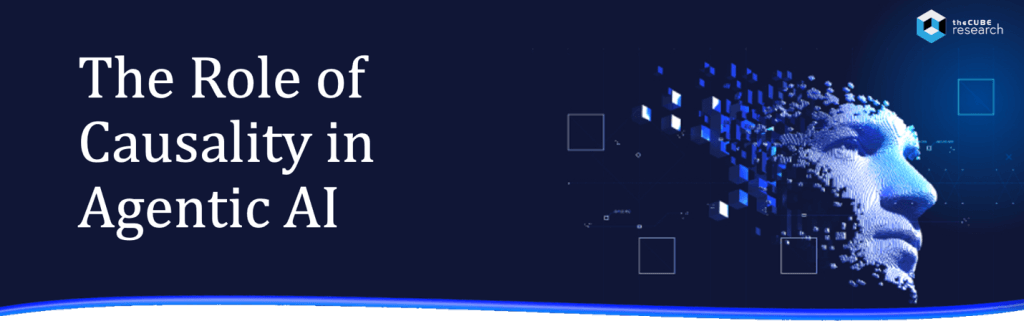
There is a missing ingredient in today’s AI, which, when added, will make AI a truly indispensable partner in business and scale ROI over the long run.
This ingredient is also fundamental to creating agentic AI systems, where networks of agents help humans make decisions, solve problems, and even act on their behalf.
The missing ingredient is CAUSALITY and the science of WHY things happen.
Here at theCUBE Research, we are focused on informing you about the latest developments shaping AI’s future and helping you prepare now rather than later.
This research note is the third in a series on the emerging impact of Causal AI. It will explore how the new capabilities of causal AI will shape the future of agentic AI systems.
- The need for causality
- Causality in agentic systems
- Causal reasoning concepts
- What to do and when
In future research notes, we’ll delve deeper into Causal AI use cases, technology toolkits, and the vendor community that is democratizing them for the masses.
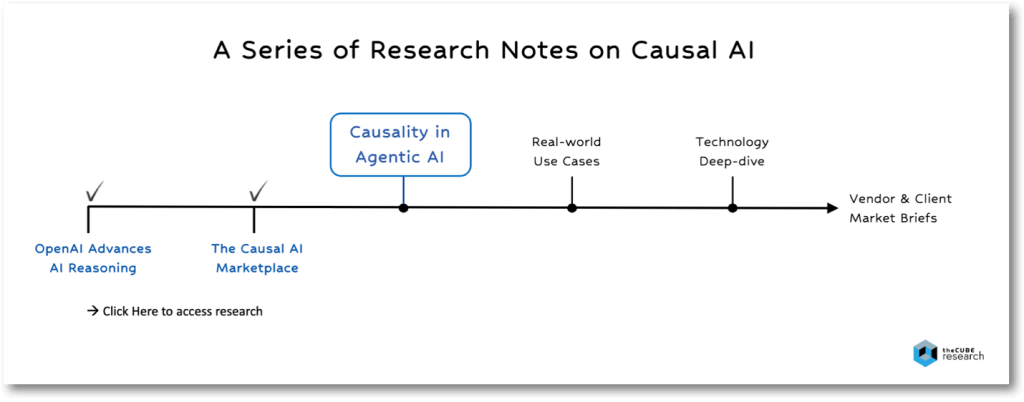
Analyst Angle
Click Here to Watch the Video Analyst Angle Podcast.
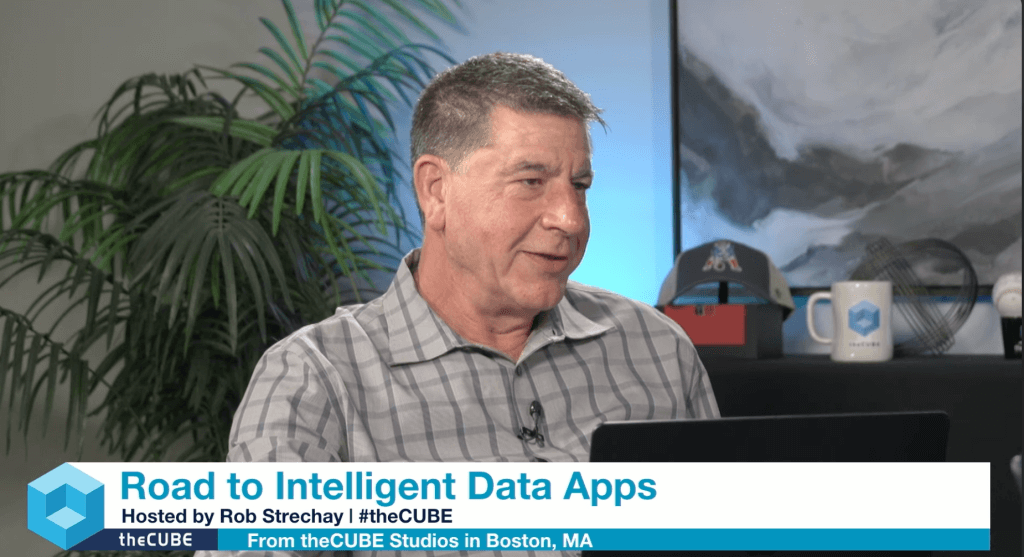
The Need For Causality
The AI journey in business started with organizations applying predictive AI models to analyze large datasets, forecast outcomes, identify patterns, and isolate anomalies across a broad range of use cases. Today, according to McKinsey & Company, over 70% of global enterprises have deployed at least some AI capabilities.
