-level leaders now expect to reduce 2025 spend more than initially planned. | Discretionary programs will face triage, forcing AI teams to justify initiatives against clearer ROI benchmarks. |
AI’s sandbox status makes it an expedient target. | Unlike cyber defenses or production workloads, AI pilots can be paused without jeopardizing day-to-day operations, making them a politically safe cut for CIOs. |
Security remains most protected. | Our research indicates that compliance mandates and breach headlines continue to shield security budgets even in tightening cycles. |
Cloud and infra enjoy middle-of-the-pack protection along with software. | These categories underpin revenue-bearing digital services; therefore, outright cuts risk revenue leakage. |
Velocity can backfire. | The very speed at which AI spend has ramped invites scrutiny—controllers see an obvious lever when forced to contain cash burn. |
In our opinion, the data reminds investors and practitioners that AI hype does not equal budget immunity. While earlier data documented robust intent to invest, the C-suite view presented above highlights a Caveat. Specifically, if macro headwinds intensify, experimental AI funding could move to the front of the line for cutbacks.
IT Budget Growth Retreats as Macro Sentiment Shifts
The data below captures one of the sharpest one quarter resets in enterprise tech spending since the initial COVID shock. ETR’s April macro pulse shows 2025 IT budget growth expectations plunging from 5.3 percent in January to 3.4 percent, a 190 basis point drop which ETR characterizes as “the biggest drop we’ve ever recorded outside a crisis.”
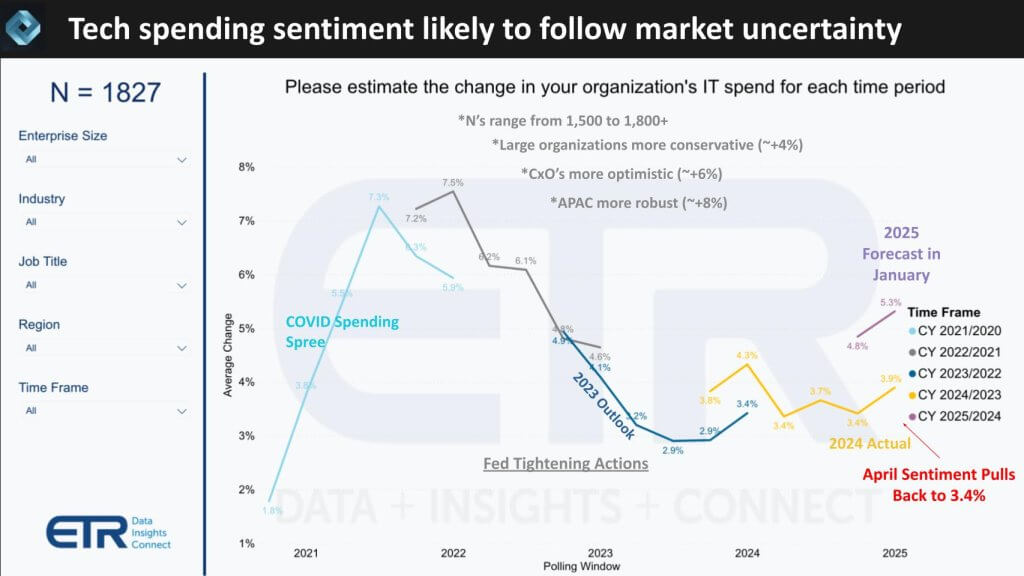
The line graph above tracks sequential quarterly revisions to aggregate IT budget growth, with call-outs for key segments. All three curves slope steeply downward between Q1 and Q2. The mainline average falls to 3.4 percent, while the Fortune 500 and Global 2000 are even less optimistic, looking for 2.4 percent and 2.2 percent, respectively. One footnote is the survey closed one day after reciprocal tariffs were announced, meaning the data miss the subsequent equity market sell-off that likely would have driven estimates even lower. The sample also was taken before the v-shaped rebound that ensued when tariffs were put on temporary hold.
