We are in the midst of a fundamental transformation of computing architectures. We’re moving from a world where we create data, store it, retrieve it, harmonize it and present it, so that we can make better decisions, to a world that creates content from knowledge using tokens as a new unit of value; and increasingly takes action in real time with or without human intervention, driving unprecedented increases in utility. What this means is every part of the computing stack, from silicon, infrastructure, security, middleware, development tools, applications and even services, is changing.
As with other waves in computing, consumer adoption leads us up the innovation curve where the value is clear, the volume is high and the velocity is accelerated, translating to lower costs and eventual adoption by and disruption of enterprise applications. Importantly, to do this work on today’s data center infrastructure would be 10X more expensive, trending toward 100X by the end of the decade. As such, virtually everything is going to move to this new model of computing.
In this Breaking Analysis we quantify three vectors of AI opportunity laid out by Jensen Huang at this year’s GTC conference: 1) AI in the cloud; 2) AI in the enterprise; and 3) AI in the real world. And we’ll introduce a new forecasting methodology developed by theCUBE Research’s David Floyer, to better understand and predict how disruptive markets evolve.
Market Uncertainty Softens IT Spending Expectations
ITDMs pull back spending outlook to 3.4% for the year, down from 5.3% and below 2024 levels
Markets remain under pressure, exacerbated by ongoing tariff concerns and back-to-back declines in key indices—most notably the tech heavy NASDAQ. Stocks have had their worst week since March of 2020. Against this backdrop, the latest recent spending data from ETR’s quarterly survey of IT decision-makers reflects a notable shift in spending expectations over the past year as shown below.
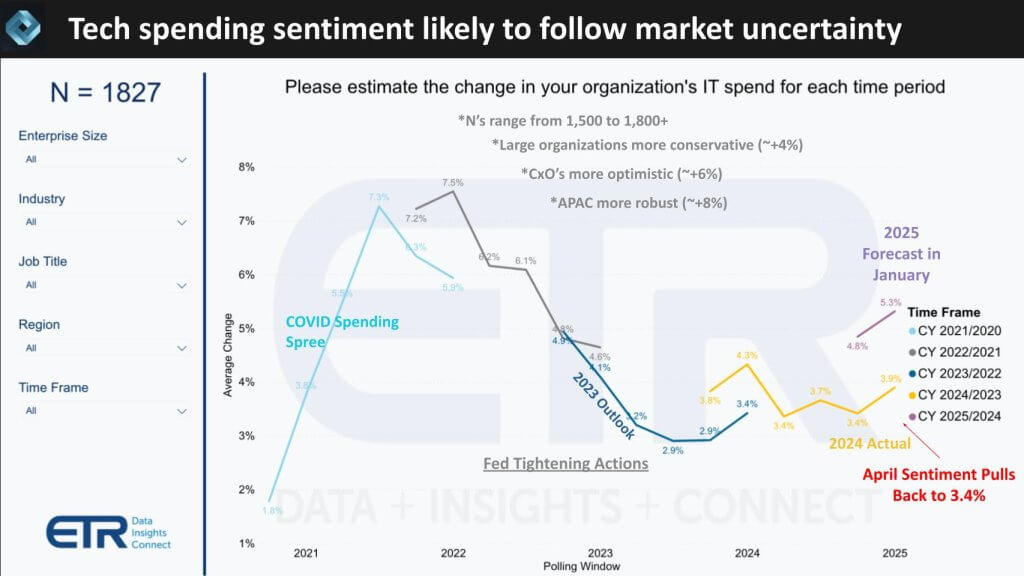
Coming out of the pandemic, the “COVID spending spree” drove projected IT budget growth above 7%. As the Federal Reserve tightened monetary policy, that figure bottomed below 3%. In 2024, overall IT spending growth settled around 3.9%, and by January of 2025, survey respondents indicated a rise to 5.3%—an improvement from 4.8% in the prior October survey. However, the April sentiment shows a concerning pullback, dropping from those optimistic levels down to 3.4%.
Although ETR has not yet finalized these numbers, the downward revision is noteworthy. Not only does 3.4% fall short of the early-year projection, it also places IT spending below last year’s baseline. This shift underscores the mounting uncertainty in today’s macroeconomic climate and reveals a pronounced dip in confidence among enterprise technology buyers.