The advent of generative AI and Large Language Models (LLMs) just a few years ago sparked a rapid acceleration of AI adoption among most enterprises. New Enterprise Technology Research (ETR) survey data from 1550 decision-makers indicates that this acceleration will continue in the years ahead. AI represents the highest spending trajectory among all technology categories with a net score of 57, nearly double all other categories.
In addition, in a recent Breaking Analysis: Gen AI Adoption Sets The Table for AI ROI podcast hosted by Dave Vellante, he reported fresh ETR data showing that 9 of 10 enterprises have already achieved ROI from their generative AI investments, with 56% having even higher ROI expectations over the next year. It also showed that generative AI use cases have been predominantly focused on creating predictions, detecting patterns and/or anomalies, analyzing information, and enhancing task-level productivity.
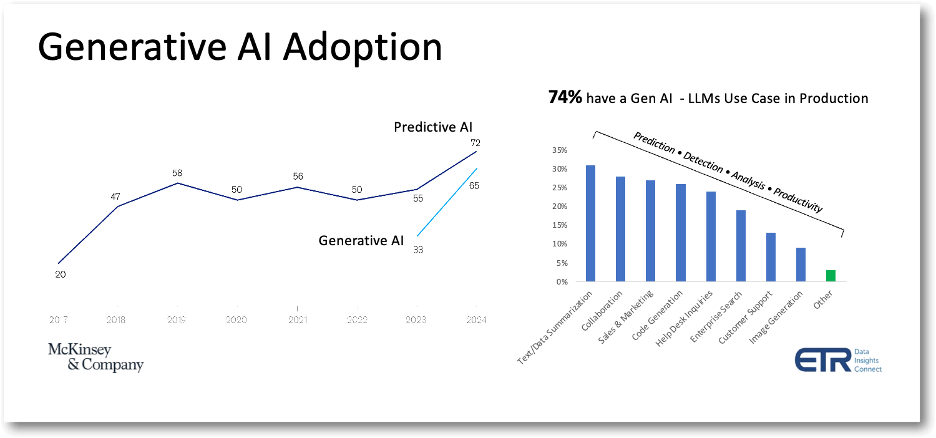
However, most businesses now envision more transformative, higher-ROI use cases that automate workflows and enhance the productivity of their workers and customers.
To do so, more and more businesses are designing new Agentic AI systems that unleash networks of AI agents that understand their business dynamics and improve organizational workflows, decision-making, and problem-solving. In many cases, these agents can even act autonomously when human intervention is not feasible or warranted.
Others plan to use AI agents to improve their talent and enable workers to take on new roles. For example, enabling a business analyst to become a data scientist or a front-line customer service representative to diagnose and solve technical problems on the spot with customers without having to pass them on to level 2 specialists.
Most plan to augment their customer-facing experiences with AI agents who, unlike today’s chatbots and AI assistants, are empowered to act quickly on customers’ behalf or better explain their options.
Given its highly intriguing promise of value, it’s likely that 2025 will become the year of agentic AI as the demand for such solutions is already strong. According to a Capgemini survey of 1,100 executives at large enterprises, 82% plan to deploy AI agents within the next three years, growing from 10% today.
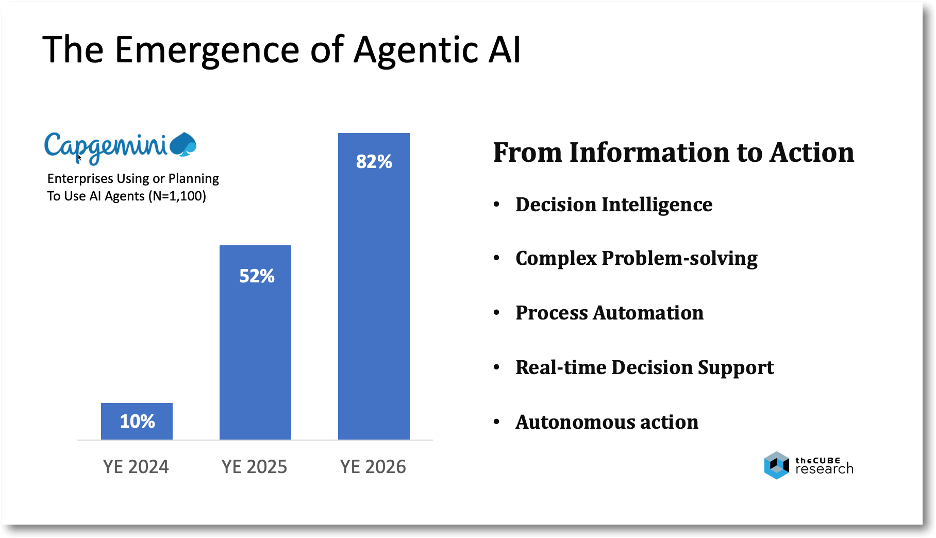
The trend toward Agentic AI does beg the question — what is the difference between AI assistants (or chatbots), AI agents, and Agentic AI?