Key Findings
Observation | Implication |
---|---|
Largest quarterly downgrade since early 2020. | Our research indicates sentiment has turned decisively cautious as GDP forecasts slip below 1 percent and rate cuts remain elusive. |
Scale amplifies pain. | Big enterprises—historically the mainspring of IT demand, are trimming the deepest, underscoring a broad-based belt-tightening rather than isolated project delays. |
Correlation with GDP is alive and well. | Contrary to notions of tech spending decoupling from macro cycles, the data reinforce a tight linkage between business outlook and IT outlays. |
Security stays sticky, AI ok (for now). | As we noted in Section 4, experimental AI remains vulnerable, whereas keep-the-lights-on categories (security, SaaS & core infra) enjoy relative protection. |
Watch the July read-out. | A modest market rebound could buoy the next survey, but we believe CFOs will demand clearer ROI articulation before restoring budgets to 5-plus-percent growth trajectories. |
In our opinion, the data’s pronounced downward inflection delivers two messages. First, the post-COVID era of “high-single-digit baseline” IT growth is over for now; budgets have snapped back to pre-pandemic norms. Second, the gravity of macro sentiment is palpable. If GDP stays sub-1 percent, we expect CFOs will continue to recalibrate spend to 2-to-3 percent territory, forcing AI champions to prove value faster or risk funding erosion.
Policy Fog Causes the C-Suite to Tap the Brakes on Net-New IT Projects
The data below is the clearest signal yet that political and trade turbulence is starting to seep into executive decision making. In a flash response cut of 219 C-level executives, 71 percent acknowledge some form of pullback, whether delaying, reducing scope, pausing, or outright canceling AI initiatives, while only 27 percent say uncertainty pushed them to accelerate or green-light new projects.
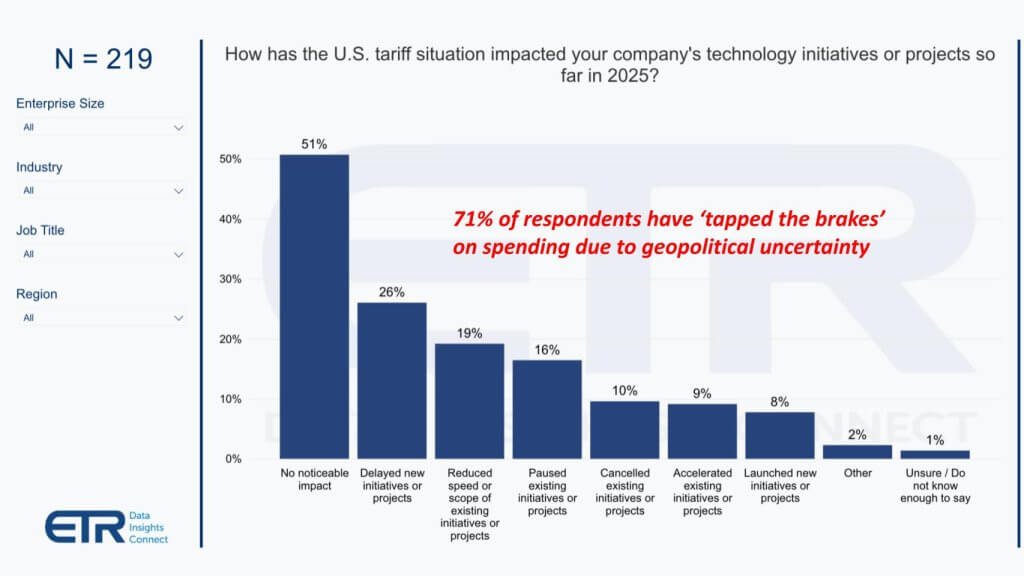
Key Findings
Observation | Implication |
---|---|
Impact is real at the top. Nearly half of C-suite respondents link policy volatility to action, and three-quarters of those actions are defensive. | Our premise is that unless and until ROI become more clear, enterprise AI enthusiasm is subject to board-level gating if the external environment turns negative. |
Delay outpaces cancellation. Most executives are hitting pause, not “stop,” implying pent-up demand could rebound if clarity emerges. | Vendors should prepare for an air-pocket in deal closures Q2–Q3, with a potential catch-up surge late in the year. |
Back-loaded budgets. Consistent with previous analysis, spending expectations are drifting to the fourth quarter, mirroring IPO market hesitancy. | We believe forecasting models should weight Q4 more heavily than historical seasonality would suggest. |
Sandbox perception persists. Experimental AI remains easier to shelve than revenue-bearing workloads, reinforcing the previous section’s points on AI vulnerability. | Teams should reframe pilots as cost-savers or revenue enablers to escape “nice-to-have” status. |
In our opinion, the chart’s lopsided distribution toward delay and down-scoping underscores an emerging tension between boardroom optimism about AI’s transformative potential versus CFO caution in an unsettled macro climate. The next survey wave will reveal whether today’s pause becomes tomorrow’s cancel, or merely a coiled spring set to release once policy headwinds subside.
