A Forecasting Framework for Highly Disruptive Technologies
Introduction
Forecasting Disruptive Technologies is difficult but essential. The disruption from AI is likely to be a disruptive technology as profound as electricity was and is being adopted far faster. The rate of improvement of OpenAI’s ChatGPT models from introduction of GPT-3.5 in November 2022 to GPT-4o in early 2025 is astounding. It will be critical for every business, technology provider, and professional to make accurate forecasts to ensure they will be an AI survivor.
There are many forecasting schools of thought. Appendix A lists nine methods, with a brief description of each. I believe that my framework, “Volume, Value & Velocity* Extended Wright’s Law” (number 9 in the list), is uniquely suitable for highly disruptive technologies and is the only one suitable for forecasting AI, likely to be the most significant disruptive technology since electricity.
*Note: there is a comparison between the Gartner 3Vs of big data at the end of this research.
I have made numerous forecasts about emerging technologies in his time at IDC, Wikibon, and theCUBE Research. This research paper discusses the framework used in previous forecasts and develops new forecasts related to AI.
While Wright’s Law focuses on how costs drop as cumulative production (Volume) grows, my framework adds two additional factors—Value and Velocity—to explain why certain technologies can grow shipments fast and displace incumbent technologies.
These forecasts will include the growth of extreme parallel platforms to support AI and the likely splits between Cloud, Enterprise Data Centers, enterprise distributed systems, intermittent network mission-critical distributed devices (automated cars, robots), and consumer-based systems. There will also be forecasts on replaced technologies such as x86 CPUs and storage HDDs.
Classic Wright’s Law: Volume
The starting point is Wright’s Law, based on observations on the cost of manufacturing aircraft. It posits that costs decline at a certain percentage whenever cumulative production doubles. In formula form:
Cost(V) = C0 × V–⍺
Where:
V = cumulative volume
C0 = initial cost at V=1
⍺ = learning exponent (related to how fast costs drop with added volume).
This model has a long track record in aviation, electronics, and more. However, disruptive technologies often exhibit faster improvements than predicted by classic manufacturing alone.
Wright’s Law is fundamentally a learning curve concept describing how some metric improves (decreases) as cumulative experience (volume) rises. Although it’s commonly framed in terms of cost, it is easily applied to any production metric that tends to improve with experience, such as manufacturing time (per unit), labor hours, or defect rates.
Floyer Extension: Volume, Value & Velocity
Adding Value and Velocity factors helps shape how quickly disruptive products emerge and mature and helps define the growth and size of new markets.
Volume is the backbone of Wright’s Law—cumulative production or unit adoption. As volume scales, learning and economies of scale reduce costs.
Value is an additional factor that reflects the benefits delivered by a technology to users, such as higher capabilities, superior performance, lower energy use, or added convenience. Higher values grow demand faster and can shift development and marketing resources into accelerating the adoption of new technology. The value of technology changes over time and can become negative later in life as new technologies replace it.
Velocity is the third additional factor, and it involves how intensely a technology is used and how easily it is adopted. If users employ it constantly (e.g., smartphones, AI accelerators), design iterations speed up, and new applications are developed. In the same way, the lower the effort for technology consumers to adopt new technologies (e.g., training time and cost, setup costs), the easier and quicker a new technology will be adopted. In addition to usage intensity and ease of adoption, Velocity can be affected by network effects, feedback loops, and governmental policy and regulations. Feedback loops, such as using AI to design new AI systems, could accelerate Velocity. Velocity fuels higher volumes and quicker cost reductions. Velocity can also increase over time, for example, when new technological capabilities make it easier to deploy. Equally, Velocity can go down over time, for example, when other technologies replace the current one.
In all disruptive markets, the high value of technology improvements and ease of adoption generates significantly more volume, increasing the overall spend on the technology and the market size.
Putting It All Together
In mathematical terms, A simple way to extend Wright’s Law is to add factors to the learning exponent as shown in formula form below:
Cost (Vol,Val,Vel) = C0 × Vol– (⍺ +f(Val) + f(Vel)) , where C0 is the cost of the first unit. C0 is almost always unknown, but the formula can be inferred relatively from previous production volumes, costs, and time periods. The relative form of Wright’s Law is Excel format is Cost(V) = Cost(V0) * ((V / V0)^(-alpha)), where a known reference point is picked as (V(Volume) 0 and Cost(V(Volume)0.
⍺ = learning exponent. ⍺ is usually stable over time but can change a little.
f(Val) captures how “value” boosts or inhibits the rate of cost decline, which can be a positive or negative number. This value can change significantly over time when a market declines.
f(Vel) captures how “velocity” (usage intensity, ease of adoption, network effects, network effects, feedback loops, and governmental policy & regulation boosts or inhibits the rate of cost decline. Policy could accelerate AI adoption (e.g., pro‐AI legislation) or slow it (e.g., restrictions on certain uses). This value can also change over time, especially as technology declines. An example could be a government subsidy raising velocity by reducing end-user cost.