AI Spending Remains a Priority
Majority of ITDMs are staying the course on AI spending patterns
ETR’s latest drilldown data indicates that public policy pressures around AI—ranging from tariffs to privacy and regulation—are not yet significantly dampening AI spending plans. In a sample of approximately 500 respondents, just under half report maintaining or even accelerating their AI initiatives, largely to stay ahead competitively. Meanwhile, a sizable middle contingent plans to proceed at a steady pace, monitoring developments without making dramatic shifts. Notably, fewer than 10% are pumping the brakes due to policy-related concerns (see below).
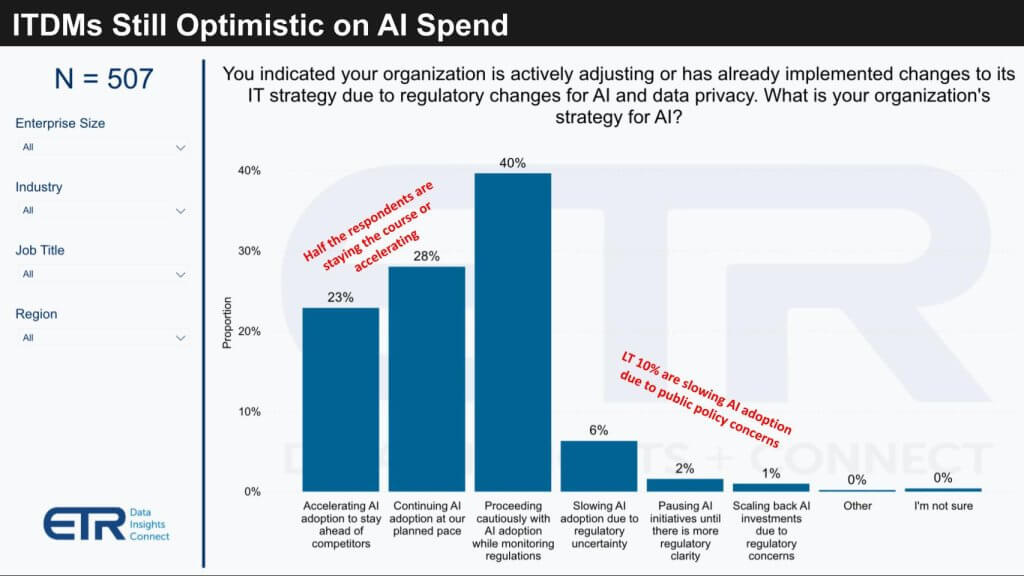
While broader macroeconomic headwinds are evident elsewhere in the data, this specific snapshot suggests that enterprise AI momentum remains largely intact. Still, a degree of caution persists. Some organizations appear to be grappling with the enormity of AI’s potential disruption, hoping regulatory uncertainties may resolve—or possibly fade. Despite recent market volatility, these survey findings underscore an ongoing drive to explore and implement AI initiatives, indicating that the technology’s disruptive promise is outpacing immediate policy concerns.
Revisiting the Data Center Supercycle 2024 – 2035
In his keynote at this past GTC, Jensen Huang laid out three primary AI opportunities, including: 1) AI in the public cloud; 2) AI in the enterprise – i.e. on-prem; and 3) AI in the real world – i.e. physical robots. Our goal today is to break down the data center forecast shown below into Jensen’s first two opportunities. And we’ll frame how we see AI in the real world evolving by applying a new forecasting methodology.
The hyperscalers have emerged as pivotal enablers by providing massive compute capacity and skilled teams with the expertise to stand up large‐scale AI “factories.” The cloud segment currently dominates AI infrastructure build‐outs, especially given the success and acceleration of consumer‐oriented services such as OpenAI, Meta, Apple, ByteDance, and TikTok. More specifically, consumer ROI is clear and dollars spent in consumer applications are paying dividends.
The second vector—AI in the enterprise—encompasses on‐premises or private data center deployments. While on‐prem stacks often encounter friction due to data gravity and incomplete AI‐specific infrastructure, organizations increasingly seek to bring AI to their data rather than move data offsite. Several major OEMs, including Dell and HPE, have introduced “AI factory” offerings tailored for enterprise data centers. However, many of these solutions remain heavily hardware‐centric. The emerging ecosystem of software components, AI‐optimized workflows, and specialized talent is still taking shape, suggesting ongoing build‐out before enterprise AI reaches maturity.