Enterprise LLM App/Dev Adoption Shows GPT Alone at the Top
The inaugural ETR snapshot of which foundation model families enterprises plan to incorporate into their own code bases provides an early but telling signal- i.e. OpenAI’s GPT commands unprecedented mindshare, with roughly 62 percent of builders already using or planning to use the model. No rival cracks the 35-percent line, underscoring GPT’s first-mover momentum and Microsoft’s distribution advantage through Azure OpenAI Service.
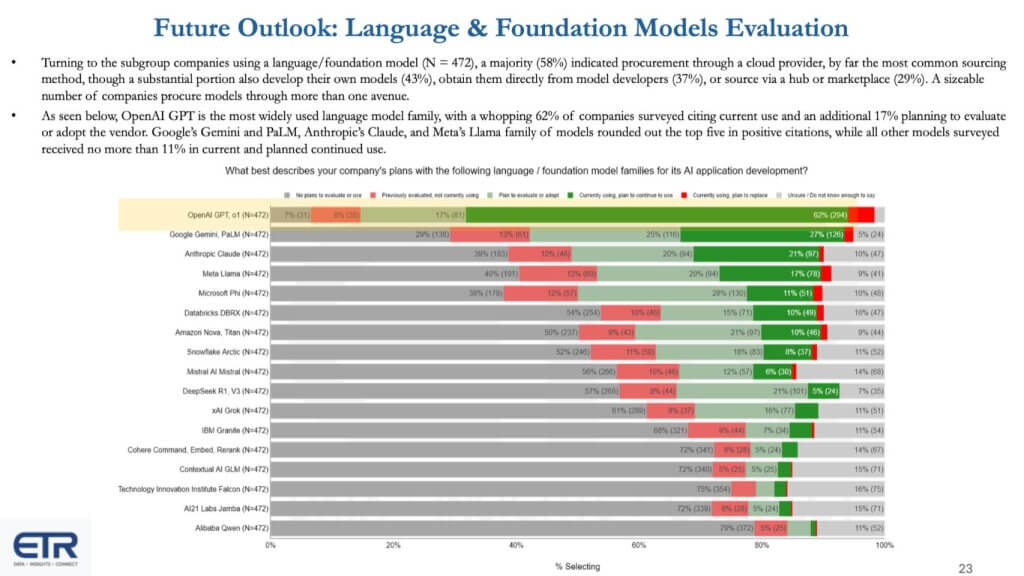
The horizontal bar chart shows more than a dozen model families from highest to lowest “currently using / plan to use” share. GPT’s bar stretches almost two-thirds of the way across the graphic, colored solid green for active use and patterned green for near-term intent. Meanwhile the next cluster (Gemini, Anthropic Claude, Llama, etc.) hovers in the high-20 to low-30 percent range. A tapering long tail follows with Databricks’ DBRX, Amazon’s Nova, Snowflake Arctic, DeepSeek, Grok, IBM Granite, and Cohere each registering lower but notable interest.
Key Findings
App/Dev Signal | Why it Matters |
---|---|
62 % gravitate to GPT | Our research indicates the combination of brand, perceived quality, and seamless Azure access gives OpenAI a wide funnel of enterprise proofs-of-concept. |
Mid-tier is crowded but viable | Gemini, Claude, and Llama demonstrate that quality alternatives already attract a meaningful minority, especially where data-sovereignty, openness requirements or cost constraints apply. |
Data-platform natives make the list | Databricks and Snowflake hit the leader board despite launching later, validating the thesis that proximity to governed data can offset model-scale disadvantages. |
Specialists remain niche—for now | DeepSeek, Grok, Cohere, and IBM Granite collectively sit well below the leaders today, but each targets white-space such as RAG optimization, private deployment, or vertical fine-tuning. |
Differentiation perceived as thin | Seventy-two percent of respondents see “only minor” quality gaps between models, suggesting price, governance, and ecosystem tooling will dictate the next share shift. |
In our opinion, the chart’s steep drop-off from GPT to “everyone else” confirms that distribution and developer experience matter as much as raw model performance in the enterprise race. Yet the presence of nine alternative families, many embedded directly inside data platforms, signals a coming phase of fragmentation and price competition once baseline accuracy converges.
