AWS re:Invent 2024 ushered in a transformative chapter for both Amazon Web Services and the broader tech ecosystem. This year’s event marked the debut of Matt Garman as CEO of AWS, stepping into a role that aligns with what John Furrier aptly describes as a “wartime CEO”—a technically adept leader and trusted consigliere. Garman’s keynote set the tone for AWS’s strategic focus: doubling down on core infrastructure capabilities across silicon, compute, storage, and networking, while extending its Graviton playbook to GPUs and potentially large language models (LLMs).
Key highlights included the unveiling of six new LLMs, formerly codenamed “Olympus,” introduced by none other than Andy Jassy, the godfather of cloud, who made a cameo appearance with a substantive keynote. Jassy’s return underscored Amazon’s commitment to innovation at scale, bridging its heritage in infrastructure with the future of AI.
A notable emphasis on simplification was palpable at this year’s event. While the company remains steadfast in offering granular primitives and service flexibility, it’s now streamlining AI, analytics, and data workflows into a unified view. This vision materialized with advancements in SageMaker, positioning it as a hub for machine learning, analytics and AI. Additionally, the event showcased a deluge of new features and services across AWS’s expansive ecosystem.
In this Breaking Analysis we explore the critical takeaways from re:Invent 2024. Leveraging insights from ETR data, we’ll unpack AWS’s strategy, the implications for the broader ecosystem, and how we believe the next several years will unfold.
Amazon’s Legacy of Primitives not Frameworks is Evolving
One of the recurring themes in AWS’s journey has been its “primitives for builders” ethos—a foundational philosophy shaped by CTO Werner Vogels. At re:Invent 2021, this philosophy came under the spotlight as AWS navigated a market shift from tech-first early adopters to mainstream corporate developers who demand simplicity and pre-integrated solutions.
During the event, Vogels articulated the essence of AWS’s approach: primitives provide unparalleled choice and power, enabling builders to craft virtually anything. However, he also acknowledged a critical trade-off: as primitives proliferate without being inherently designed to fit together, they introduce complexity. This bottom-up approach starkly contrasts with the top-down strategy employed by competitors like Microsoft, which prioritizes simplification and abstracts away the complexity of underlying primitives.
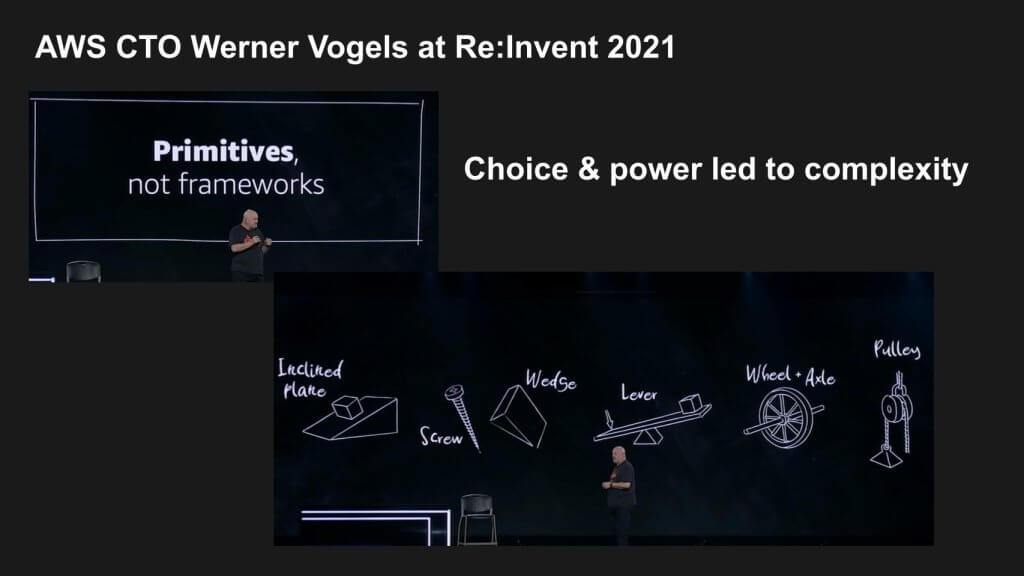
Key Takeaways:
- AWS’s Bottom-Up Philosophy: Vogels emphasized that frameworks often take years to perfect, by which time market dynamics may have shifted. Primitives, in contrast, offer immediate flexibility and enable innovation, but at the cost of added complexity.
- Competing Philosophies: AWS’s reliance on primitives underscores a bottoms-up strategy, which prioritizes developer control and flexibility, deferring simplification. Microsoft’s top-down approach flips this, starting with simplicity and hiding the complexity of primitives behind polished frameworks.
- Evolving Market Demands: The shift from tech-savvy builders to corporate developers requires AWS to balance its traditional ethos with growing demands for streamlined, pre-opinionated solutions. This tension has been a defining narrative in recent years.
Context and Broader Implications:
Selipsky’s tenure at AWS attempted to address this tension by introducing a simplification narrative, beginning at re:Invent 2021. However, these efforts are akin to what Bill Gates said many years ago: When you have an architecture or a business model that you really try and radically shift, it’s like kicking dead whales down the beach.” In other words, it’s a monumental shift that takes time to execute fully. Now, under Matt Garman’s leadership, AWS is positionion to rationalize these seemingly competing philosophies, leveraging its traditional strengths while addressing the complexity concerns of mainstream enterprises.
AWS’s challenge remains clear: integrating its extensive portfolio of primitives into cohesive solutions without sacrificing the flexibility that has long defined its identity. As the market continues to demand simplification, AWS must reconcile these competing forces to maintain its leadership.
Matt Garman at the Helm
AWS, under its new CEO Matt Garman, is reasserting its dominance in core infrastructure while embracing the simplification imperative, for certain parts of its estate. This marks a significant evolution, blending AWS’s traditional ethos of “primitives for builders” with a shift toward pre-integrated, simplified solutions tailored for corporate developers.
One of the standout strategies involves leveraging Nitro and applying the AWS Graviton Playbook—originally devised to lower x86 compute costs—to GPUs and perhaps even LLMs. This year, AWS introduced six new LLM models (previously codenamed Olympus), along with enhancements in SageMaker. These announcements underscore AWS’s commitment to lowering the cost of AI training and inference while reducing its reliance on NVIDIA’s expensive GPUs. With Graviton, Trainium, and Inferentia silicon offerings, AWS is making strides toward a more independent and cost-efficient AI strategy.
This approach has also allowed AWS to get on the Gen AI training and inference learning curve at lower cost, using its own infrastructure (silicon and networking). We’ll come back to this point later in the post but we see this as a significant competitive differentiator.