After all, it’s widely estimated that over 70% of global businesses have already deployed business-unique AI assistants that are responding to billions of annual inquiries.
The general consensus is that they differ based on their use case maturity:
- AI assistants (or chatbots) assist users in executing tasks, retrieving information, or creating some type of content. They are dependent on user input and act on explicit commands. Generally speaking, they are limited to predefined inputs and outputs (with some personalization) and respond based on learnings from past datasets.
- AI agents assist users in achieving goals by interacting within a broader environment of considerations. They sense, learn, and adapt based on human and algorithmic feedback loops. Since they operate more autonomously, they can help people make better decisions and automate workflows without direct guidance on how to do so. They’ll also be better equipped to handle real-time conditions.
- Agentic AI systems assist users and organizations in achieving goals that are too sophisticated for a single agent to handle. They do so by enabling multiple agents with their own sets of goals, behaviors, policies, and knowledge to interact. Think of the “wisdom of crowds,” where agents share knowledge, negotiate, cooperate, coordinate, or even compete to optimize outcomes based on their own datasets.
The differences represent an evolution from achieving tasks to achieving goals, whether those goals are individual, organizational, or both, with increasing levels of task automation, decision intelligence, and problem-solving capabilities.
And doing so by evolving from processing information to taking controlled, consequence-aware actions with the highest degree of quality, transparency, and consistency.
In addition, given the objective of AI agents to partner with humans to accomplish goals, the agents will need to rely on more sophisticated and natural ways to collaborate with their human counterparts. This may involve combining enterprise knowledge with the knowledge extracted from humans during conversations to understand their intentions, emotions, gestures, and personas while being able to explain the steps they took or will take. We believe that multimodal neuro-symbolic AI conversational capabilities, such as those from Openstream.ai, will become an important part of AI agent designs.
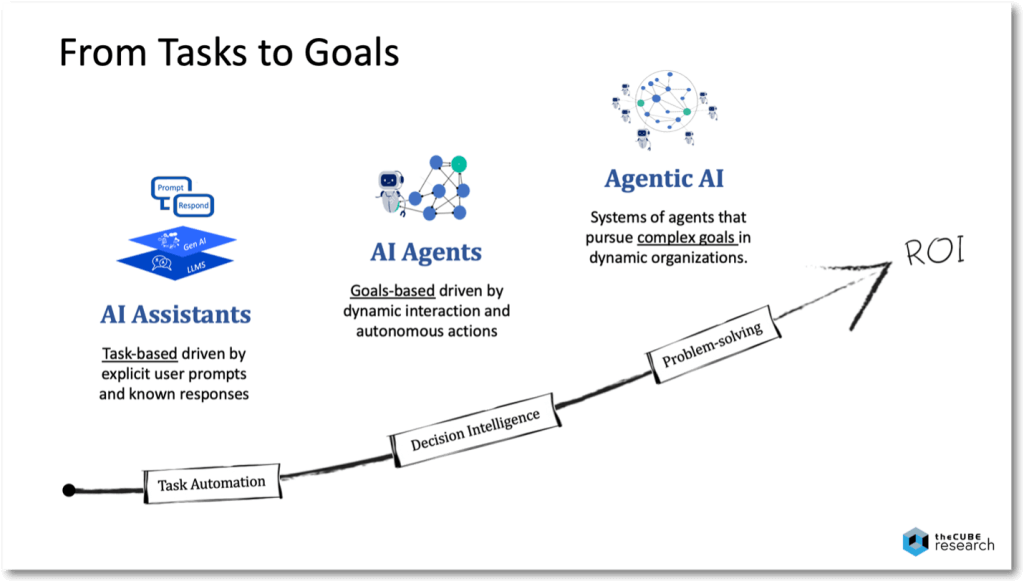
As the Capgemini survey revealed, businesses are starting their agentic AI journeys to increase workflow productivity (71%), improve decision-making (64%), and help organizations solve more complex problems (64%). These objectives generally involve identifying, analyzing, and finding solutions to overcome challenges or obstacles to achieve a desired goal. This requires the agent to reason through a series of steps to understand the nature of the issue, current conditions, possible approaches, consequences of alternate actions, and how to construct an effective solution.
However, as Gartner Group points out in its agentic AI research, a big gap exists between current generative AI and LLM-based assistants and the promise of AI agents. Today’s AI struggles to understand complex goals, adapt to changing conditions, proactively plan, and act more autonomously. We agree that the promise of agentic AI resides in enabling a new generation of agents that can:
- Adapt to change
- Proactively plan
- Understand complex goals
- Operate in complex environments
- Act autonomously when needed
The bottom line is that today’s AI capabilities (predictive AI, Gen AI, and LLMs) will enter a phase of diminishing returns for business unless the missing ingredients are added to the mix of AI model architectures to close this gap. Its the key to evolving from static-state AI to dynamic AI.
Unless, of course, you believe that your future will be just like your past.