There are many mathematical ways these factors could be expressed (e.g., the three factors in the equation above could be multiplied). However, practical experience fitting the factors to previous technology lifecycles and previous experience in long-term forecasting indicates that the Volume, Value, and Velocity factors are largely independent, and an additive model best fits historical data.
This framework is a constant work in progress, and I would love to hear ideas and criticisms from forecasters and users of forecasts at davidfloyer@wikibon.org.
V3 vs. Moore’s Law
Moore’s Law described the relationship between the time to double the number of transistors on a chip and is a different way of expressing Wright’s Law. Moore’s Law ceased to operate for Intel when the volume of x86 PCs started to decline in 2012, and the smaller increases in x86 volume from server chips stabilized later that decade. The reason for the Moore’s Law failure was the because the Value delivered to end users decreased. A forecast I made in 2013 showing the replacement of x86 by Arm-based chips (see the “X86 PC Decline & Arm Mobile Domination (2013)” section below). The Arm-based chips in mobile devices continued to follow Moore’s Law and Wright’s Law!
Why 3Vs Matter for Forecasting
Explaining Exponential Growth
• New technology with high value and near‐continuous usage can see the cost drop faster and volume increase quicker than standard manufacturing learning curves suggest.
• The 3Vs methodology helps explain why smartphones rose so dramatically, why particular AI hardware is improving so quickly, and why SSDs outpaced HDDs in many use cases.
Seeing the “Next Wave” Coming
• By observing how valuable a product is (are people willing to pay a premium?) and how often or easily it’s used, you can anticipate whether it might achieve multiple volume doublings quickly.
• That signals a potentially steeper cost decline, higher volumes, and rapid displacement of older technologies.
Avoiding Under and Overestimates
• Classic models can underestimate how quickly a disruptive innovation can undercut incumbents.
• Value and Velocity amplify the volume‐driven cost decreases, causing “surprises” for those relying on historical manufacturing curves alone.
Examples from Past Forecasts
IBM CMOS vs. Hitachi Bipolar Mainframes (1995)
In 1994, IBM introduced low-cost CMOS-based to replace its previous bipolar mainframes. The performance of each CMOS processor was lower than that of bipolar processors, but the overall throughput was higher because more processors were included. Hitachi initially announced that Skyline 2 would use a new, more powerful bipolar chip.
The analysis using the above methodology concluded that the higher individual processor power was valuable to some mainframe users. However, the much lower cost of CMOS processors was a more important value factor to most users. In addition, the CMOS mainframes were more straightforward to install and maintain. The analysis showed that Hitachi would sell far fewer processors at much higher costs with only one feature of additional value. The published forecast in late 1995 stated that Hitachi was unlikely to ship Skyline 2, because the cost of Skyline manufacture would be 2-3 times the cost of IBM CMOS. A few months later, Hitachi canceled Skyline 2, closed the bipolar chip foundry, and withdrew from the IBM-compatible mainframe market. All the other forecasts predicted that Hitachi would announce and ship Skyline in volumes.
X86 PC Decline & Arm Mobile Domination (2013):
While I overshot the exact timing, the fundamental logic was that Arm‐based chips offered substantial value (low power, better instruction set, performance gains) and near‐continuous velocity (people on phones and texting all day), thus eventually displacing traditional x86 in many use cases. Today, Apple PCs with parallel-computing designed large chips with integrated CPUs, NPUs, a GPU, and accelerators sharing SRAM outperform x86 systems by a large margin. Microsoft has moved its developmental focus from x86 to Arm-based processors.
SSD vs. HDD
NAND flash has huge consumer and enterprise demand, raising volume quickly. Its “Value” (greater speed, greater durability, & lower space) plus “Velocity” (no adoption cost, used extensively in every smartphone, most PCs, etc.) drove more R&D dollars into Flash, leading to a steep cost decline and broader adoption. For example, the value of HDD on mobile devices and PCs has gone negative, and the data has moved to cloud services. The HDD vendors were faced with declining volumes and, as a result, have not been able to reduce the cost of new technologies, such as HAMR, to be below the cost of existing HDD technologies. Although cheaper now, HDD will join floppy disks in the Mountain View Computer History Museum before the end of the decade.
Our AI Forecasting Focus
We have already produced a forecast of AI datacenter vs. traditional datacenter spend. We will be tackling the following list of forecasts in 2025.