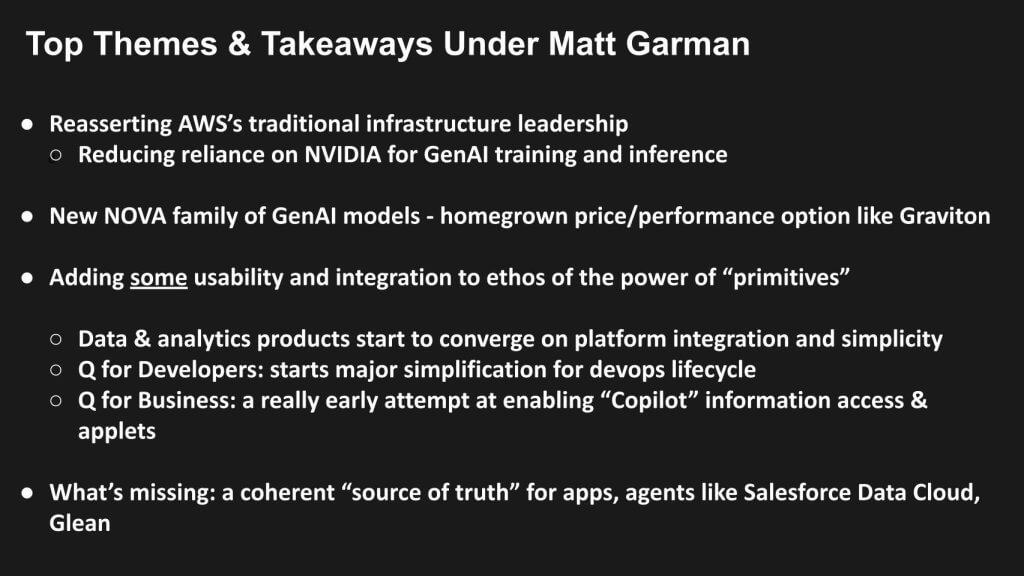
Key Takeaways:
- Simplification vs. Choice: Amazon is trying to do both. By no means is it steering away from its Unix-inspired ethos of providing granular primitives. But it’s adding a more refined focus on simplicity and integration, which resonates with mainstream corporate developers.
- Composable Infrastructure: AWS is able to deliver on a framework for composable infrastructure, breaking free from constraints tied to NVIDIA’s allocation and scarcity challenges. This is explicitly enabled by Nitro and its internal silicon capabilities.
- SageMaker Enhancements: The new SageMaker integrates data, analytics, and AI more cohesively, with improved usability. AWS is converging these areas to better serve developers and enterprises alike.
- Nova Models: Six new homegrown LLMs deliver enhanced price-performance, expanding on AWS’s Graviton-inspired approach to silicon.
- Q for Developers: While Q Business remains in its early stages, Q Developer shows promise in simplifying the software development lifecycle and is more advanced in our view.
- Data Harmonization Gaps: Despite progress, AWS still lacks a unified data source akin to Salesforce’s Data Cloud, leaving room for improvement in establishing a coherent source of truth.
The Broader Perspective:
The irony of this shift is that AWS’s original “primitives-first” ethos left the perception that it was lagging behind in LLM infrastructure and, like others, was forced to rely on NVIDIA. However, for the past two years, AWS has been able to gain critical learnings by evolving its AI infrastructure, utilizing its lower cost chips. Moreover, Garman’s leadership is now reaping the benefits of predecessor Adam Selipsky’s simplification groundwork. AWS’s renewed focus on user-friendly, integrated solutions positions it to compete more effectively in the evolving enterprise market in our view.
As AWS evolves, the simplification and choice positioning will remain central to its strategy. We’ll continue monitoring how this balance shapes AWS’s trajectory in AI, analytics, and infrastructure.
AWS Leverages Silicon and Systems Expertise to Challenge NVIDIA’s Dominance
Matt Garman, AWS’s new CEO, brings a deeply technical background, having led EC2 for years. Garman’s leadership reflects AWS’s strategy of combining technical depth with a pragmatic approach to infrastructure. His tenure marks a shift from Adam Selipsky’s operational focus, positioning AWS to capitalize on its extensive systems expertise. As the industry increasingly relies on AI-driven innovation, AWS is reasserting its dominance in silicon, networking, and infrastructure, aiming to reduce dependency on NVIDIA’s GPUs for AI training and inference.
This strategy stands in contrast to Microsoft’s approach, which has been limited by GPU constraints and a reliance on NVIDIA’s supply chain. AWS’s silicon strategy changed dramatically when it started working with Annapurna Labs and eventually acquired the firm in early 2015. Its silicon roadmap, including Graviton, Trainium, and Inferentia, is focused on lowering costs and optimizing performance for AI workloads. The result? AWS is able to aggressively position itself as a leader in scalable, cost-effective AI infrastructure. Microsoft got started much later in its silicon journey and as such is more exposed to supplies of NVIDIA GPUs.
Google, like Amazon, has been on a custom silicon journey for more than a decade with its Tensor Processing Unit (TPU), first deployed around the 2015 timeframe. Subsequently, Google has rolled out other silicon innovations and has a leading position in the space.
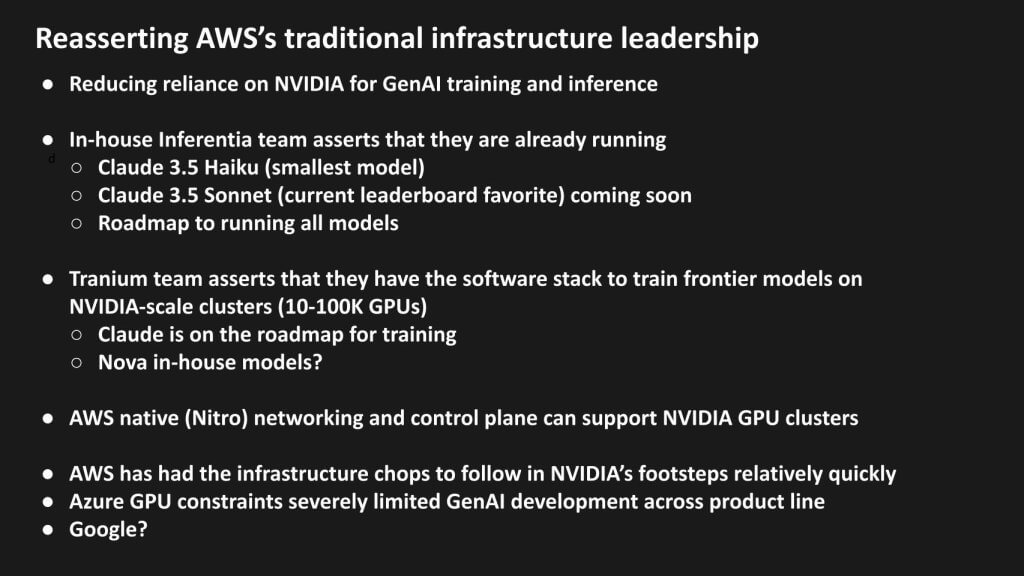
Key Takeaways: AWS, Microsoft, and Google in the Silicon Race
- Reducing NVIDIA Reliance: AWS’s silicon offerings, particularly Inferentia and Trainium, are designed to lower the cost of AI training and inference while reducing reliance on NVIDIA’s GPUs. Notably, AWS has achieved significant milestones in running advanced models like Claude 3.5 on its silicon, a step toward challenging NVIDIA’s inference dominance.