The third arena Jensen Huang highlighted is AI’s role in the “real world,” specifically physical robotics. From single‐purpose, task‐based machines to versatile humanoids, the potential is vast. Platforms such as NVIDIA Isaac—recently demonstrated via the Blue humanoid—foreshadow a future where AI moves well beyond virtual interactions and enters factories, warehouses, and ultimately everyday settings. This third area of opportunity is not included in our data center forecasts shown here.
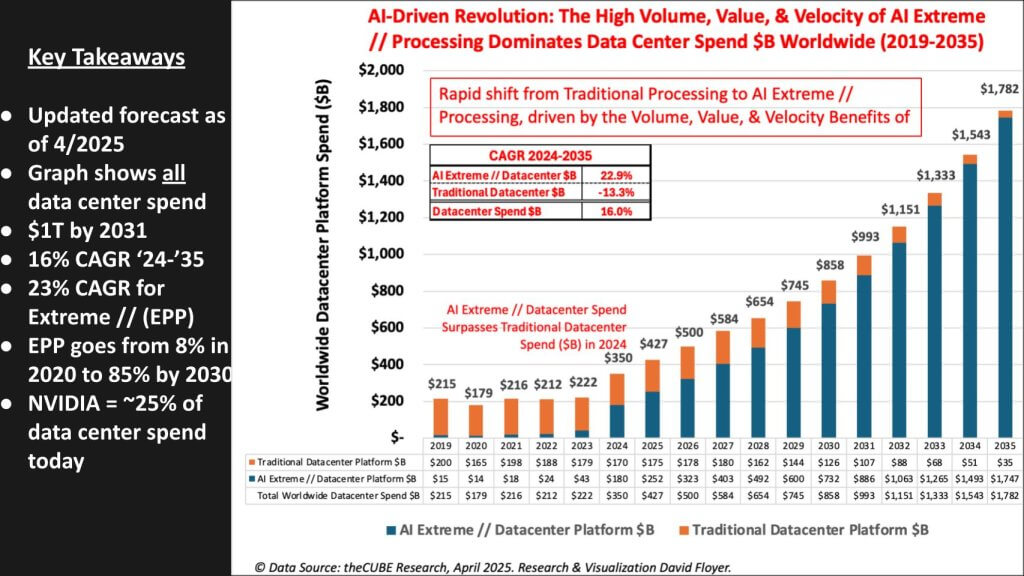
To underscore the magnitude of these shifts, our updated forecasts project a dramatic rise in accelerated computing or what we call Extreme Parallel Processing (EPP). Our analysis shows worldwide data center spending growing at a 16% compound annual growth rate (CAGR) over an 11‐year horizon, reaching a trillion dollars by the early 2030s. Within that total, EPP, or accelerated computing, is set to climb at a 23% CAGR, driving the market from roughly $43B to $180B in a single year (2024). This was the true beginning of the supercycle. By contrast, traditional computing, dominated by x86‐centric infrastructures, continue a slow decline—reflecting the growing emphasis on GPU‐ and AI‐optimized architectures.
In practical terms, this shift means that by 2035, overall IT spending could be ten times higher than it was in 2024, with much of that outlay dedicated to advanced AI data centers. While organizations may be cautious about transforming core applications and retooling legacy code, the trajectory of spending indicates a clear pivot. Over time, the center of innovation and budget allocation is poised to move decisively from legacy systems toward AI‐driven infrastructure and workflows.
Our research shows accelerated computing moving from under 10% of total data center spending in 2020 to 85% by 2030. While a large portion of this rapid growth is being driven by public cloud hyperscalers, on‐premises enterprise deployments are also beginning to take shape. The next step is to break out the portion of spending that directly pertains to private data centers.