Microsoft Extends Its Lead in AI Development Platforms, but Optionality Remains Critical
We believe the data below illustrates a familiar pattern, i.e. the farther enterprises move up the AI stack, the more dominant Microsoft becomes. When respondents were asked which development platforms they are using or plan to use for building AI applications, three Azure offerings occupy the top three positions.
Rank | Platform | “Using / Plan to Use” Share | Comment |
---|---|---|---|
1 | Azure OpenAI Service | Low- 60s % | Direct pipeline to GPT fuels rapid PoCs |
2 | Azure Machine Learning | High-50s % | Deep Databricks integration, automated MLOps |
3 | Azure AI Studio | Mid-50s % | Low-code glue for vision, speech, and RAG |
Following Microsoft’s dominant adoption, the chart below shows Amazon’s Bedrock (Mid-30s %) and SageMaker (low-30s %) form the second tier, followed by Google Vertex AI in the 30 % range. A mixed long tail rounds out the chart, including Hugging Face, LangChain, Databricks Mosaic AI, Snowflake ML/Cortex, IBM watsonx, Oracle AI, Cloudflare Workers AI, Red Hat OpenShift AI, and H2O.ai.
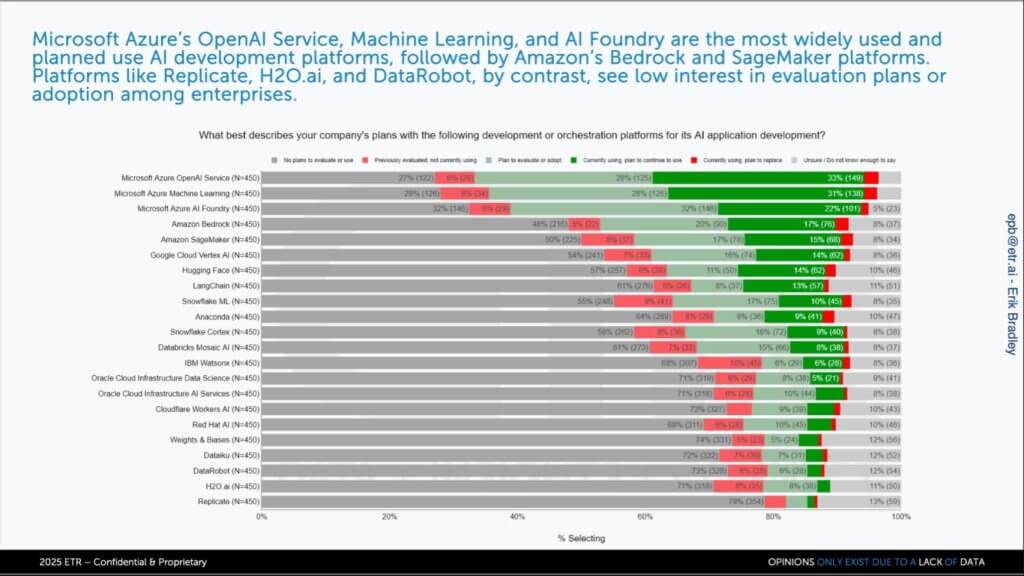
Key Findings
- Azure as de-facto starting point: The data indicates that Microsoft’s simplified hand-off from GPT to ML tooling provides a facile on-ramp that few rivals can currently match, at least in terms of account capture.
- Public-cloud friction is real: CIOs at heavily regulated firms told ETR they cannot put sensitive data in public-cloud AI services, underscoring why on-platform options register meaningful interest.
- AWS shows a two-track strategy: Bedrock’s model garden attracts greenfield generative-AI projects, while SageMaker continues to serve classical ML workloads. Together and combined with likely higher ASPs protect against Azure’s account penetration.
- Google Vertex gains on developer experience: Despite lagging GPT in raw hype, Vertex’s integrated tooling and first-party Gemini access place it firmly in the mix, especially for cloud-native shops.
- Long-tail resilience through proximity to data: We believe offerings embedded directly in data platforms like Snowflake Cortex and Databricks Mosaic, punch above their weight because they minimize data movement and governance overhead.
- Compliance drives optionality: The sheer breadth of vendor logos in the chart reflects enterprise demand for deployment models that span public cloud, dedicated region, on-prem, and hybrid Kubernetes estates.