But that is not a reality since businesses operate in a dynamic world with a never-ending desire to continuously improve and shape future outcomes.
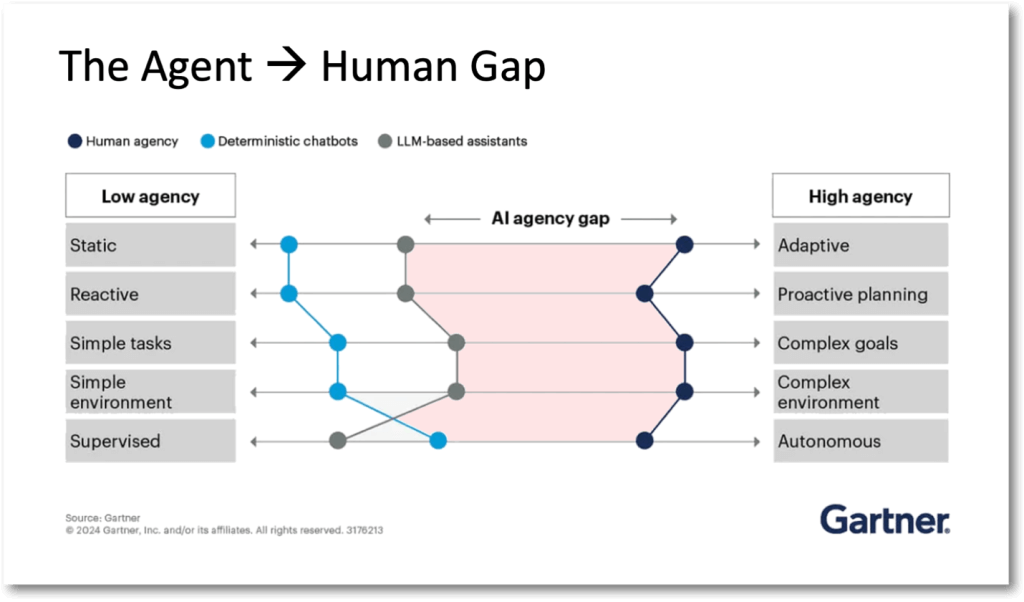
Causality in Agentic Systems
One of the most critical missing ingredients in closing the “AI agency gap” is causality and the science of why things happen. We believe AI systems will rapidly mature in the years ahead by infusing progressive degrees of causal reasoning capabilities. These systems will be much better equipped to deliver on the ROI promise of Agentic AI.
Causality will enable businesses to do more than create predictions, generate content, identify patterns, and isolate anomalies. They will be able to play out countless scenarios to understand the consequences of various actions, explain their business’s causal drivers, make better decisions, and analytically problem-solve. AI agents will be better equipped to comprehend ambiguous goals and take action to accomplish those goals.
In essence, they’ll be help people know WHAT to do, HOW to do it, and WHY certain actions are better than others to shape future outcomes more prescriptively.
An array of emerging causal AI methods and tools are now supplying data scientists with new “ingredients” for their AI recipe books, empowering them to infuse an understanding of cause-and-effect into their AI systems. The new ingredients include:
- Interventions: determine the consequences of alternate actions
- Counterfactuals: evaluate alternatives to the current factual state
- Root Causes: detect and rank causal drivers of an outcome
- Confounders: identify irrelevant, misleading, or hidden influences
- Intuition: capture expertise, knowledge, tacit know-how, known conditions
- Pathways: understand interrelated actions to achieve outcomes
- Rationalizations: explain why and how certain actions are better than others
Collectively, these new “ingredients” promise to enable AI systems to progressively deliver higher degrees of causal reasoning to close the gap in today’s LLM limitations and future-state agentic systems. And, in turn, better understand the dynamics of how a business operates, creates value, and engages the world to help humans make better decisions.
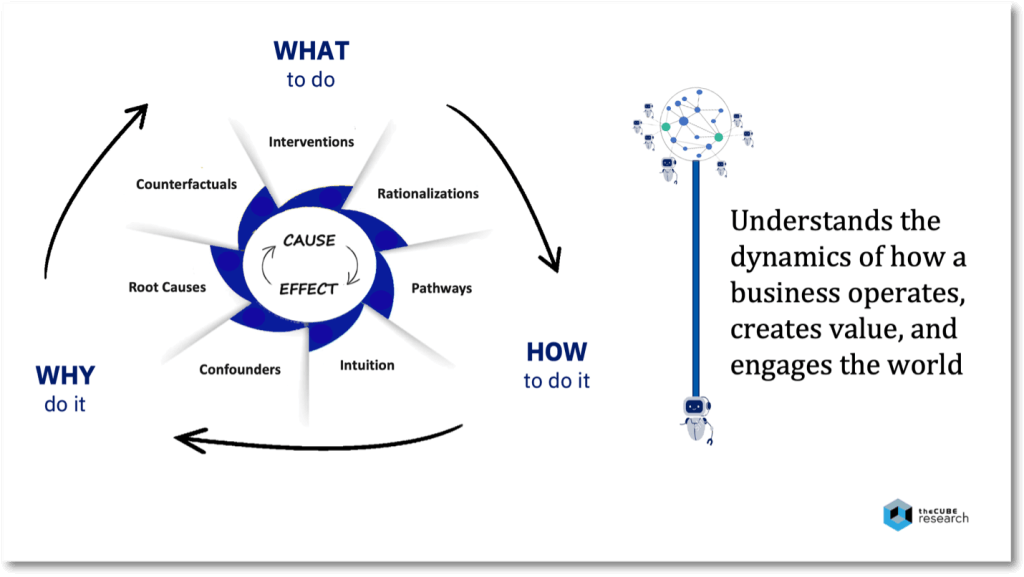
The view of theCUBE Research and an array of Agentic AI pioneers we have engaged is that causal reasoning will become an integral component of future-state agentic systems. These systems will orchestrate an ecosystem of collaborating AI agents and a mix of predictive, generative, and casual models to help people problem-solve and make better decisions. They will also continuously teach each other to improve outcomes learned from real-world, outcome-based experiences.
