Premise
Mainstream enterprises need to view ML and AI technology as a hierarchy of abstraction layers. That’s the best way to prioritize how and where they can source this technology. It’s possible to work all the way down at the “metal,” where researchers and elite data scientists build new algorithms. At the other extreme, it’s possible to work with semi-finished applications that developers can simply customize with their own data.
An extreme shortage of data scientists exists outside tech-centric companies (see figure 1). In order for mainstream CIOs to avoid having their AI/ML pilots bog down – or unnecessarily fail outright – CIOs and line of business (LOB) executives need to inventory their in-house skills. With an understanding of limited skills availability, executives can establish concrete strategies for acquiring AI/ML knowledge into their organizations in some other, non-labor form. Effectively, mainstream enterprises have to leverage as much “packaged” external expertise as possible. This expertise includes developer-ready models with APIs as well as packaged apps with AI and ML built-in and pre-trained (see the first two levels in figure 2):
- Leverage developer-ready, pre-trained models for incorporation into applications. Enterprises with limited data science expertise can use API-level services packaged for traditional developers from the public cloud vendors. Vendors of these services have made the most progress with what they call cognitive services.
- Buy packaged applications with built-in ML and AI models. Virtually lost in all the hype around ML and AI is the availability of packaged applications where the ISV has embedded just about all the necessary data science. ML and AI are a seamless part of a bigger solution where the analytics can anticipate and influence user interactions or inform or automate business transactions.

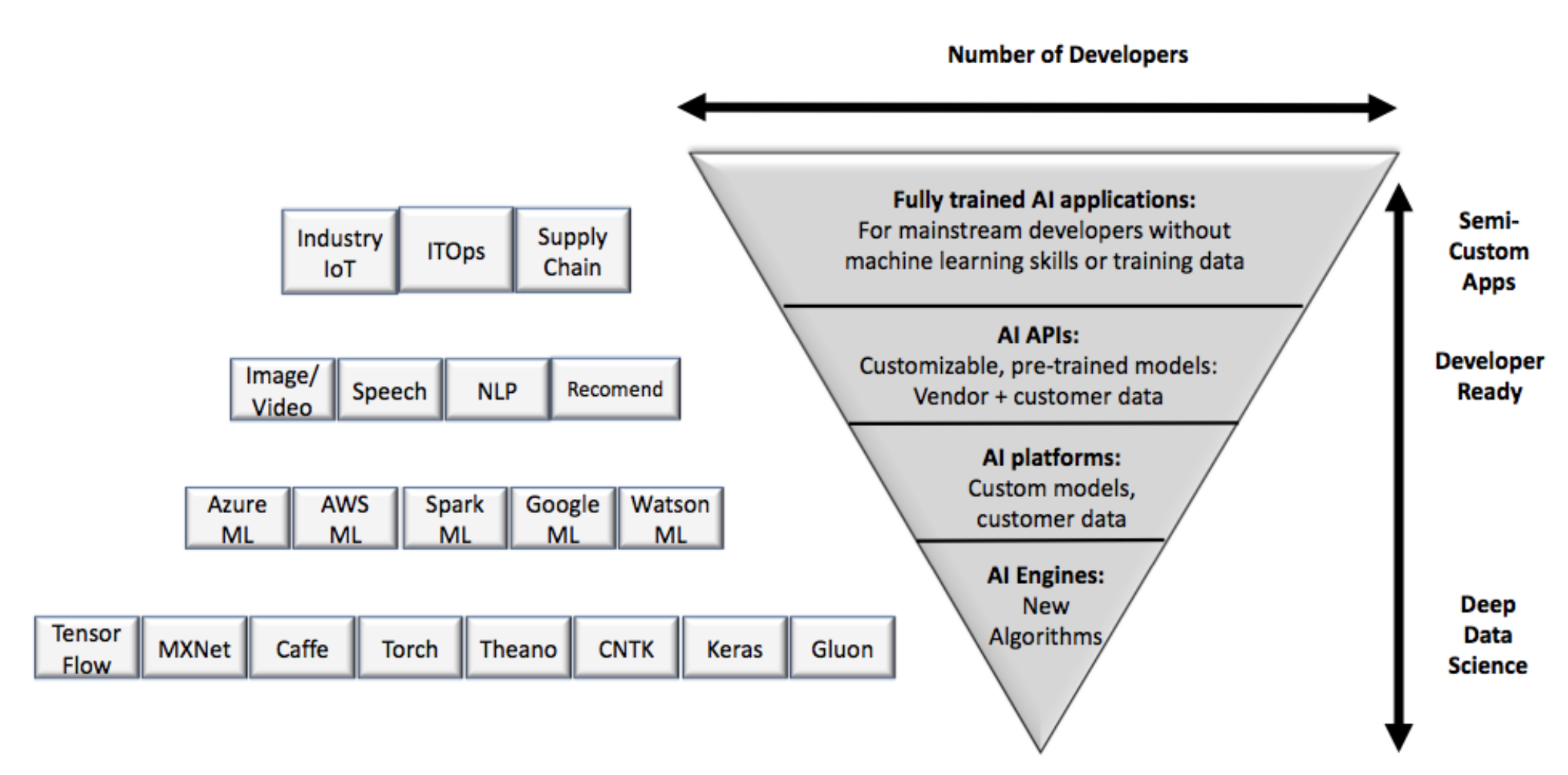
Leverage Developer-Ready, Pre-trained Models For Incorporation Into Applications.