• Hyperscale Cloud AI Spend
• Enterprise AI Datacenter Spend
• AI Edge Spend
• Automated Transport AI Spend
• AI Classic Robotics Spend
• AI Humanoid Robotics Spend
• AI Military Spend.
Current Forecasts
Worldwide Datacenter Spend on Traditional Datacenter platform vs. AI Platforms
Figure 1 below shows total data center spending.
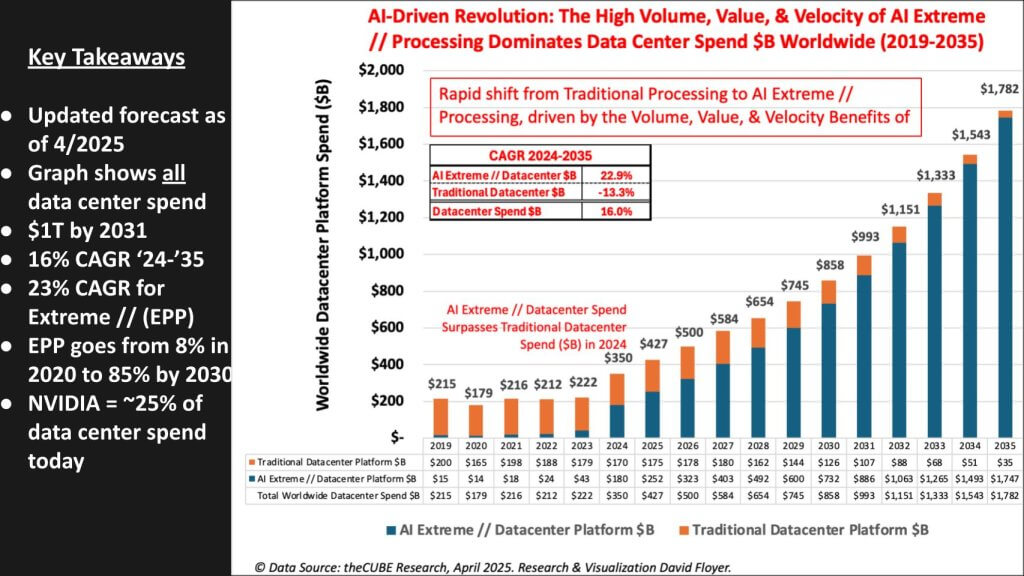
Worldwide Datacenter Spend on Traditional Datacenter platform vs. AI Platforms
The AI datacenter spend ($B) shown in Figures 1 and 2 above are very different from the traditional datacenters, which are built on x86 processors, traditional hybrid storage arrays, and traditional communication.
The AI datacenters are built to manage Tokens for creating AI Models and inference services using these models. and in this research we have covered the impetus for a new forecasting method (Volume, Value, Velocity). It helps to explain fast disruptions and explain the end-of-life behavior of
Figure 2 below shows worldwide AI datacenter spend ($B) split into Cloud AI Datacenter and Enterprise Datacenter AI.
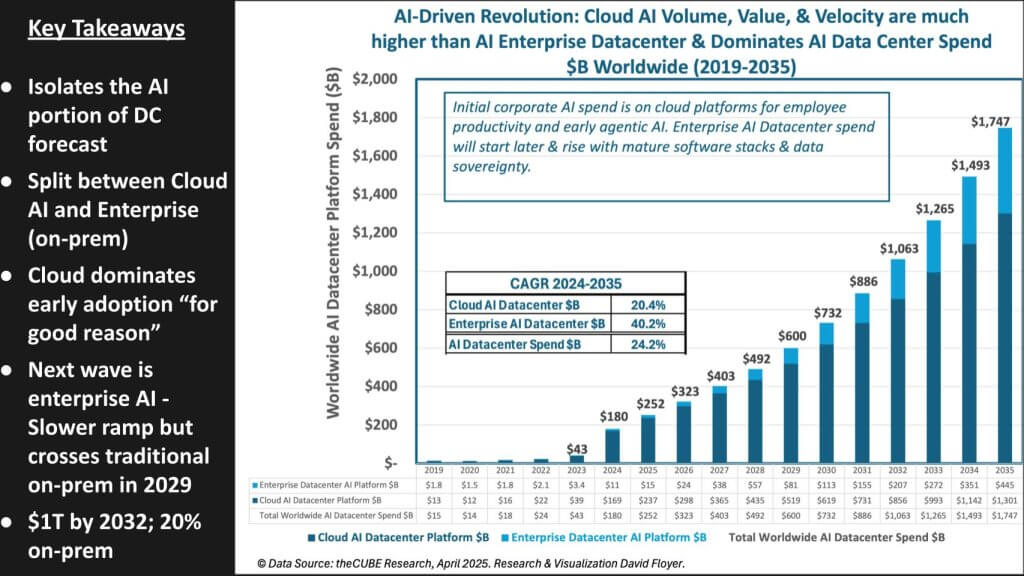
Conclusion & Recommendations
In this research we have covered the impetus for a new forecasting method (Volume, Value, Velocity). It helps to explain fast disruptions and explain the end-of-life behavior of technologies. It is also uniquely relevant for AI because of the enormous value of AI models and inference and its extremely rapid adoption. The three V3 gives a framework for forecasting different rates of adoption for different market sectors.