- Microsoft’s GPU Constraints: Microsoft in our view faces certain challenges in the AI space due to its dependence on NVIDIA. Limited GPU access forced Microsoft to prioritize applications like Bing before scaling Copilot functionality across Office and Azure. This sequencing highlights the challenges of scaling GenAI while reliant on external supply chains.
- Google’s Strategic Lead: Google’s early investment in TPUs and high-performance networking allowed it to embed GenAI capabilities throughout its products. Unlike AWS and Microsoft, Google anticipated the infrastructure needs of AI and began building its accelerator and networking capabilities years ahead. This foresight enabled Google to operationalize GenAI ahead of competitors.
AWS’s Competitive Edge: Systems Expertise and Nitro
AWS’s Nitro system is a core differentiator. The company is able to deliver high-performance, Ethernet-based networking infrastructure optimized for AI workloads, avoiding reliance on Nvidia’s InfiniBand infrastructure. Nitro exemplifies AWS’s ability to integrate hardware and software into cohesive systems, and to a certain degree, replicate NVIDIA’s systems-level approach to AI. While NVIDIA continues to lead in training at scale—leveraging software beyond CUDA to optimize massive clusters—AWS is catching up by porting its entire Model Garden to Trainium-based clusters.
The Claude 3.5 Milestone and Anthropic Partnership
Anthropic, with AWS as its primary cloud sponsor, represents a pivotal partnership. While we believe much of the $8 billion investment in Anthropic includes cloud credits, the collaboration showcases AWS’s ability to support advanced models like Claude 3.5 on Inferentia. This capability is a key step toward cracking NVIDIA’s moat around inference, which has historically been a bottleneck for cloud providers. In short, despite some naysayers, we believe the Anthropic investment is working for both companies.
Broader Implications: AI and the Future of Cloud Infrastructure
The GenAI revolution is reshaping every phase of the software development lifecycle. AWS, once perceived as a laggard in AI, now appears well-positioned to compete at scale. By leveraging Nitro and its silicon expertise, AWS is aligning its infrastructure to support GenAI workloads effectively, even as it plays catch-up in areas like large-scale training.
However, AWS’s systems expertise gives it a critical edge. While Microsoft struggles with GPU constraints and Google continues to lead in AI functionality, AWS is building a roadmap to independence. By optimizing its silicon and networking capabilities, AWS is on track to challenge NVIDIA’s dominance and expand its AI offerings within its own ecosystem.
The Road Ahead
AWS’s focus on silicon and systems integration signals a long-term strategy to redefine AI infrastructure. While NVIDIA remains the leader in large-scale training, AWS’s accelerated progress in silicon and networking could erode that dominance, at least within the AWS installed base. With its Model Garden and advanced development tools maturing, AWS is proving that even lower-cost infrastructure can drive meaningful innovation.
Garman’s leadership will be pivotal in navigating this transition. AWS is no longer just following NVIDIA’s footsteps; it is leveraging its systems expertise to chart a path forward that could reshape the competitive landscape of AI infrastructure.
AWS’s Large Language Models – From Alexa to Olympus to Nova
At re:Invent, the introduction of the Nova family of GenAI models marked a significant milestone for AWS, signaling its intention to build leadership in the foundation model space. Andy Jassy’s return to the stage to unveil these models was both a symbolic and strategic move, emphasizing AWS’s commitment to innovation and reinforcing its cultural and technical legacy.
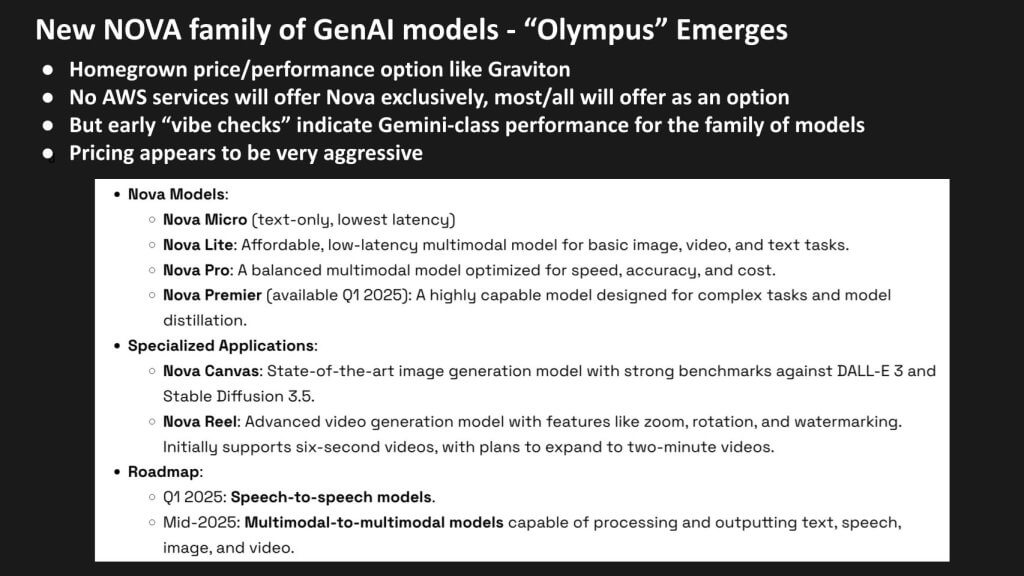
The Nova family, much like Graviton’s positioning against x86 chips, is designed to provide customers with optionality and competitive price-performance. AWS indicated to theCUBE Research that Nova models will not be exclusively tied to any AWS services, maintaining its ethos of flexibility and customer choice. This however could also indicate an internal bias against Nova, which comes out of Amazon.com’s Alexa team. Our understanding is there were at least two competing teams for LLM leadership and it was decided that the Nova team was further along so it won the resource battle.