In our opinion, the data underscores a two-fold conclusion: (1 Microsoft’s end-to-end positioning from model to tooling is paying off; yet (2 The market is far from winner-take-all because data-sovereignty, latency, and existing platform commitments compel a diversified toolkit. Vendors that can meet customers where their data already lives—while abstracting away model orchestration complexity will gain outsized leverage as the AI stack evolves.
Vector Search Has Shifted From Hot Topic to Table Stakes, But the Field Remains Crowded
We believe the data below demonstrates how rapidly vector search has moved from headline innovation to checkbox feature – and how that shift has splintered adoption across a surprisingly wide set of vendors. When asked which vector-search engines they are currently using or plan to use, respondents placed Microsoft’s Azure AI Search narrowly in front, followed by MongoDB’s native Atlas Vector Search and an almost dead-heat between Elasticsearch and Redis. A second cluster comprising Google’s Vertex AI, Amazon Kendra, Databricks’ Vector Store, Snowflake Arctic Vector, and Oracle’s OCI AI Search, occupies the mid-teens, followed by IBM watsonx.data (IBM uses Milvus) and specialist standalone offerings such as Pinecone, Milvus, and Cloudflare Workers AI round out a long tail of single-digit intent.
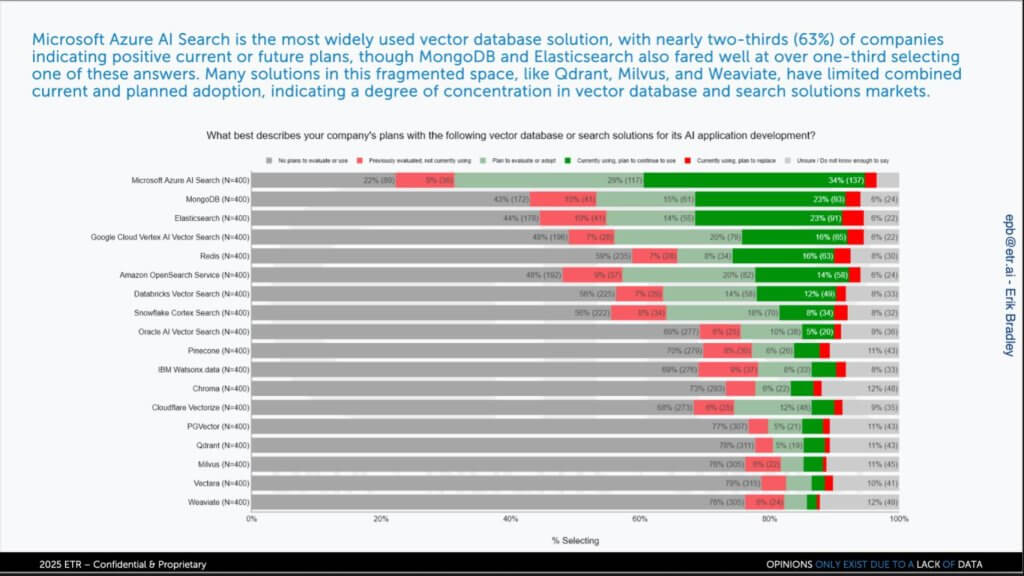
Key Findings
Signal | Implication |
---|---|
Feature-ization of vector search. | The technology is now table stakes; differentiation shifts to latency, scale, and integration with data governance. |
Microsoft tops the chart, but barely. | The date indicates enterprises default to Azure AI Search when already committed to GPT/Azure, yet the margin is slim, evidence of low switching friction. |
MongoDB’s document-plus-vector model resonates. | Early (pre-GA) reviews translated into strong intent, validating that proximity to operational data trumps specialized point solutions for many workloads. |
Redis is the stealth climber. | Redis’ high ranking surprised many, suggesting that in-memory performance for real-time personalization is a compelling niche. |
Elastic maintains relevance through unified search. | Elasticsearch’s observability footprint gives it a built-in vector use case for log analytics and security telemetry. |
Specialists must prove value. | Pinecone, Milvus, and Cloudflare attract experimentation but must differentiate on multi-tenant isolation, hybrid deployment, or cost efficiency to climb the stack. |
In our opinion, the data confirms that vector search has crossed the chasm into “must-have” territory, yet remains far from winner-take-all. Enterprises still evaluate options by asking, Which engine fits my existing data topology and latency SLAs? rather than defaulting to a single cloud offering. We expect consolidation to follow two paths: 1) A deeper embedding of vector indexes inside mainstream databases; and 2) Specialist engines targeting high-throughput, cross-region retrieval-augmented-generation (RAG) at lower cost.
