Premise
The Big Data marketplace is poised to grow 20% CAGR over the next decade, but will rise and fall in response to customer adoption of three distinct application patterns. Simplicity is emerging as a central theme: The Big Data ecosystem has to make it easier for enterprises to deploy and manage increasingly sophisticated data preparation and analysis chains.
Wikibon’s forecast for big data application patterns foresees strong market growth through the mid-2020s, but also a sequence of technology transformations intended to simplify administration and accelerate developer productivity. Hadoop will likely play a central role, but we expect complementary and substitute technology to improve data preparation and analysis tool chains and to streamline the analytic data pipeline. The business potential of big data remains enormous, but future prospects are dependent on:
- Getting past hit or miss: simplification and integration with application portfolios. Wikibon forecasts the big data market will grow at 20% CAGR from 2014 to 2026, but growth will reflect the evolution of three distinct application patterns (see Figure 1). Big data today features hit or miss returns from solving big problems with tools that are difficult to use and resist integration with each other as well as into broader application portfolios: In other words, it remains an immature technology domain with significant, but uncertain, potential.
- The three critical milestones to maturity. Achieving our growth estimates depends on 3 key innovations: 1) simplifying the tool chains data scientists and developers use to craft their predictive models, and 2) integrate the analytic engines into a pipeline and the disparate storage products into a platform the way AWS, Azure, and Google do with their native services, and 3) apply machine learning to the data scientist and developer tool chains to automate more of what those individuals must do today.
- Following a path to proficiency that builds on previous success. Our clients are discovering successive generations of big data technology will grow on each other, each generation featuring a particular pattern that emerges to solve a class of big business problems. Companies that master these patterns early will be the winners, either by selling the products and services that facilitate these patterns or advancing the state of the art in their applications to increasingly complex and differentiating business opportunities.
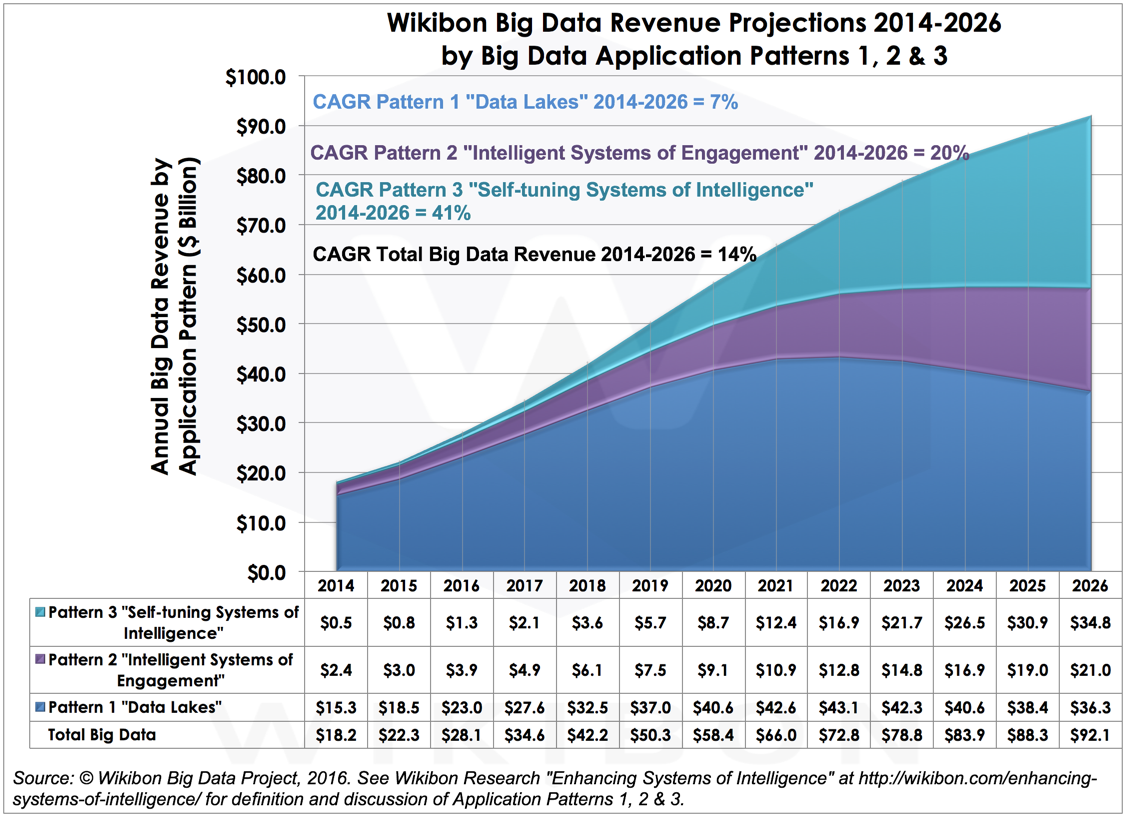
Source: Wikibon Big Data Research 2016
2016-2019: Refining Data Lakes
Data lakes are pre-requisite infrastructure for unlocking answers to previously intractable problems such as application infrastructure management, fraud prevention, and recommendation engines. In 2016, we forecast growth of 25% for this application class. Over the next few years, early-adopters will drive data lake technology hard by integrating subsets of data lake output into a business’s operational systems. However, Wikibon believes data lake growth will taper to 18% by 2018. Why? Because Hadoop’s open source success has a complexity downside. Hadoop is not a single product. Instead, it’s an ecosystem comprised of several dozen products, each generally utilizing unique approaches to handling sign-ons, permissions, intrusions, failures, and even administrative consoles.