Nonetheless, the roadmap and benchmarks for these models demonstrate Amazon’s ambition to compete head-on with industry leaders like OpenAI, Google, and Anthropic in the GenAI space, again, providing potentially lower cost solutions for customers.
Key Takeaways: The Nova Family Breakdown
- Diverse Model Offerings:
- Nova Micro: A text-only model designed for lightweight, cost-effective applications.
- Nova Lite: A multimodal model supporting text, image, and video, priced for accessibility.
- Nova Pro: A balanced multimodal model offering advanced capabilities, including a planned upgrade to a 5-million-token context window.
- Nova Premier (2025): A sophisticated model designed for complex tasks like model distillation, launching in Q1 2025.
- Nova Canvas: Focused on image generation, competing strongly against benchmarks like DALL-E and Stable Diffusion.
- Nova Reel: Specializing in video generation, offering advanced features like zoom, 360-degree rotation, and watermarking.
- Roadmap:
- Q1 2025: Introduction of speech models.
- Mid-to-late 2025: Launch of multimodal-to-multimodal models capable of processing and outputting text, speech, image, and video.
- Price-Performance Leadership:
- Initial performance indicators and early demos highlight the competitive pricing of Nova models.
- The planned 5-million-token context window for Nova Pro vastly outpaces competitors like Gemini Ultra, providing greater flexibility for applications requiring extended context.
- Training Infrastructure:
- Pre-training for Nova models was conducted on NVIDIA hardware, but fine-tuning and preference optimization are now performed on Trainium, signaling AWS’s gradual shift away from NVIDIA.
Why Nova Matters for AWS
AWS’s entry into the foundation model game can be considered a strategic necessity. Just as the acquisition of Annapurna Labs laid the groundwork for in-house silicon development (e.g., Nitro, Graviton), the Nova family provides AWS with critical skills in GenAI, ensuring it remains competitive in a rapidly evolving market. This approach also positions AWS to offer differentiated solutions while gradually reducing its dependence on third parties for LLMs and training infrastructure.
Cost-Effectiveness and Technical Implications
The fine-tuning of Nova models on Trainium represents a deliberate effort by AWS to control costs and build independence in its AI strategy. As noted, while pre-training on NVIDIA hardware remains standard due to its compute intensity, fine-tuning and inference—less resource-intensive phases—are being transitioned to AWS’s silicon.
This shift highlights AWS’s focus on cost-effectiveness, contrasting sharply with the high costs associated with training competitors’ models. For instance, Google’s Gemini Ultra reportedly cost nearly $200 million to train, underscoring the financial burden of building large-scale GenAI models.
The Competitive Landscape: Nova vs. Industry Giants
- Flexibility and Optionality: AWS favors Anthropic but its decision to offer other industry models reflects its strategy of enabling customer choice, a core tenet of its overall approach.
- Long-Term Vision: The roadmap for multimodal-to-multimodal models demonstrates AWS’s intention to try and lead in advanced GenAI capabilities.
- Strategic Comparisons: Nova’s design parallels Graviton’s approach to x86 CPUs, providing a signal that AWS views foundation models as essential to its infrastructure portfolio.
The Road Ahead: AWS’s GenAI Ambitions
AWS appears committed to a long-term strategy of innovation in GenAI. While still leveraging NVIDIA for pre-training, its investments in Trainium and other in-house silicon solutions position it to gradually reduce reliance on external vendors. The Nova models are an essential step in building the technical and operational capabilities required to compete in an increasingly AI-driven world.
With a clear roadmap, competitive pricing, and a focus on optionality, AWS is signaling that it is not just participating in the GenAI race but is determined to shape its trajectory.
AWS SageMaker Evolves – Toward a Unified Data Platform
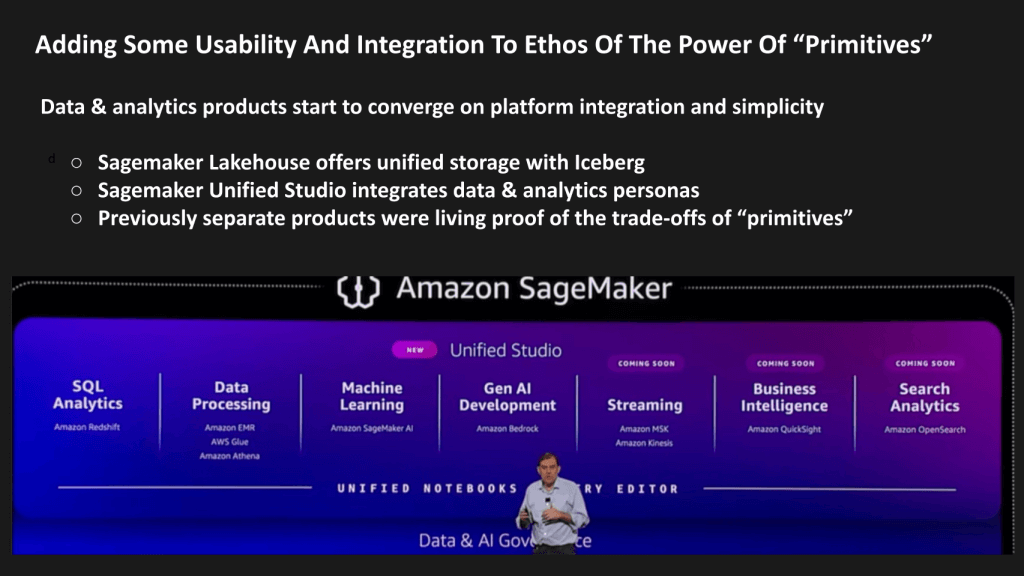
AWS continues to push forward in the data and analytics space, balancing its core ethos of power primitives with the industry’s demand for simplification. At re:Invent, Amazon unveiled significant updates to SageMaker, signaling its ambition to redefine the data and analytics landscape. Historically, AWS’s data platform struggled to compete as a cohesive solution. The updates position SageMaker as a robust, unified platform, aimed at simplifying workflows for data and analytics professionals while preserving flexibility for developers.
Key Takeaways: A Unified SageMaker Ecosystem
- Unification Across Personas:
- The new SageMaker Studio integrates previously disparate tools into a cohesive platform, catering to a broad range of personas, from data engineers to business analysts.
- S3 Iceberg Tables: A critical advancement, enabling managed Iceberg tables with quasi-unified metadata that bridges operational and technical data, incorporating Amazon DataZone within Sagemaker Catalog.
- Bedrock Integration: Amazon integrates Bedrock into its new SageMaker platform through the Amazon Bedrock IDE, which is now part of Amazon SageMaker Unified Studio (preview).