Skills, Data Quality, and Legacy Integration Form Constraints on DIY AI
What the chart shows
A vertical bar chart ranks these hurdles left to right. The first three bars—skills, data quality, legacy integration—tower over the rest, followed by a mid-pack of compliance, budget, and scaling, then a taper toward soft factors such as culture.
The data underscores why large enterprises find do-it-yourself (DIY) AI far harder than the marketing demos imply. In a 600-respondent data cut focused on organizations building their own apps, several obstacles jump out as shown below, led by skills, data quality and integration challenges.
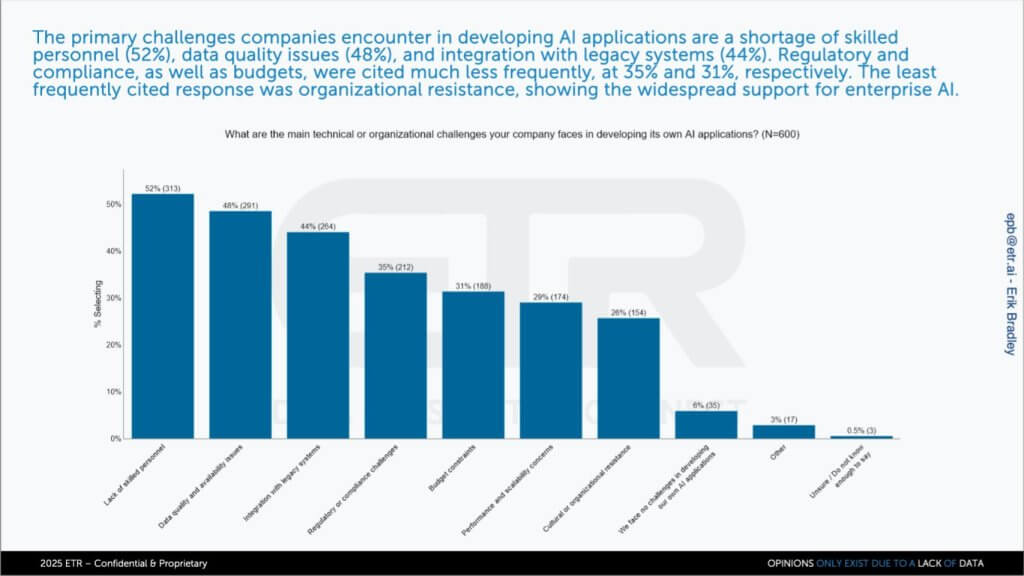
Key Findings
- Build-over-buy skews big-company: Our research indicates Fortune 500 firms prefer DIY stacks for customization and IP control, but that preference amplifies the constraints—talent, data prep, and legacy plumbing—they rate most painful.
- Data remains the gating asset: Without reliable lineage, masking, and vector-friendly schemas, fine-tuned models under-deliver. This in our view validates the idea of “bring AI to the data, not the data to AI” narrative we outlined in prior research (#4 from our 2025 Predictions post).
- Compliance casts a long shadow: Security teams still view public-cloud inference as riskier than on-prem sandboxing, pushing organizations toward private deployments even as GPU scarcity persists.
- Cost is not the primary barrier as of yet: Only 30 percent cite budget as a top-three concern, implying CFO scrutiny will intensify once initial pilots transition to production-scale clusters.
- Talent wars could decide platform winners: Vendors that bundle turnkey MLOps, lineage, and guardrails will siphon demand from DIY pipelines as hiring constraints eat into talent pools.
In our opinion, the data underscores a clear truth – i.e. AI’s limiting factors are less about technological breakthroughs and more about enterprise plumbing. Solving for data hygiene, skills acquisition, and legacy connectivity will matter more to adoption velocity than squeezing a few extra tokens per dollar out of the next model release.
Bring AI to the Data: Deployment Patterns Split Between Cloud Convenience and On-Prem Control
We believe the data below validates a core theme running through this research note – i.e. that most enterprises are bifurcating their AI estates, keeping sensitive workloads close to the data while off-loading everything else to the public cloud.
