Recent announcements from the AWS Summit New York signal a significant leap forward in the democratization and practical application of generative AI technologies. I’ve covered some of the news coming out of this analyst forum event previously, but couldn’t wrap up my coverage without diving into Bedrock. AWS has clearly positioned Amazon Bedrock as a cornerstone of the company’s AI strategy, with the service experiencing rapid adoption and becoming one of AWS’s fastest-growing offerings in the past decade. Amazon Q is an equally important part of AWS’s generative AI stack and announcements around enhancements to Q as well as general availability of Amazon Q Apps, a feature of Amazon Q Business, were also key highlights. Amazon’s three-tiered Gen AI stack has been purpose built to deliver for customers wherever they happen to be on their generative AI journeys.
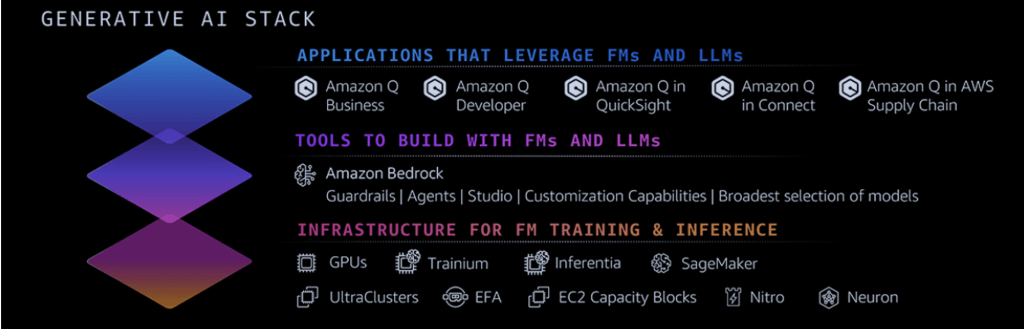
Image credit: AWS
Enhancements to Amazon Bedrock
The enhancements to Amazon Bedrock, which I call the “toolset layer,” are all about providing tools to build, scale, and deploy gen AI apps that leverage LLMs and other foundation models, are particularly noteworthy. AWS is addressing key challenges in the enterprise AI space, namely customization, performance, and responsible use.
By introducing fine-tuning capabilities for Anthropic’s Claude 3 Haiku model, AWS is enabling businesses to tailor large language models (LLMs) to their specific needs and industries by fine-tuning Anthropic Claude 3 Haiku on proprietary datasets. This level of customization is crucial for organizations looking to derive maximum value from AI with regard to specific tasks or domains, using an organization’s own proprietary data.
Fine-tuning is an important part of model performance, as part of the NLP process of customizing a model for a specific task. This allows for the adaptation of knowledge within models, allowing for customized use cases that have task-specific performance metrics. Fine-tuning Anthropic’s Claude 3 Haiku model is significant as it will not only boost model accuracy and quality, but also provide better performance. Equally important, this will deliver on the reduced costs and latency fronts. It’s likely you’ve seen feedback in social channels in the past couple of weeks expressing excitement about this — that’s because it is very, very big news.
An expansion of Retrieval Augmented Generation (RAG) capabilities is another significant step. By adding connectors for popular enterprise platforms like Salesforce, Confluence, and SharePoint, AWS is making it easier for companies to leverage their existing data repositories to enhance AI model outputs. This integration of proprietary data with powerful LLMs has the potential to create highly contextualized and relevant AI applications across various business functions.
Vector search improvements across AWS’s data services portfolio, particularly the general availability of vector search for Amazon MemoryDB, underscore the company’s commitment to performance. The ability to achieve single-digit millisecond latency for vector searches is a game-changer for real-time AI applications, opening up new possibilities in areas like semantic search and personalized recommendations.
The enhancements to Agents for Amazon Bedrock are particularly intriguing from my perspective. The addition of memory retention across multiple interactions and the ability to interpret code for complex data-driven tasks significantly expands the potential use cases for AI agents. This moves us closer to truly intelligent assistants capable of handling sophisticated, multi-step processes that integrate seamlessly with existing business systems.