For data lake market and productivity growth to remain high, the big data ecosystem must start to coalesce around a common set of integration approaches, especially across data preparation and analysis tool chains (see Table 1). Improving auditability and data provenance is at the top of the list. There’s no one answer to these issues. A multitude of parties must improve how they work together to drive the ecosystem forward, including open source project leaders, commercial tool providers, big data service companies, and most importantly enterprise customers.

Source: Wikibon Big Data Research 2016
2020-2022: Maturing Intelligent Systems of Engagement
Intelligent systems of engagement (SoE) unlock measurably more business value than applications based solely on data lakes. Data lakes don’t disappear, though. Instead, data lakes are evolving into platforms that infuse more complex SoE applications with intelligence. SoE integrate recommended actions from data lakes with customers interacting through digital experiences supported by systems of record. Wikibon forecasts category growth at 21% in 2020 with share of total spend then rising steadily from 16% to 23% in 2026. By this forecast period, Spark and more unified storage should simplify the traditional task of herding the zoo of products in the Hadoop ecosystem. Mainstream maturity and adoption should materialize for unified analytic engines such as Spark. Spark today is already starting to perform many functions that previously required individual engines such as streaming, SQL, machine learning, statistics, geospatial, and graph processing. At the same time, simplification should come to storage, with greater unification between the file system, a multi-model database, and a stream processor, like MapR’s version of Hadoop.
Once greater unification comes to the analytic engines and storage platform, doers will have to push both the design-time and runtime data prep and analysis tool chains deeper into production applications. The design-time process that spans data prep to machine learning to model deployment has to operate as a repeatable, production process. That process will require custom integration. For the run-time tool chain, doers need to reinvent traditional ETL that connects applications point to point into a new design where applications connect through a central hub of low latency, continuously streaming data.
2022 And Beyond: Self-Tuning Systems of Intelligence Drive Growth Longer Term
Visionary customers are starting to experiment with using machine learning to enable systems to “self-tune,” maintaining or even improving the predictive models operating in production applications. Today, self-tuning systems of intelligence account for 5% of big data spending, but the percentage is growing, and growing rapidly. Next year, Wikibon estimates that growth rates for these systems will be 68%. While systems based on this pattern will significantly expand big data returns, they build so heavily on the prior two that they don’t actually consume the majority of total spend, reaching 38% in 2026.
In addition to improving facilities for integrating advanced analytics and production applications, self-tuning systems will support other crucial big data innovations. At design-time, machine learning needs to recommend data prep algorithms as well as which additional data elements will improve the models. At run-time, machine learning needs to recommend improved models and which candidate models are a better fit. If the Hadoop ecosystem remains too complex, doers should look to Azure, AWS, and Google waiting in the wings with their native services. While they may not have all the specialized capabilities of the Hadoop ecosystem, their native services are increasingly designed, built, integrated, tested, delivered, and operated as a unit. Their clear direction is to simplify and democratize the process of building ever more capable self-tuning systems of intelligence.
Action Item
An increasing share of the profit pool will accrue to vendors who can deliver an ever more integrated stack. Many vendors, especially those with open source products, deliver and capture value by helping customers run their products, whether on-premise or in the cloud. But it is the interactions between products that were not designed together, including many in the Hadoop ecosystem, that create the most complexity. A greater breadth of integration may compromise leading-edge functionality. But it offers greater simplicity for admins and developers, a trade-off mainstream customers generally favor.