- The promise of read-write capabilities for Iceberg Tables in GA is beneficial to customers as it doesn’t exist in competing platforms today. Our understanding is Databricks is closing in on unifying Delta and Iceberg with read write capabilities targeted for early next year. It’s unclear what this means for Snowflake, Polaris and Horizon. We reached out to Snowflake to get an on the record comment, which we summarize at the end of this section.
- Addressing Historical Shortcomings:
- AWS has long been seen as the “redheaded stepchild” in data and analytics due to fragmented tools and a lack of cohesion compared to competitors like Snowflake, Databricks, and Microsoft.
- This new platform makes strides in unifying the storage and metadata layers, critical for transforming data into a strategic, unified asset.
- Convergence of Data, Analytics, and AI:
- The convergence of AI and analytics tools within SageMaker allows for a seamless pipeline, from ingesting and refining data to analytics feeding feature stores and LLMs.
- SageMaker Lakehouse now bridges the gap between storage and analytics, while integrating personas ensures accessibility across the enterprise.
Summary of Snowflake’s take on the AWS announcements: Snowflake underscored its growing partnership with AWS, citing 68% year-over-year growth in bookings and recognition with nine AWS re:Invent 2024 awards, including “Global Data and Analytics ISV Partner of the Year.” The collaboration spans integrations like Snowpark for Python with SageMaker and joint work on Apache Iceberg to enhance data interoperability, reduce complexity, and mitigate vendor lock-in. Snowflake’s unified platform approach, supported by its Datavolo acquisition, accelerates AI application development and maintains robust governance through its Horizon Catalog. Highlighting AWS SageMaker’s recent improvements and its shared commitment to Apache Iceberg, Snowflake announced a multi-year partnership with Anthropic to integrate Claude 3.5 models into its Cortex AI platform on AWS and expressed plans to deepen collaboration in analytics and AI innovation.
Our take on Snowflake’s response is it’s no surprise the company would put forth an optimistic stance. As well, Snowflake and AWS (and Databricks) have shown this is not a zero sum game. At the same time we continue to feel that Snowflake’s primary competitive advantage is its integrated experience and the quality of its core database. As we’ve previously discussed, the value is shifting up the stack toward the governance catalog, which is becoming more open. This in our view will push Snowflake in new directions which their response acknowledges.
From the Databricks perspective, we believe what AWS announced validates much of that which Databricks CEO Ali Ghodsi has been espousing, that open formats will win and customers want to bring any compute to any data source.
The Competitive Landscape: Metadata as a Key Battleground
As AWS moves more toward a unified data platform, metadata emerges as the key value layer. This harmonization or semantic layer transforms technical metadata into business-relevant information—people, places, things, and their relationships.
- The Metadata Gap: Today, no conventional data platform has fully addressed the harmonization layer. Leaders in this direction include Salesforce, Celonis, RelationalAI, and Palantir within their specific contexts.
- The Opportunity: AWS’s updates to SageMaker suggest it’s targeting this space, evolving from an infrastructure-first mindset to a business process-driven approach, bridging SQL systems with knowledge graphs.
Why Metadata and Semantic Layers Matter
- From Strings to Things: Traditional platforms focus on data snapshots (strings), representing past states. The next step is modeling real-world entities (people, places, activities) and their relationships to enable forward-looking insights.
- Processes and Knowledge Graphs: Incorporating business processes into this semantic layer shifts the focus from infrastructure to applications, creating a cohesive model of the business that drives insights and automation.
Challenges and Market Implications
Skepticism remains about AWS’s ability to execute at this level of integration. Industry experts have previously doubted Amazon’s capability to unify its platform effectively. However, the reimagined SageMaker, with its unified storage and metadata layers, represents a meaningful step forward.
The updates will intensify competition with Snowflake, Databricks, and others in the battle for enterprise data dominance. As AWS builds out its platform, market confusion could grow as enterprises weigh the benefits of SageMaker versus other well-established data platforms.
The Road Ahead: AWS’s Data Ambitions
The new and improved SageMaker signals AWS’s commitment to delivering a unified platform that simplifies workflows without sacrificing power. While competitors like Snowflake and Databricks have led the charge in unifying tools and storage, AWS is rapidly closing the gap by converging data, analytics, and AI capabilities under one umbrella.
AWS’s roadmap points toward greater integration, including knowledge graph capabilities and semantic layers. By bridging its infrastructure expertise with application-centric design, AWS is positioned to redefine how enterprises harness the power of their data.
This evolution will determine whether AWS can shift from a fragmented toolkit provider to a leader in unified data platforms. The stakes are high because we believe ultimate agentic architectures won’t scale without this harmonization capability. We believe Amazon is laying the foundation with SageMaker and AWS is prepared to compete at the highest levels of the data ecosystem.
Q Developer – Ushering in a Usability Revolution for Cloud and AI Throughout the SDLC
AWS continues to bridge its core ethos of “primitives for builders” with the need for usability and integration. A standout example of this strategy is Q Developer, a suite aimed at transforming the software development lifecycle by introducing agents that automate non-coding tasks such as documentation, unit testing, and code reviews. This represents a major step forward in simplifying cloud and AI development, making these capabilities more accessible to developers and enterprises alike.
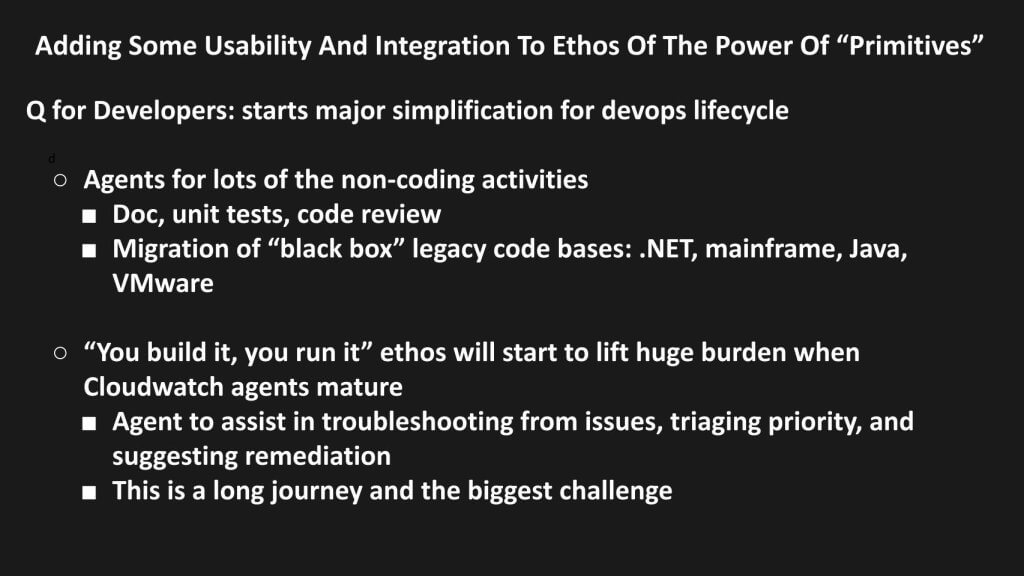
At the same time, AWS is pursuing legacy modernization efforts, targeting workloads like mainframes, Windows/.NET, and VMware, with varying degrees of success and challenges. While these efforts showcase AWS’s ambition, the real headline is the growing ecosystem around GenAI-powered development agents, which AWS is integrating into its services, signaling a new era of productivity and accessibility for developers.