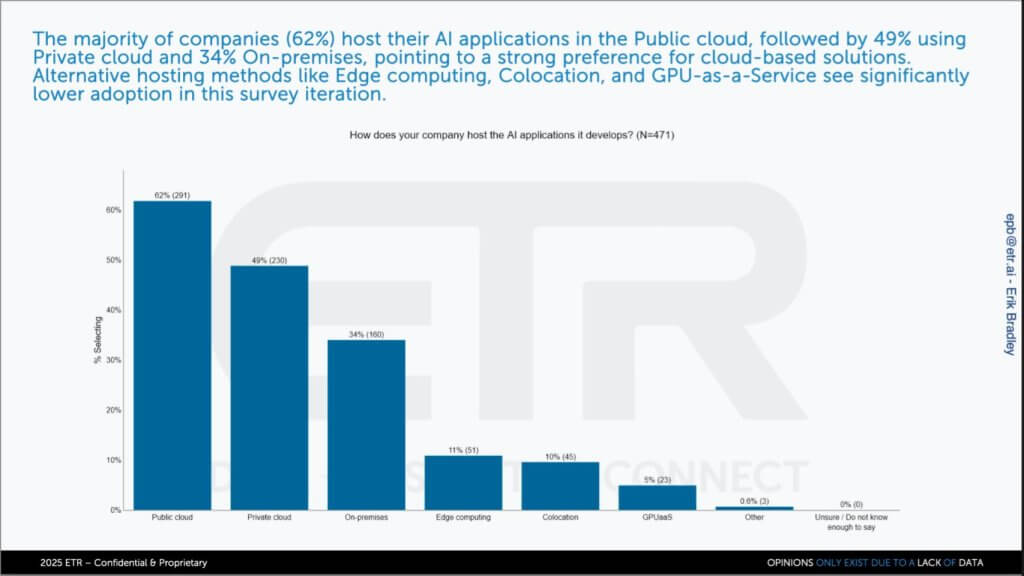
Key Findings
- Cloud dominates—but not universally. Our research indicates that more than half of AI workloads spin up in hyperscaler regions thanks to turnkey tooling, elastic GPUs, and mature MLOps services.
- On-prem remains mission-critical. Roughly one-third of respondents split between private-cloud and legacy on-prem deployments, driven by data-gravity, latency, and regulatory mandates. This is particularly true in financial services, insurance, and healthcare.
- Private-cloud is different than legacy on-prem. Private-cloud environments deliver cloud-like APIs and self-service portals behind the firewall. Our interpretation is that “on-prem” in this survey refers to classic siloed estates lacking unified storage/compute/network control planes. Scale, not geography, is the distinguishing factor.
- Dell and HPE lead the on-prem race. When respondents were asked which vendors they would choose for in-house AI stacks, Dell Technologies and Hewlett Packard Enterprise topped the list, confirming their early traction with “AI factory” bundles.
- GPU-aaS is a sleeper segment. Only ~2 percent of workloads land on GPU utility clouds today, yet interest is climbing; Lambda Labs and CoreWeave out-poll several Tier-2 hyperscalers for burst capacity.
- Edge is nascent but inevitable. Retail point-of-sale, robotic inspection, and autonomous-vehicle inference account for a small slice today, but we believe edge share will double once AI models shrink and 5G private wireless densifies. Notably, cloud players (e.g. Amazon Lambda, Azure Functions) are prominently cited by respondents.
In our opinion, the chart crystallizes the emerging “bring AI to the data” narrative. Public-cloud agility remains unmatched for rapid prototyping, yet sovereignty-minded sectors refuse to copy/move exabytes of sensitive data into someone else’s region. Vendors that can deliver a cloud-like experiences everywhere (supercloud) – e.g. public region, colocation cage, data-center row, or factory floor – will capture the next tranche of enterprise spend. The race is on to make on-prem AI as turnkey as SaaS while preserving the governance guarantees boards now demand.
The AI Data-Center Super-Cycle Is Real: Cloud Leads, but On-Prem Will Top $100 B by 2030
We believe the data below confirms that generative AI has ignited the largest single-purpose infrastructure boom since the client-server era. The stacked area forecast—split into dark-blue “AI in the cloud” and light-blue “AI on-prem in the enterprise”—shows aggregate AI-only data-center spend (compute, storage, networking, power, and cooling) vaulting from roughly $43 B in 2023 to $180 B in 2024, surpassing $250 B in 2025 and kicking off what we’ve called a data center super-cycle.