Introducing a New Method to Forecast Disruptive Markets
Applying volume, value and velocity to forecast the AI opportunity
To project these shifts effectively, a forecasting approach that uses but goes beyond classic Wright’s Law is necessary. Wright’s Law states that costs decline in a predictable manner as cumulative production doubles, but current market dynamics demand additional dimensions. Our methodology incorporates “Volume, Value, and Velocity” (3Vs) to capture how disruptive technologies like AI can undergo faster and deeper adoption:
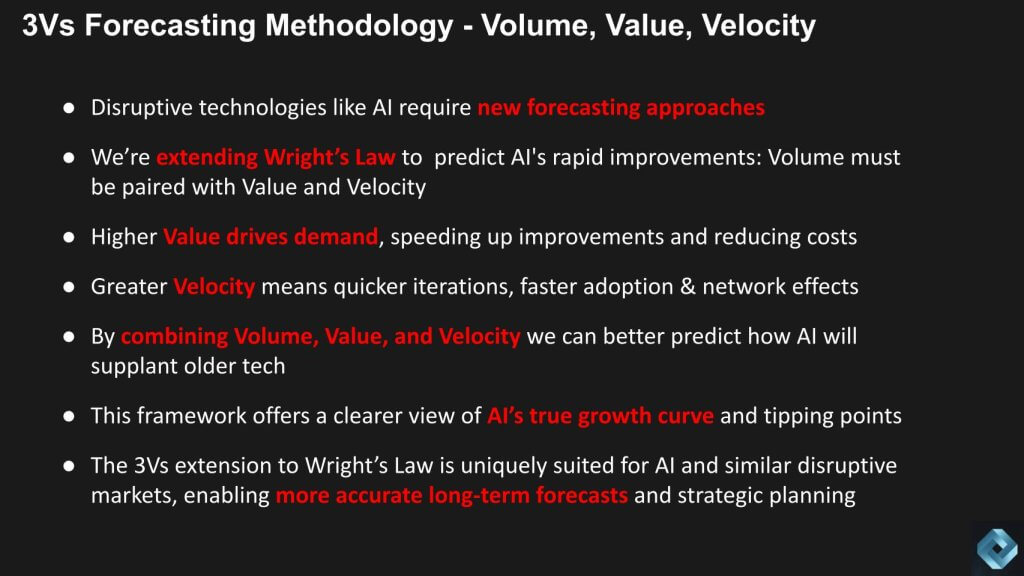
- Volume: Increasing scale drives down cost, consistent with Wright’s Law.
- Value: Higher perceived benefits (e.g., improved productivity, lower energy usage) raise demand, funnel more R&D investment, and further reduce cost.
- Velocity: Ease of deployment and frequent use speed up design cycles, adoption, and network effects, intensifying both the cost decline and the pace at which new technologies replace older systems. Velocity can also have a drag effect if legacy environments, regulation, or complex integration requirements slow down deployment.
AI provides unprecedented value in both consumer (today) and enterprise (eventually) domains, creating a powerful incentive for organizations to accelerate their adoption cycles. Although on‐prem stacks have to address data gravity, software dependencies, and skill gaps, the overall trajectory suggests a steady pivot toward AI‐optimized infrastructure. Our analysis indicates that x86‐centric data centers will remain in place for the foreseeable future, but the momentum behind extreme parallel processing is expected to reshape spending patterns and ecosystem investments in the years ahead.
Cloud Dominates Early AI Adoption – On-prem AI Builds Slowly
Our more granular forecast shown below, isolates just the AI portion of total data center spending, separating the market into public cloud environments and private on‐premises deployments. Cloud currently dominates due to hyperscalers’ advanced tooling, specialized skill sets, and strong consumer‐driven use cases that demonstrate immediate ROI. Platforms such as Meta, Google, ByteDance, and Apple are justifying substantial capital expenditures on AI, which accounts for the lion’s share of near‐term growth. We also include platforms like Grok (xAI) and so-called neo-clouds (e.g. Coreweave) in the cloud segment shown below in the dark blue bars.