Key Takeaways: Q Developer and the GenAI Ecosystem
- Revolutionizing Developer Productivity:
- Q Developer integrates AI agents to handle non-coding activities, significantly easing the burden on developers and enhancing productivity.
- The suite exposes AWS services in a way that allows third-party GenAI development agents (e.g., Poolside, Cursor, Replit) to fit seamlessly into AWS’s ecosystem.
- This combination marks a usability revolution, making cloud services more consumable for developers and aligning with broader trends in low-code/no-code tools.
- Legacy Modernization Efforts:
- AWS continues its push to modernize legacy workloads, particularly:
- Mainframes: While AWS claims to compress migration timelines from years to quarters, the success in this area remains to be seen. We are skeptical that this will move the needle.
- Windows/.NET: AWS has become a major destination for Windows workloads, competing directly with Microsoft in retaining these applications.
- VMware: Targeting VMware environments appears more straightforward, leveraging AWS’s existing relationships and tooling, migrating customers from VMware Cloud on AWS.
- AWS continues its push to modernize legacy workloads, particularly:
- Long-Term Vision for Proactive Management:
- AWS’s telemetry data collection aims to proactively manage services by identifying issues, suggesting fixes, and even automating remediation. However, this remains an ambitious, long-term journey due to the complexity of building coherent models from a fragmented set of services.
- The Killer App for GenAI: Software Development Agents:
- GenAI-powered development agents are emerging as a killer app for GenAI. These agents assist developers in navigating AWS services more easily, creating a synergy between automation tools and AWS primitives.
- The combination of GenAI development agents and AWS services is one of the most significant advancements in cloud development usability since the inception of the cloud.
Developers: From Cloud Kingmakers to AI Kingmakers?
As cloud evolves, so too does the role of developers. While they have long been the “kingmakers” in cloud, the rise of GenAI and low-code tools signals a shift in focus toward data-centric application development. In this context:
- Microsoft and Salesforce Advantage: Tools like Microsoft’s Power Platform and Salesforce’s Agentforce leverage coherent sources of truth, enabling GUI-based drag-and-drop application development. These platforms offer a strong foundation for low-code developers, enabling them to build data-driven applications efficiently.
- AWS’s Challenge: AWS must balance its traditional developer-centric approach with the growing demand for low-code solutions that empower business users and analysts.
Harmonizing Data and Metadata: The Future of AI Development
The interplay between GenAI agents and harmonized metadata is central to this usability revolution. By integrating operational and technical metadata, AWS is creating a framework where agents can navigate seamlessly across datasets and services. This approach aligns with the broader vision of an AI-driven development ecosystem:
- Unified Metadata: The harmonization of operational and technical metadata acts as a central traffic system, guiding agents and ensuring a coherent source of truth.
- AI-Driven Insights: By embedding intelligence into development tools, AWS is enabling developers to focus on innovation while offloading mundane tasks to AI agents.
The Big Takeaway: A Usability Revolution in Cloud Development
AWS’s Q Developer and its integration of GenAI agents signal a paradigm shift in how cloud development is approached. By combining the power of primitives with enhanced usability, AWS is paving the way for a more accessible and productive development environment. This effort positions AWS to compete not just with traditional cloud competitors but also with platforms that emphasize low-code, AI-driven workflows.
As the market evolves, the battle will increasingly focus on usability and integration, where AWS, Microsoft, Salesforce, and others compete to define the next generation of software development. AWS’s success will hinge on its ability to harmonize its deep infrastructure expertise with the growing demand for developer and enterprise simplicity.
An Early Foray into Copilot-Style Information Access
Q Business represents a nascent attempt to bring “Copilot”-like functionality to the enterprise, echoing Microsoft 365 Copilot’s promise. This early iteration from AWS seeks to unify collaboration and business data under a single index, enabling Large Language Model (LLM) queries. The potential benefits are substantial but in our view will take more time to evolve:
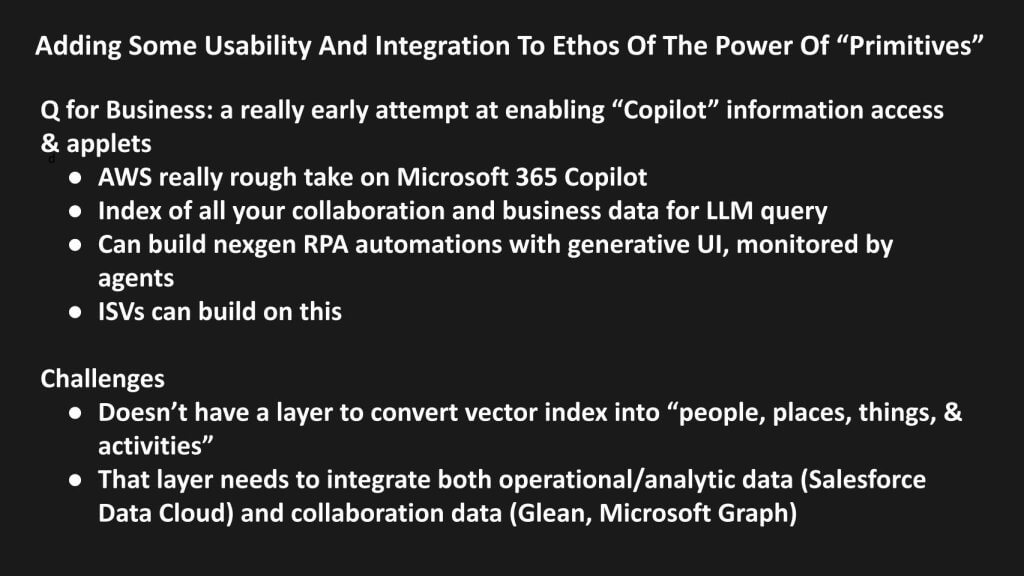
Key Features
- Unified Index: Consolidates collaboration and business data, facilitating seamless LLM queries.