Volume, Value, & Velocity have been my framework for a long time and have helped me interpret where technologies like AI, PCs, or SSDs are headed and when and why they can outrun conventional forecasts. We will happily run bespoke forecasts for vendors, enterprises, and government. If you integrate these 3Vs into a Wright’s Law approach, you’ll better capture the proper pace at which disruptive innovations transform markets—and see the tipping points long before they happen.
*Note: My Forecasting 3Vs are different from Gartner’s “3Vs” of Big Data, Volume, Velocity, and Variety”, which represent the challenges of managing the sheer amount, speed, and diversity of big data. They have volume in common, but Gartner’s volume refers to the amount of data to be managed, and my forecasting volume refers to the volume of a manufactured product.
Appendix A: List of Common Forecasting Methods
1. ARIMA (Auto-Regressive Integrated Moving Average)
A time‐series technique using past data patterns (trends, autocorrelations) to project future values. Typically used for short to medium‐term forecasts where historical trends are assumed to continue.
2. Bass Diffusion Model
Explains how innovations spread through a population by splitting adopters into “innovators” and “imitators.” Widely used for new consumer products, relying on parameters for market size, innovation rate, and imitation rate.
3. Delphi Method
A structured way of gathering expert opinions through multiple rounds, with anonymized feedback, to reach a reasoned consensus on future developments.
4. Exponential Smoothing
A basic time‐series family (e.g., Holt‐Winters) that weights recent data more heavily and is effective for stable patterns with possible trends or seasonality. Less suited to major disruptive shifts.
5. Extended Wright’s Law & Domain‐Specific Variants
Ties cost declines to cumulative production (standard Wright’s) but include specialized forms like Henderson’s Law (solar PV) and Moore’s Law (semiconductors), adapted to a particular industry’s financial empirics.
6. Scenario Planning
Builds multiple plausible futures based on different assumptions (economic, technological, regulatory) rather than producing a single forecast and often used in high‐uncertainty environments to inform strategic decisions.
7. S‐Curve / Logistic Growth Models
Capture how adoption starts slowly, accelerates, and plateaus as the market saturates (e.g., logistic or Gompertz curves). Helpful in tracking how a technology’s market penetration evolves.
8. System Dynamics & Agent‐Based Models
Complex simulation approaches that incorporate feedback loops, interactions, and network effects. Suitable for understanding multifaceted systems but can be data‐ and assumption‐intensive.
9. Volume, Value & Velocity Extended Wright’s Law
Augments Wright’s Law by explicitly incorporating Value (benefits that users find) and Velocity (usage intensity, ease of adoption, network affects, feedback loops, governmental policy and regulation), each adding to the learning exponent. It is particularly suited for disruptive products where strong demand and heavy usage accelerate cost declines.
Appendix B: A Few Practical Forecasting Techniques
• Ranges for Value and Velocity: You should define f(Value) so that f(Value)=0 means “neutral,” i.e. baseline learning. A small positive number (like 0.10) might be moderate added value, whereas 0.30–0.50 signals a game‐changing leap in user benefits. You should define f(Velocity) the same way).
• Gauge Demand & Usage: Look for how compelling the new product is and how often it’s used. High daily use can often accelerate feedback loops.
• Track Ecosystem & Ease of Adoption: Examine network affects, feedback loops, If setup or integration is frictionless, expect volume doubling at a rapid clip, which can yield bigger cost drops and higher volumes.
• Take a Hard Look at Adoption: Examine any difficulties in implementation such as competitive moats and governmental policy and regulations.
• Model the previous technology market trajectory: if the older technology’s value proposition weakens, or if its usage velocity diminishes, it might lose momentum fast and increase adoption of new technologies.
Additional Information
The more tokens used,the more AI system resources used, the higher the quality of output and the greater the value to the user. The value to business is the increase in productivity and decrease in headcount. The table below shows the range of tokens used and the potential for extending the depth and quality of answers and the increase in Tokens required.
For example: Using Deep Research on ChatGPT 4o Pro can take 10-15 minutes to complete a research task.
The table below shows some incomplete data about the volume of AI request from the leading providers of AI Cloud.
We appreciate and welcome any feedback to help us fine tune and improve the methodology. Thanks for reading.