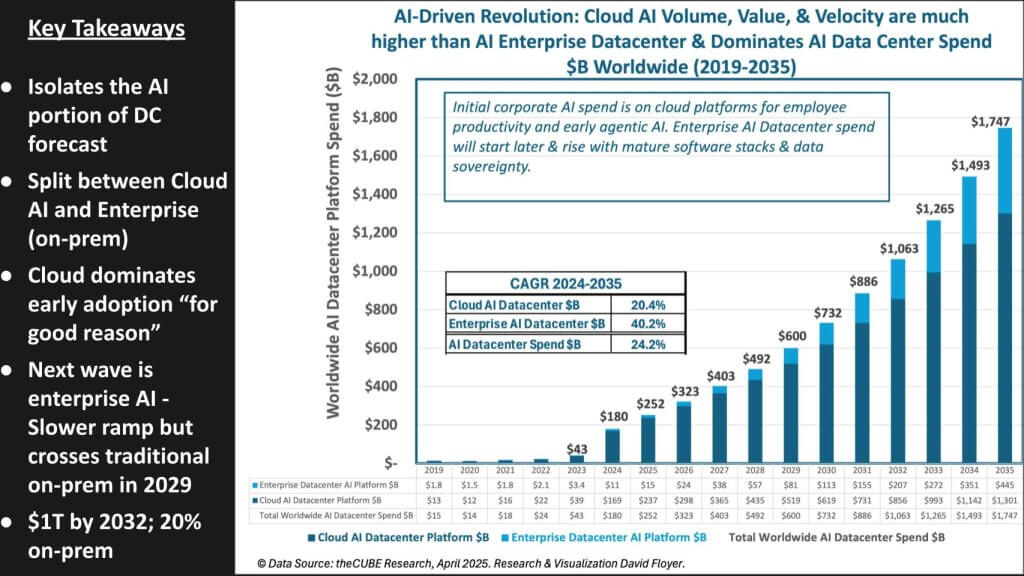
On‐premises enterprise infrastructure shown in the light blue above, shows a more gradual ramp, reaching steeper adoption curves around 2026 and accelerating into 2029. The relative delay reflects a number of factors, including a lack of fully evolved solution stacks, fewer in‐house AI experts, and the added complexity of modernizing legacy data and applications. Although certain large institutions—particularly in financial services—can invest heavily in retooling data centers for liquid cooling, bringing in specialized hardware, building out their own software stacks and managing overall AI stack complexity, many organizations must wait for integrated solutions that address data gravity, governance, and existing transactional systems.
Note: We include collocation facilities like Equinix in the on-prem component of our forecasts.
Our analysis projects a trillion‐dollar AI data center opportunity by approximately 2032, with around 20% of that spending in private enterprise environments. Jensen thanked the audience at GTC for adopting new architectures, such as disaggregated NVLink, liquid cooling, and high‐power racks approaching 120 kilowatts each. While he didn’t say this specifically, this is happening in hyperscaler markets. By contrast, most enterprises still rely on air‐cooled facilities and stacks optimized for general‐purpose computing. Despite the slower start, the momentum around AI on‐prem is expected to gain speed as data harmonization, real‐time processes, and “agentic AI” systems mature. This evolution will require broader access to legacy metadata, transactional platforms, and a more advanced software layer—factors that point to a significant, but lengthier, transition path for traditional data centers.
AI Applied to Robots – Single Purpose vs. Humanoids
Our belief is that robotics is one of the most compelling frontiers for AI, with wide-ranging implications for both closed and open environments. Single-purpose robots—such as those found in factories, transport fleets, and specialized defense applications—demonstrate significant near-term value because they automate well-defined tasks and offer predictable ROI. Closed-system deployments exhibit high velocity, as organizations can design workflows and facilities from scratch for maximum automation. This approach lowers costs, reduces errors, and boosts adaptability, enabling newcomers to capture market share by building AI-native operations that can achieve revenue per employee at a scale few traditional companies can match.