The architectures of these systems will be composed of:
- Data & AI Platforms that provide the foundational data fabric and the capabilities to build, integrate, manage, and govern AI models and agentic systems.
- LLMs (large language models) that deliver enterprise-wide intelligence and generally applicable Gen AI services customized from an ecosystem of models.
- SLMs (small language models) that deliver domain-specific knowledge and an understanding of relationships among entities.
- CCMs (causal component models) that incapsulate cause-and-effect mechanisms within a system and how causal effects in one part of the system affect others.
- SAMs (small action models) that deliver decision intelligence to AI agents and empower autonomous actions aligned to a goal, plan, or problem set.
- AAOLs (Agentic AI Orchestration Layers) that coordinate and govern interaction among agents and the gathering of intelligence from within the model ecosystem.
- Agents that enhance or automate certain aspects of the human or organizations effort to achieve a goal, plan, solve a problem, or perform a task.
The heart of causal reasoning within the system is delivered by the causal component models, which can be flexibly integrated to achieve the objectives of specific use cases (think, model micro-services). Causal component models divide a system into distinct, manageable components representing specific causal relationships or dependencies. These components are then interconnected to form a complete causal model of a system, allowing them to examine how different causal relationships combine to produce overall system behavior.
Furthermore, by integrating Multi-Agent Reinforcement Learning (MARL) and/or self-taught reasoning (e.g., STaR) methods into the system of interconnected models and agents, they collectively learn based on each other’s actions, knowledge, and rewards through a process of managed transfer learning. Collectively, they create a self-educating loop that continuously refines the system’s overall accuracy and productivity.
Suppose the objective of agentic AI is to help humans (and organizations) achieve goals. In that case, this is not truly possible without the ability to reason, just as it is not true for humans. Thus, our view is that differentiation and ROI expansion will grow as a function of the degree of causality infused into Agentic AI systems.
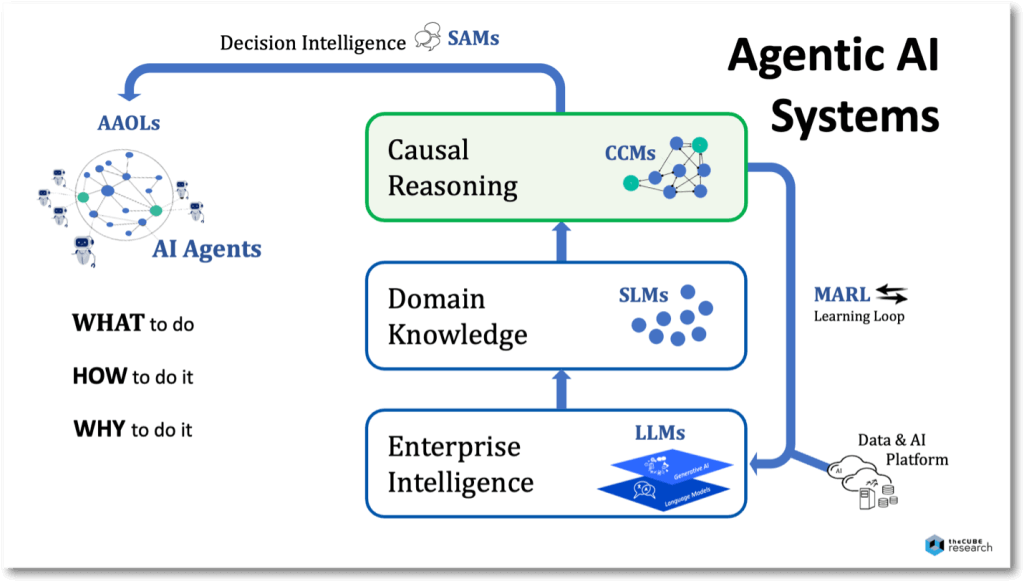
Causal Reasoning Concepts
People, businesses, and markets rely heavily on causality to function. It allows us to explain events, make decisions, plan, problem-solve, adapt to change, and guide our actions based on consequences.
Given that humans are causal by nature, AI must also become causal by nature. And, in turn, create a truly collaborative experience between humans and machines.