- Next-Gen RPA Automations: Empowers the creation of advanced automations with generative UI, overseen by agents.
- ISV Ecosystem: Offers a platform for Independent Software Vendors (ISVs) to build upon.
Challenges Ahead
However, Q Business faces significant hurdles:
- Entity Disambiguation: Lacking a layer to convert vector indexes into meaningful entities (people, places, things, and activities).
- Data Integration: Requires seamless integration of operational/analytic data (e.g., Salesforce Data Cloud) and collaboration data (e.g., Glean, Microsoft Graph) to unlock its full potential.
As Q Business continues to evolve, addressing these challenges will be crucial to realizing its vision of streamlined information access and automation.
Tracking Momentum and Penetration in the ML/AI Landscape
The chart below provides a classic view into spending momentum and market penetration within the ML and AI ecosystem. Using ETR data from 1,775 enterprise IT decision-makers, the vertical axis reflects Net Score, a measure of spending momentum—essentially the percentage of customers increasing their spending on a platform. The horizontal axis, labeled as Overlap, represents penetration within the surveyed accounts. Together, these metrics highlight both platform momentum and adoption across the ML/AI market. The red line at 40% on the vertical axis represents a highly elevated Net Score.
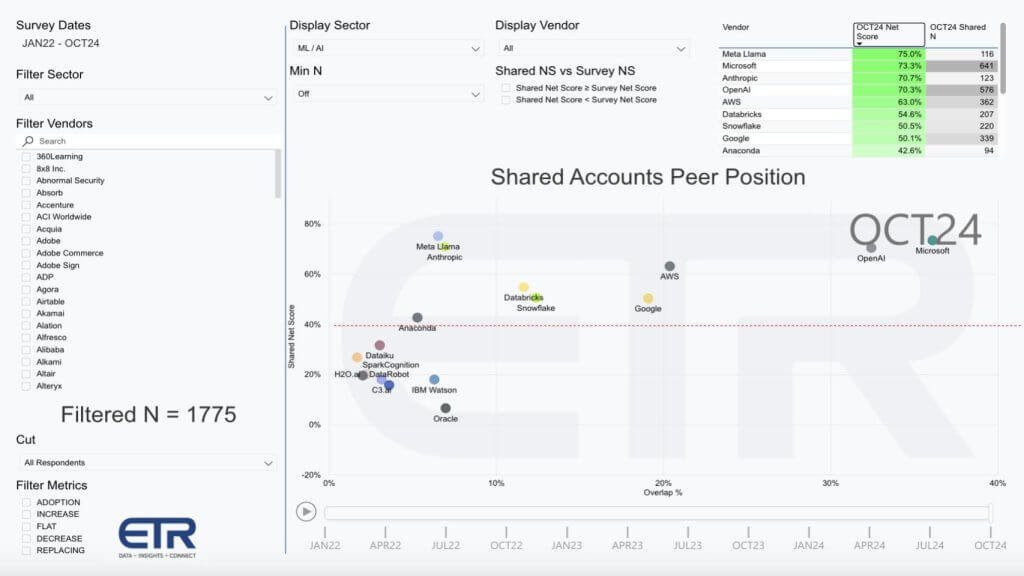
Key Insights: Leaders and Emerging Contenders
- Dominance in Momentum and Penetration:
- OpenAI and Microsoft continue to dominate the horizontal axis with strong penetration across enterprise accounts, reflecting widespread adoption of their AI solutions.
- Meta’s Llama makes a significant leap, overtaking both Microsoft and Anthropic in spending momentum for the first time. This signals increased activity and interest around Llama, particularly among enterprises experimenting with open foundational models.
- Anthropic’s Position Through Bedrock:
- Anthropic maintains strong momentum, ranking close to Meta Llama, Microsoft and OpenAI. A significant portion of Anthropic’s enterprise traction is facilitated through AWS Bedrock, highlighting Amazon’s role in extending Anthropic’s reach within its ecosystem.
- Google’s Momentum in the ML/AI sector has been notable since the October 2022 survey. Google has shown steady increases in Net Score, peaking at 62% in the July survey and pulling back in the latest polling to 50%, still highly elevated with a meaningful presence in the data set.
- GenAI’s Role in Data Platform Players:
- Databricks and Snowflake are solidifying their positions within the ML/AI space. Both companies are leveraging their data estate strategies, with Databricks building on its established ML/AI foundation and Snowflake entering the space more recently but showing encouraging momentum.
- Their integration of GenAI capabilities underscores the importance of AI in driving data platform differentiation.
- Legacy AI Players:
- Companies like Dataiku, DataRobot, C3 AI, and Anaconda continue to hold their positions but lag behind in terms of both momentum and penetration compared to newer GenAI-driven players.
- IBM Watson shows a slight uptick in momentum, signaling renewed interest, but IBM Granite and other recent offerings are not yet prominent in this data.
- Oracle is a player within its confined ecosystem.
Notable Observations and Gaps:
- Amazon Nova: While AWS Bedrock is driving Anthropic adoption, Amazon’s Nova models are not yet represented in this data.
- Legacy Players in Niches: Legacy AI platforms, including Oracle and IBM Watson, show limited but noticeable presence. IBM Watson’s uptick is worth watching for potential resurgence.
- Emerging Dominance of Meta Llama: Meta’s rapid rise in momentum reflects the growing appeal of its Llama models, likely driven by the accessibility and versatility of open foundational models. This momentum is a key development to monitor.
Framing the Competitive Landscape:
- Momentum Leaders:
- OpenAI, Microsoft, Meta Llama, and Anthropic dominate the high-momentum, high spending velocity space, reflecting their leadership in both adoption and innovation.
- Data Platform Leaders:
- Databricks and Snowflake are leveraging their data-first strategies to carve out a significant space in GenAI and ML/AI, aligning their tools with enterprise AI demand.
- As enterprises integrate GenAI into their workflows, these data platforms will likely see sustained momentum.
- The Legacy Guard:
- Players like IBM Watson and Oracle represent legacy AI platforms attempting to maintain relevance, but their influence remains limited in the context of modern GenAI leaders with greater account penetration. That stated, both companies command premium pricing and sell value with significant drag for other products and services that drive revenue.
This snapshot of the ML/AI sector highlights a evolving competitive landscape where foundational model players like OpenAI, Meta Llama, and Anthropic are redefining the space. Data-centric platforms such as Databricks and Snowflake are integrating AI to remain competitive, while legacy players like IBM and Oracle are fighting to stay relevant.