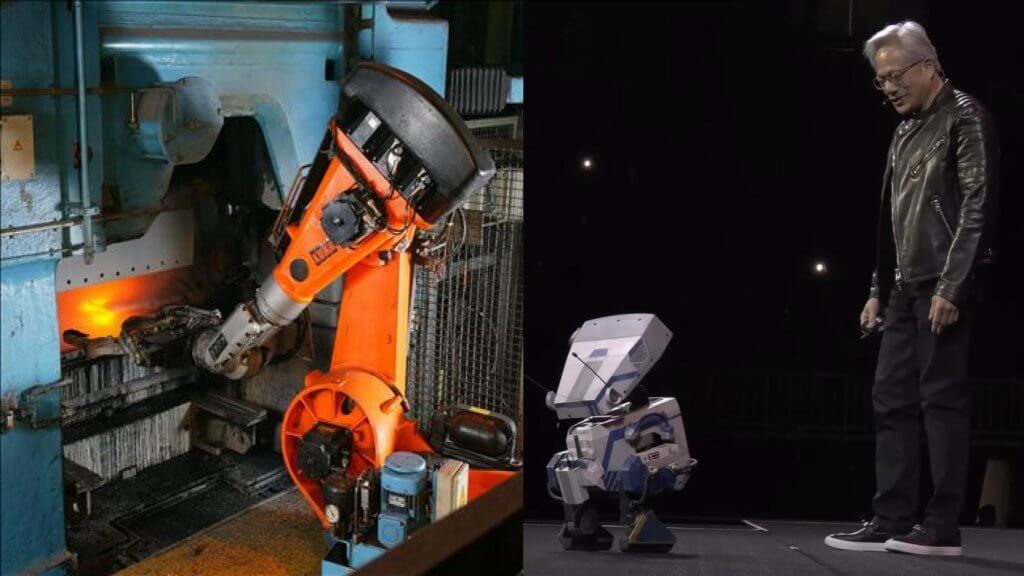
We see a distinct difference between single purpose robots in factories, doing one job really well, and robots that mimic humans and perform a variety of tasks. Jensen wowed the audience with his demonstration of a humanoid on stage but we see clear opportunities in the near term for single purpose automation and believe mimicking humans has a much longer road ahead.
Open-ended humanoid robots in our view face more complex adoption curves. Physical reality introduces an extensive range of edge cases, including unforeseen interactions with humans, other robots, and the environment itself. These factors will temper the velocity of humanoid deployment, and we believe that broader adoption will likely take longer to unfold. Nonetheless, the long-term potential remains vast.
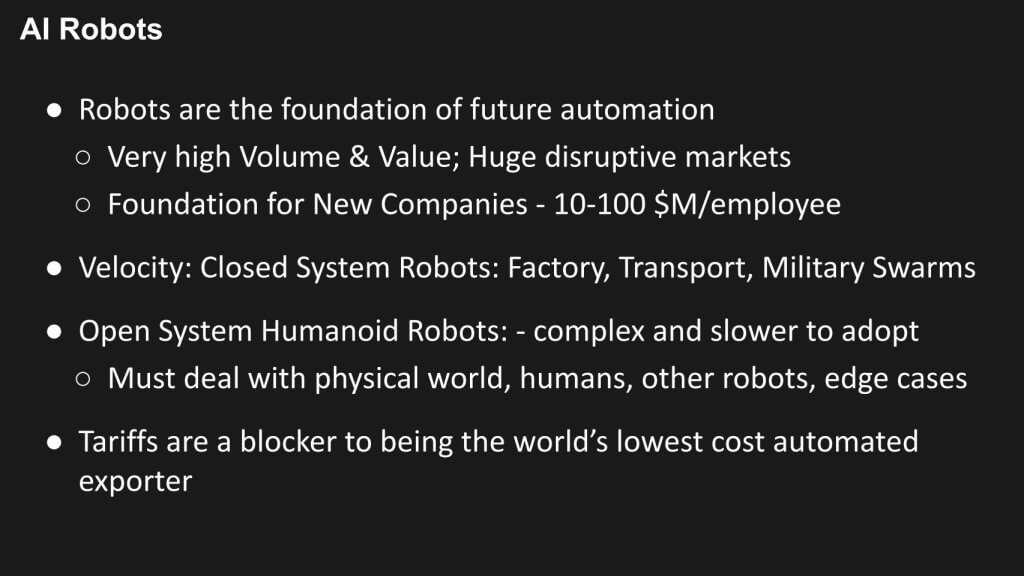
Trade policy also plays a role, as tariffs can slow global adoption by reducing the incentives for automation and giving competitors time to close technological gaps. However, the opportunity to become a leading low-cost exporter of AI-driven goods remains an influential force. If companies or entire regions accelerate investment in robotics, they may replicate the kind of transformation once seen during the world’s Industrial Revolution, where Britain was the low cost provider to the world. The US could replicate that dynamic on a global, AI-fueled scale. But tariffs introduce dislocations to that vision and are backwards-looking. Rather we’d like to see investments in automation where sensible, making the US the world’s low cost producer at scale, and let other countries hide behind tariffs.
In future Breaking Analysis episodes we’ll apply the 3Vs methodology and provide a forecast of these markets in more detail.