In other words, without casual reasoning, AI will lack the ability to mimic how people think or how the world works, as nothing can happen or exist without its causes.
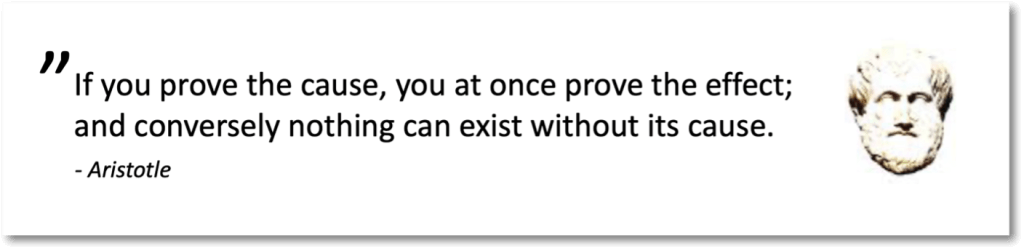
The method upon which AI helps people reason is its inferencing design — that is, how it progresses from a premise to logical consequences to judgments that are considered true based on other judgments known to be true. In essence, it must determine WHAT will happen, HOW it will happen, and WHY it knows certain actions are better than others. This level of intelligence can only occur by fusing AI’s correlative powers with causality.
Applying Aristotle’s proclamation to the business world, every outcome has causes, and no outcome can exist without its causes. Thus, understanding cause and effect is how we understand the dynamics of how a business operates, creates value, and engages the world. In turn, this better equips us to shape future outcomes.
More specifically, it will help businesses better know:
- WHAT to do — Today’s GenAI and LLM designs correlate variables across datasets, telling us how much one changes when others change. They essentially swim in massive lakes of data to identify patterns, associations, and anomalies that are then statistically processed to predict an outcome or generate content. This design, however, is prone to hallucinations, bias, and the concealment of influential factors. LLMs operate similarly to the limbic brain, which drives instinctive actions based on memories, which is what makes them good at determining the “what,” automating tasks, and creating content.
- HOW to do it — Beyond predicting or generating an outcome (the “what”), businesses also want to understand how to accomplish a goal or how an outcome was produced. This requires advanced transformations integrating correlative patterns, influential factors, neural paths, and causal mechanisms to de-code the “HOW.” Have you ever tried to explain something without invoking cause & effect? Think of causal AI techniques as simulating the cerebral cortex that’s responsible for encoding explicit memories into skills and tacit know-how. This is key to recommending prescriptive action paths that are trusted, transparent, and explainable.
- WHY do it— Businesses will also want AI to help them evaluate the consequences of the various actions they could take to improve outcomes. That is, why is one set of actions better than another? For AI to truly help humans reason and problem-solve, it must algorithmically understand precise cause-and-effect relationships. That is, to understand the dynamics of why things happen so that people can explore various “what-if” propositions. This mimics the neocortex, which drives higher-order reasoning, such as decision-making, planning, and perception. These powers are necessary for AI to collaborate with humans to solve problems
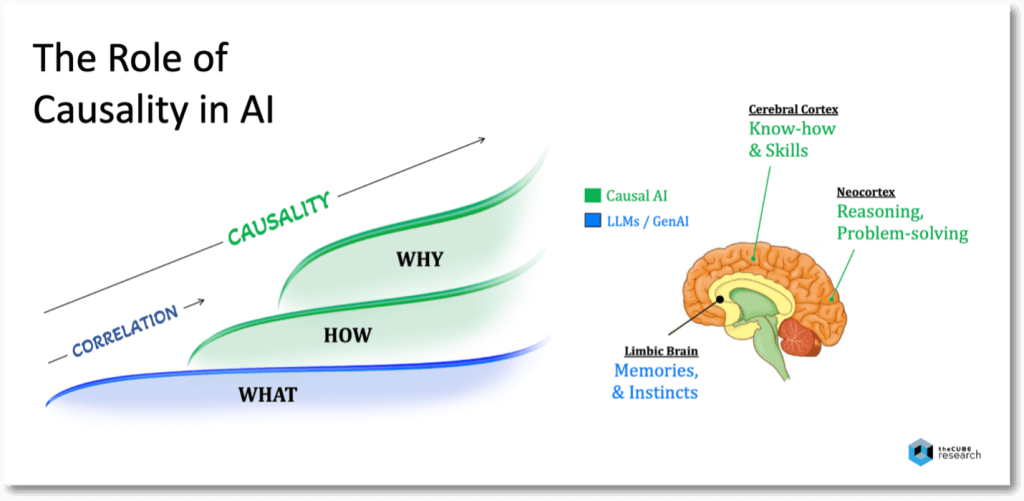
From a technical perspective, today’s predictive AI models operate on brute force, processing immense amounts of data through numerous layers of parameters and transformations. This involves decoding complex sequences to determine the next best token until it reaches a final outcome. This is why you often see the words “billions” and “trillions” associated with the number of parameters in large language models (LLMs).