Developers with only moderate knowledge of AI/ML can take advantage of some of the most advanced pre-trained models delivered as online services. Some of the big public cloud vendors have repurposed the immense amounts of consumer data they’ve gathered in order to train services that then can be made available to businesses. The data collected for Google’s Assistant feeds back to improvements in speech recognition, natural language understanding, and speech synthesis. Google’s Photos, YouTube, Translate, and Knowledge Graph also feedback into image recognition and object classification; video search; translations between language pairs that weren’t manually paired; and a semantic classification of people, places, events, and things, respectively. These services and similar ones from other cloud vendors generally fall into the category of cognitive services that support next-generation user interfaces. Pre-trained models make AI/ML accessible to far more of the world’s 20M developers.
Some enterprises may need to customize the standard models. For example, an apparel company might want a cloud image recognition and classification model to differentiate between different articles of clothing more precisely than the standard model. There are two ways to improve the model’s ability to discriminate. Either developer will have to (1) know more about how to customize models by training them with additional, labeled data or (2) a third party application developer would put a UI over the online service to make customization accessible to non-developers. Similarly, cloud services offer chatbots with only general training. B2C enterprises will need developers with some degree of understanding of how natural language understanding models work. Developers will need to add new intents, entities, and contexts to support conversations around new topics. Finally, the online service provider, commercial ISVs or the ultimate enterprise customer will have to work out IP rights around these customizations.
Buy Packaged Applications With Built-in ML And AI Models.
Virtually lost in all the hype around AI/ML is the increasing availability of packaged applications where the ISV has embedded just about all the necessary data science tools and models. AI/ML are a seamless part of a bigger solution where the analytics can anticipate and influence user interactions or inform or automate business transactions. Prototypical applications of packaged AI/ML applications include cybersecurity such as User Behavior Analytics; applications that leverage legacy ERP and CRM packages such as lead scoring and nurturing, demand forecasting, replenishment optimization, and price optimization; ad targeting; and a rapidly increasing number of IT operations management applications.
New entrants should have several years head start because incumbent application developers have different technical skills, different go to market motions and different pricing models. Legacy applications feature record keeping for standardized business processes. With AI/ML applications, by contrast, data informs and shapes models which, in turn, inform decisions. On the go-to-market side, rather than selling to CIOs, AI/ML applications affect business outcomes where the LOB executive is the decision maker. In addition, industry-specific solutions may cross company boundaries and an ISV’s customer may turn out to be a channel partner to reach the rest of the supply chain. Finally, successful deployment will be less about absorbing new technology and more about accommodating changes to the decision-making process in the parts of the organization that the applications touch.
Action Item
Mainstream businesses are aggressively pursuing AI/ML technologies. But the extreme skills shortage outside tech-centric companies is impeding meaningful progress. CIOs and LOB executives need to be prudent. Executives must tap what’s available in API-accessible cloud services as well as enterprise apps with embedded machine-learning. These sourcing strategies must emphasize protection of existing and future IP, while keeping options for reinstitutionalizing ML/AI work as experience and talent availability grows.