AWS’s focus on responsible AI use is evident in the company’s introduction of contextual grounding checks in Guardrails for Amazon Bedrock. The ability to detect and filter out hallucinated responses in RAG and summarization workloads addresses one of the most pressing concerns in generative AI adoption. By claiming to reduce hallucinations by over 75%, AWS is taking a significant step towards making AI more trustworthy and reliable for enterprise use
Amazon Q: Meeting Customers Where They Are on Their AI Journeys
Amazon Q, Amazon’s generative AI-powered assistant is at the top layer of the AWS tech stack. AWS has officially launched Amazon Q Apps, moving it from public preview to general availability. This new capability, part of the Amazon Q Business suite, allows users to rapidly develop applications directly from their conversations with Amazon Q Business or by describing their desired app in natural language.
Some of the key features of Amazon Q Apps include:
Streamlined app creation. Users can transform ideas into functional apps in a single step, enhancing productivity for individuals and teams.
Sharing and customization made easy. Apps can be easily shared and tailored to specific needs, with the option to publish to an admin-managed library for wider organizational access.
Robust security. The robust security built into Amazon Q Apps might be one of my favorite features. Amazon Q Apps inherits user permissions, access controls, and enterprise guardrails from Amazon Q Business, ensuring secure sharing and compliance with data governance policies.
API integration. New APIs enable the integration of Amazon Q Apps into existing tools and application environments, allowing for seamless creation and consumption of app outputs.
Iterative development. App creators should be excited by the fact that they can now review and refine their original creation prompts, facilitating continuous improvement without starting from scratch.
Data source selection. Users can choose specific data sources to enhance the quality of app outputs.
Amazon Q Business and Amazon Q Apps are now available in two AWS Regions: US East (N. Virginia) and US West (Oregon).
This release is exciting: it marks a significant step in AWS’s efforts to democratize app development and enhance business user experience through AI-powered tools.
Wrapping Up: Good Things Ahead for Partners and Customers on the AI Front
The partnership ecosystem AWS is building around its AI services is impressive and bodes well, both for AWS partners and customers. The expansion of the AWS Generative AI Competency Partner Program and the new collaboration with Scale AI demonstrate AWS’s understanding that successful AI implementation often requires specialized expertise and tools. This ecosystem approach should help accelerate AI adoption across various industries and geographies.
From a market perspective, these announcements position AWS strongly in the competitive cloud AI landscape. By offering a comprehensive suite of AI services that address customization, performance, and responsible use, AWS is catering to the needs of enterprises that are looking to move beyond experimentation and into production-scale AI deployments.
The case studies shared, such as Ferrari’s use of Amazon Bedrock for various applications and Deloitte’s development of the C-Suite AI™ solution for CFOs, illustrate the real-world impact of these technologies. These examples demonstrate how generative AI can be applied to create tangible business value across different sectors and functions.
However, it’s important to note that while AWS has made significant strides, the AI landscape is rapidly evolving. Competitors like Google Cloud and Microsoft Azure are also innovating in this space, and it will be crucial for AWS to maintain its momentum and continue to address emerging challenges in AI adoption.
One area that warrants further attention is the long-term implications of model fine-tuning and customization. As more organizations create bespoke versions of LLMs, questions around model governance, version control, and the potential for bias amplification will need to be addressed.
AWS’s latest announcements represent a maturation of the company’s AI offerings. By focusing on customization, performance, and responsible use, it’s clear that AWS is positioning itself as a leader in enterprise AI solutions. I’ll be watching closely to see how these new capabilities translate into real-world implementations and whether they can truly accelerate the adoption of generative AI in the enterprise space. The coming months will be crucial in determining whether AWS can maintain its edge in this highly competitive and rapidly evolving space.
See more of my AWS coverage from this event here:
Deloitte AWS Collaboration Designed to Accelerate and Scale Enterprise AI Adoption