But no matter how big or sophisticated today’s LLMs and predictive AI models are, they still establish only statistical correlations (probabilities) between behaviors or events and an outcome. However, that is very different from saying that the outcome happened because of the behaviors or events. Correlation doesn’t imply causation. There can be correlation but not causation. And while causation implies correlation, its influence may be so minor that it’s irrelevant. Equating them risks creating an incubator for hallucinations, bias, and drawing incorrect conclusions based on incorrect assumptions.
In addition, correlation-driven AI systems generally operate in mostly static environments because they rely on statistical correlations and patterns derived from historical data. They often assume that the relationships within the observed patterns will remain stable over time. For AI systems to adapt and respond to changing conditions, they must understand the underlying causes driving those relationships. By augmenting correlative AI with the mechanisms of causality, AI systems will be better able to understand the dynamic behaviors of their businesses when conditions and interactions change over time.
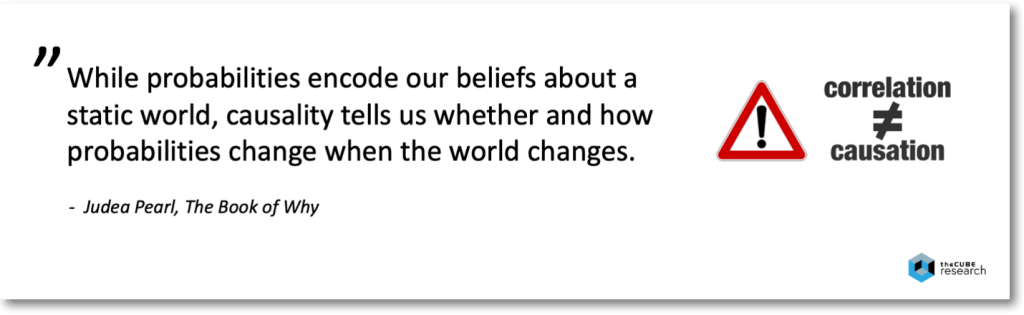
This challenge becomes even more apparent when LLMs are presented with more ambiguous, goal-oriented prompts, which are at the heart of Agentic AI’s promise of value.
A recent study by Apple explored the limitations of LLMs to reason, finding that all state-of-the-art LLMs experienced significant accuracy declines when promoted with:
- different versions of the same problem
- altered values in a problem
- complex problems involving multiple clauses
- seemingly relevant but ultimately irrelevant information
These limitations resulted in up to a 65% decline in accuracy due to difficulties in discerning pertinent information and processing problems. The findings illustrate the risks of relying on an LLM for problem-solving as it is incapable of genuine logical reasoning but instead attempts to replicate the reasoning steps observed in its training data.
For enterprises looking to deploy AI agents, LLMs must be complemented with new technologies that support the fundamental pillar of comprehension, reasoning, and problem-solving, namely the ability to understand cause and effect.
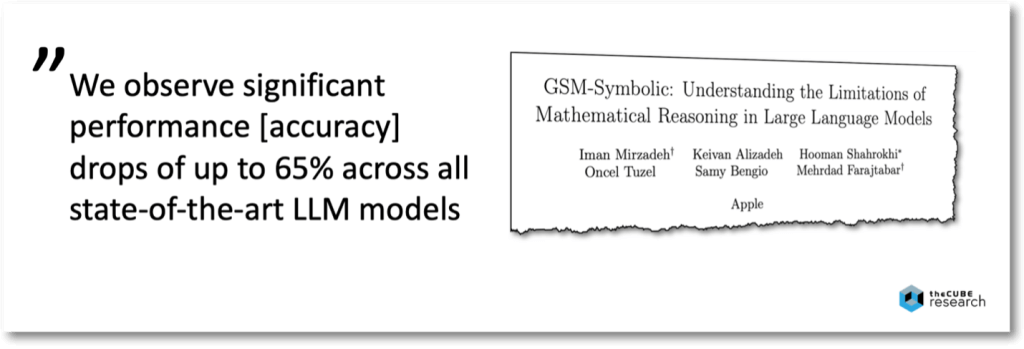
Furthermore, the pathways through today’s LLMs and neural networks are black boxes. They cannot tell you how variables interacted, their values, or how they influenced an outcome. This significantly limits their ability to explain its outcomes. As a result, they cannot truly understand the dynamic nature of how a business operates, creates value, and engages the world.
Causal AI methods help uncover and infer causal pathways within neural networks by combining traditional neural network architectures with causal inference techniques. This integration enables neural networks to identify correlations AND model cause-and-effect relationships to understand relationships among variables in a dataset and how much those variables influence each other. This allows progressive discovery of complex causal relationships that are ranked (scored) in terms of influence, enhancing the decision-making process for users. And, in turn, recommend more trustworthy actions.