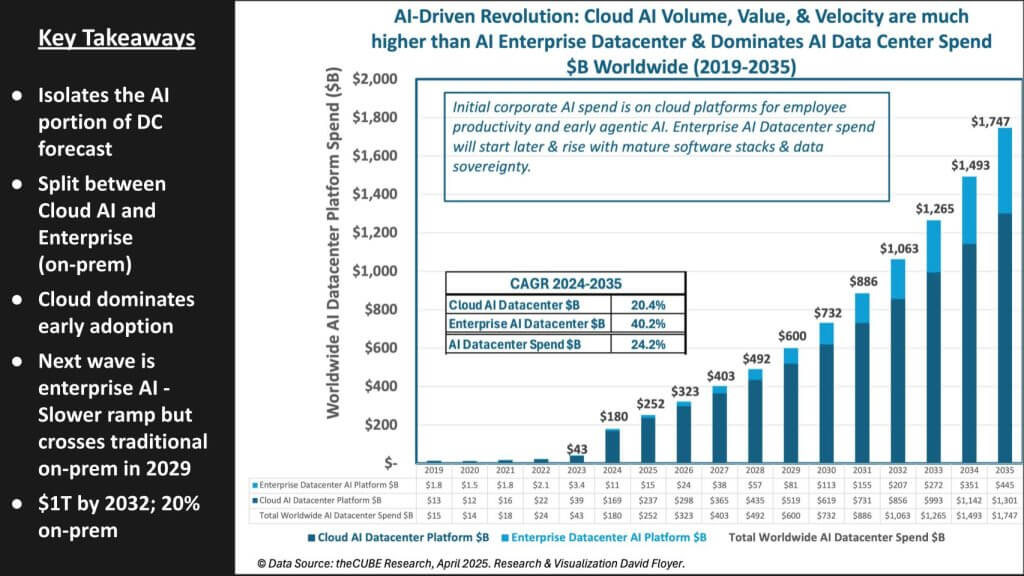
Our model projects a 24 % 10-year CAGR for AI data center buildouts through 2034, but an even faster 40 % CAGR for enterprise on-prem deployments. By 2030, we estimate on-prem AI data center infrastructure will eclipse $100 B, overtaking today’s entire footprint of general-purpose data-center gear and carving out a market that rivals early hyperscale spend profiles. Importantly, this view excludes SaaS consumption, underscoring just how massive the physical-infrastructure TAM is before a single subscription dollar is counted.
Key Findings
Observation | Implication |
---|---|
$180 B step-function in 2024 was propelled by NVIDIA-driven GPU clusters, ancillary power/cooling, networking and storage. | Our research indicates extreme-parallel processing (EPP) shifted from “tens of billions” to “hundreds” virtually overnight, validating the super-cycle narrative. |
Cloud remains dominant today, but the slope of enterprise spend is steeper. | Data-gravity, latency, and sovereignty are forcing banks, insurers, and manufacturers to replicate AI factories behind their own firewalls. Look to Dell, HPE, and Supermicro as major suppliers of gear. |
Build and buy coexist—for now. | Enterprises are simultaneously licensing agentic SaaS (e.g., Salesforce Agentforce, ServiceNow Now Assist) while building bespoke models; both flows ultimately land on hyperscaler infrastructure and inflate the dark-blue area. |
Decision point looms. | ETR notes CFOs will eventually choose between perpetual SaaS fees and self-operated stacks once models stabilize and ROI crystallizes. |
GPU-aaS and colocation emerge as swing factors. | CoreWeave-style utilities enable burst capacity without full capex, blurring lines between public cloud and private DC economics. |
Robotics wave is still off-chart. | Jensen Huang’s third vector – AI in physical robots and far edge – lies beyond this forecast model, implying additional upside not yet modeled. |
In our view, the chart’s widening light-blue band crystallizes the “bring AI to the data” thesis. On-prem estates may have ceded generic workloads to hyperscalers, but they will reclaim budget as organizations embed AI next to proprietary datasets that can’t, or won’t, move. Vendors that deliver turnkey AI factories with cloud-like control planes, rich data-stack partnerships, and ecosystem breadth will be positioned to capture the next $100 B wave.
How do you see it? Where are you putting your AI bets? Let us know and thanks for reading.