A Few Nuggets from GTC
A Wall Street analyst dubbed last year’s GTC the “Woodstock of AI.” Our John Furrier, in the weeks leading up to this year’s GTC, called it the “Super Bowl of AI,” a phrase Jensen used as well. It’s appropriate, building on last year’s watershed moment and highlighting a wholesale transformation of computing architectures that will continue each year like the Super Bowl.
Here we highlight just a few of the many notable takeaways from GTC 2025.
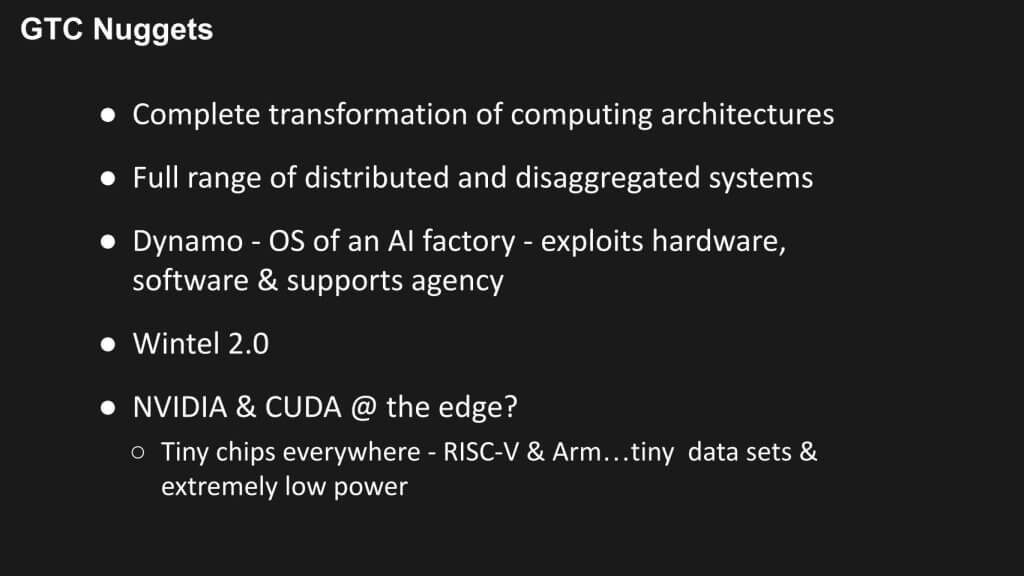
One major focal point is the shift from integrated, monolithic designs toward more disaggregated, distributed systems. These high‐density, liquid‐cooled racks can contain hundreds of thousands of components and deliver exaflop‐scale performance within a single rack—far outstripping conventional data center footprints. The combination of hardware innovation and sophisticated software layers is creating an entirely new systems paradigm, rather than just another generation of GPUs.
A second standout announcement is the release of Dynamo, described as the operating system for the AI factory. This new layer orchestrates inference at scale by managing resource allocation across multiple racks and GPU pools. The design aims to optimize workloads for minimal latency and maximum throughput, laying the foundation for next‐generation distributed AI environments. Over time, inference is projected to account for the largest proportion of AI spending, making a robust OS essential for integrating advanced accelerators, data pipelines, and both x86 and Arm processors.
These developments reinforce what we see as a “Wintel replacement strategy,” where NVIDIA appears positioned to capture the full stack—much like Intel and Microsoft did for PCs. NVIDIA is combining hardware and software in a way that leverages the high volume of consumer‐driven AI while also preparing for enterprise adoption. Historically, the volume advantage propelled x86 and Windows into market dominance. Today’s AI revolution similarly benefits from massive consumer deployments in areas like search, social media, and targeted advertising, which feed breakthroughs and scale economies that ultimately migrate to corporate data centers.
NVIDIA’s Edge Dominance of AI at the Edge is not Sure Bet
At the edge, however, adoption patterns are less certain. A proliferation of ultra‐low‐power chips—such as those based on RISC‐V or alternative minimal‐footprint Arm designs—may capture segments where small data sets, embedded applications, and cost‐efficiency are paramount. While NVIDIA continues to expand its footprint in edge computing, the hardware and energy requirements and constraints differ substantially from those in high‐density AI data centers. As a result, multiple architectures are likely to coexist, especially in use cases that require thousands—or millions—of embedded, power‐constrained devices.