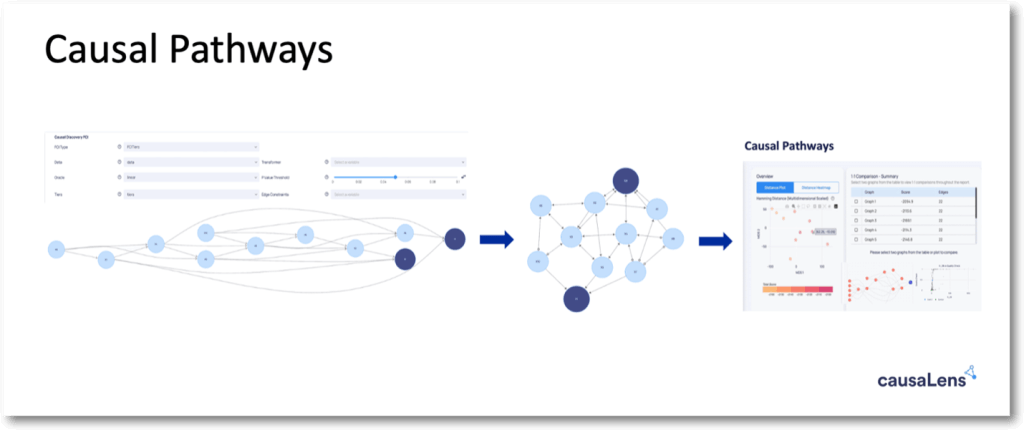
To summarize, we define Causal AI as a branch of machine learning that emphasizes understanding cause-and-effect relationships rather than solely processing patterns or semantical relations in data. It enables data scientists and AI engineers to infuse expanding degrees of causal reasoning into AI systems, building upon the semantic reasoning that knowledge graphs provide.
While LLMs typically forecast or predict potential outcomes based on historical data, Causal AI goes a step further by elucidating why something happens and how various factors influence each other. In addition, Causal AI can understand statistical probabilities and how those probabilities change when the world around them changes, whether through intervention, creativity, or evolving conditions. This will enable businesses to explore countless scenarios while confidently understanding how actions impact outcomes.
This is crucial because, without causality, AI agents will only perform well if your future resembles the past, which is not the reality of inherently dynamic business. After all, businesses rely on people to make decisions and act, and those people work within dynamic environments.
This is why, in part, the 2024 Gartner AI Hype Cycle predicted that Causal AI would become a “high impact” technology in the 2-5 year timeframe, stating:
“The next step in AI requires causal AI. A composite AI approach that complements GenAI with Causal AI offers a promising avenue to bring AI to a higher level.”
We couldn’t agree more.
More holistically, we expect agentic AI to become the catalyst for businesses to deploy ecosystems of LLMs, SLMs, Knowledge Models, and Causal Models that architecturally augment each other to create more capable underlying AI systems.
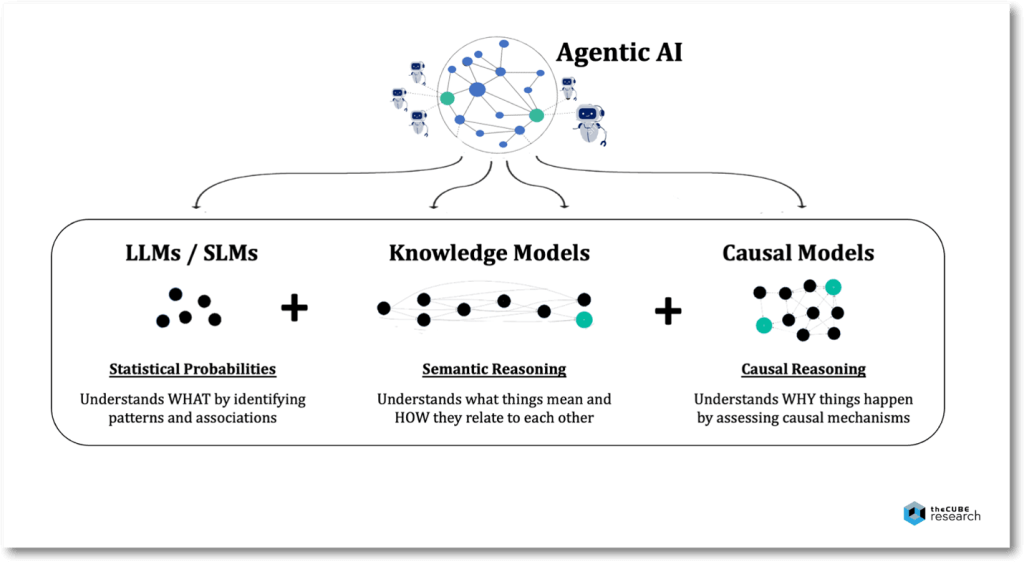
What To Do and When
As the world of Agentic AI matures, causality will only become necessary. Its advent is inevitable, as the limitations of correlative-based AI will eventually hinder innovation.
Perhaps the time is now to start preparing, especially for those who are pursuing the promise of agentic AI. We’d recommend you:
- Build competency in both agentic and causal AI
- Evaluate the impact of causality on your use cases
- Engage with the ecosystem of pioneering vendors
- Experiment with the technology and build skills
We also recommend watching the following podcasts:
From LLMs to SLMs to SAMs: How Agents Are Redefining AI
OpenAI Advances AI Reasoning, But The Journey Has Only Begun
Stay tuned for the next in this series of research notes on the advent of Causal AI, where we will cover an array of real-world use cases and the ROI achievements.
Thanks for reading. Feedback is always appreciated.
As always, contact us if we can help you on this journey by booking a briefing here or messaging me on LinkedIn.