AWS, Nova models may move the needle but it’s likely Anthropic will continue to be the dominant platform in the ETR data AWS will in our view continue to exert influence through Bedrock and its other partnerships and its optionality strategy is playing out as planned. As enterprises increasingly view AI as foundational to their strategies, the momentum and penetration of these platforms will shape the next wave of innovation in the ML/AI ecosystem.
The Emerging Harmonization Layer – Toward a Virtual Assembly Line of Expertise
The evolving software stack (shown below) is pushing beyond traditional silos of infrastructure, platform services, and SaaS applications. We believe the imperative for the next decade is the creation of a harmonization layer—a transformative new abstraction that orchestrates end-to-end business processes, aligning activities like demand forecasting, internal operations, and supplier coordination into a seamless whole.
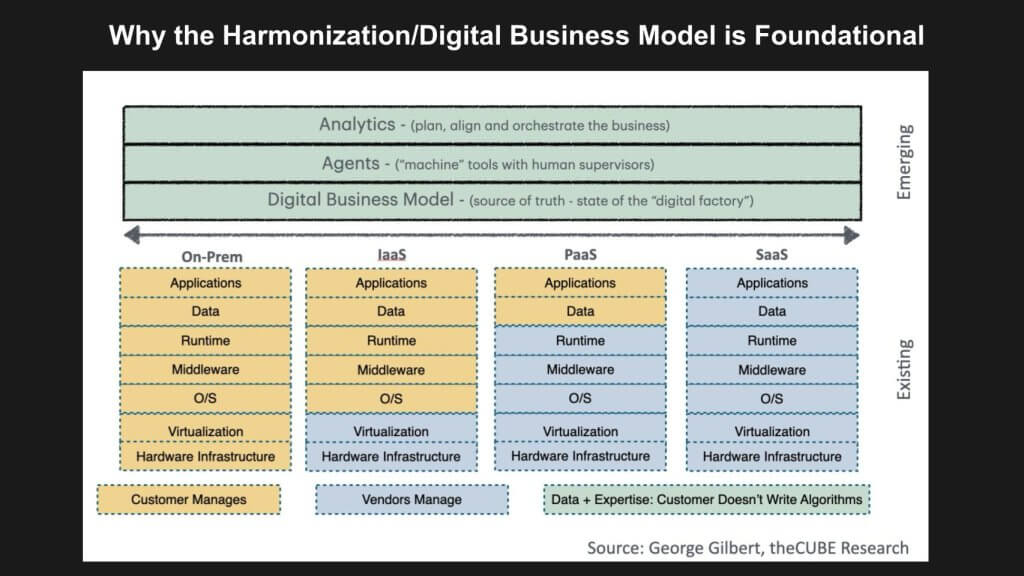
We describe this vision as a “virtual assembly line of expertise,” which seeks to achieve for managerial and collaborative work what the physical assembly line did for manufacturing a century ago: optimize throughput, eliminate inefficiencies, and enable expertise to flow seamlessly across the organization.
Key Takeaways: The Role and Evolution of the Harmonization Layer
- From Silos to Orchestration:
- For 60 years, enterprise IT has been characterized by islands of applications and analytics. While these have enabled incremental automation, they lack the ability to connect processes into a coherent, predictive framework.
- The harmonization layer aims to unify these silos, spreading a layer of intelligence across disparate systems and turning fragmented work cells into a predictive assembly line.
- Foundations of the New Application Platform:
- The harmonization layer is built on top of coherent data foundations like open table formats (OTFs) like Iceberg tables, but it requires an equally coherent metadata layer. This layer transforms operational and technical data into a knowledge graph of the business, linking people, places, activities, and processes.
- Emerging Players and Approaches:
- Companies like Celonis, RelationalAI, and EnterpriseWeb are pioneering this space, focusing on integrating and optimizing business processes.
- Collaborative tools like Microsoft’s 365 Graph and startups like Glean are developing knowledge graphs that contextualize operational and collaborative data, bridging the gap between disparate systems. We’ve also seen Kubiya.ai show some early promise in developer environments.
- Agentic Frameworks and Governance:
- The rise of AI agents necessitates robust governance frameworks to manage agents, enforce access control, and ensure aligned outcomes.
- While Amazon’s Q Business is indexing operational and collaborative data into a vector index, more sophisticated platforms like Glean and Microsoft’s tools are advancing toward creating knowledge graphs that enable deeper contextualization and insights.
Transformative Potential and Industry Challenges
- Automation’s Untapped Opportunity:
- A significant portion of enterprise processes remains non-automated, representing a 10x multiplier opportunity. SaaS and custom applications have only scratched the surface, leaving vast areas for innovation in end-to-end process alignment.
- This opportunity underscores the importance of the harmonization layer as the key to unlocking exponential productivity gains.
- The Decade-Long Journey:
- The top layers of the emerging stack, represented by green areas in the diagram, remain undefined and are the focus of intense innovation. Startups, SaaS companies, and hyperscalers like AWS, Google and Microsoft are all vying to define this space.
- Building a cohesive harmonization layer will require sustained efforts over the next decade, with incremental advancements shaping the journey.
- Knowledge Graphs as a Core Component:
- Knowledge graphs represent a critical building block of the harmonization layer, contextualizing data and processes for actionable insights. For example:
- Celonis integrates business process intelligence.
- Glean focuses on collaborative data.
- Kubiya AI applies these concepts to the developer lifecycle.
- Knowledge graphs represent a critical building block of the harmonization layer, contextualizing data and processes for actionable insights. For example:
A Virtual Assembly Line of Expertise: The New Paradigm
This new harmonization layer represents a seismic shift in enterprise IT. By extending the concepts of databases and ERP systems (e.g., Oracle and SAP) across the entire data and application estate, this layer creates a predictive, interconnected framework that transcends traditional silos.
- From Snapshots to Predictions: Moving beyond static snapshots of processes, the harmonization layer enables dynamic predictions and real-time alignment of activities.
- From Strings to Activities: The focus shifts from data as isolated strings to interconnected entities (people, places, things) and their activities.
Closing Perspective: The Long Road to Transformation
The emergence of the harmonization layer is nothing short of transformative, redefining how enterprises align their processes and leverage expertise. However, this evolution is still in its early stages, with significant challenges in governance, agent integration, and metadata harmonization.
As AWS, Microsoft, and startups like Glean, Celonis, and Kubiya AI push the boundaries of this space, the journey will define the next generation of application platforms. While the payoff may take a decade or more, the potential to revolutionize enterprise productivity and collaboration is undeniable.
What do you think? Did you catch the action at AWS re:Invent 2024? What caught your attention that we didn’t touch upon? Are there things in this research note you disagree with or to which you can add context?
Let us know.