Overall, the core message from GTC is that accelerated computing has moved beyond the realm of specialized GPUs into a fundamentally new model, where advanced fabrics, next‐level operating systems, and massive consumer volume converge to push AI into every layer of technology—from hyperscale clouds and enterprise data centers to the far edges of distributed environments.
AI Watch Party
Below we summarize and highlight several areas we’ll monitor with respect to our forecasts and predictions.
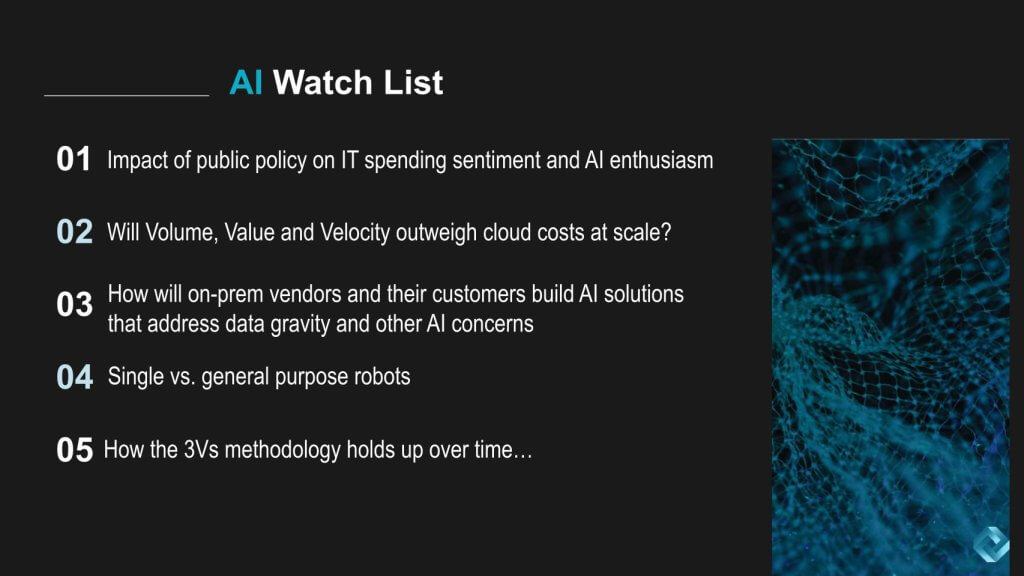
Public policy remains a critical factor to watch, especially in light of ongoing tariffs, macroeconomic shifts, and heightened regulatory conversations around AI. The key question is whether accelerated AI value will continue overshadowing cloud costs at scale, or whether on‐premises suppliers—primarily Dell, HPE, IBM, Lenovo, and to a certain extent Oracle, and others in the ecosystem such as Pure Storage, VAST, Weka, DDN, etc. —will move rapidly enough to deliver AI‐optimized solutions that address data gravity and compliance requirements in a hybrid state. Many enterprise workloads in regulated sectors, such as financial services, healthcare, and manufacturing, have never fully transitioned to the public cloud, creating an opening for on‐prem incumbents to build specialized, high‐performance AI stacks.
We believe a window of roughly 18–24 months appears to be the timeframe in which on‐prem vendors can prove they can deploy turnkey AI infrastructures at scale. A key question is will past migration trends repeat if public cloud offerings continue to innovate at a faster pace, capture more user volume, and build ecosystem velocity. The opportunity exists for on‐prem vendors to avoid the scenario where cloud simply “washes over” enterprise workloads. Colocation and sovereign models are also playing a role, positioning hybrid‐cloud approaches as an alternative to pure hyperscalers – but much work needs to be done to attract startups and build full stack, opinionated AI solutions for hybrid / on-prem environments.
As indicated, in the future, we’ll quantify the single‐purpose versus general‐purpose robotics markets and further test the Volume, Value, and Velocity (3Vs) forecasting methodology. Early research findings indicate that consumer‐driven AI usage remains a catalyst, fueling investments in hyperscale platforms that could carry over into private data centers. The overall trajectory points toward the most impactful adoption curve in modern history, driven by generative AI breakthroughs that have propelled organizations to the steep portion of the S‐curve far sooner than most industry veterans anticipated.
How do you see the future of AI? What are your organizations doing to apply AI to data that lives on premises? Are you investing in new data center infrastructure such as liquid cooling or are you more cautious and waiting for more proof.
Let us know and thanks for reading.