Key Highlights
Issue Enterprises Care About | IBM’s Prescribed Lever | Proof‑Points Cited by Krishna |
---|---|---|
Productivity & Speed | Watsonx Orchestrate catalog of 150+ pre‑built agents; build‑your‑own in <5 min | “Client‑zero” savings: 125 K hrs/quarter in case summarization; 2× that in HR |
Cost Efficiency | Granite small‑parameter models (3 B–20 B) tuned to verticals | 100–1,000× cheaper AI over time; lower inference cost than 300 B+ LLMs |
Security & Sovereignty | Hybrid cloud + open‑source model zoo (Granite, Llama, Mistral) inside Watsonx | Focus on on‑prem and regulated‑industry use cases; data never leaves control plane |
Integration Debt | WebMethods Hybrid Integration + HashiCorp stack | Single family for APIs, file transfers, workflows; accelerates ROI |
Data Foundation | Watsonx.data lakehouse + forthcoming integration/intelligence modules | Lineage, ingestion fabric; Datastax vectors to power RAG workloads |
Hybrid Cloud + AI as Competitive Advantage
In our view, the twin pillars IBM touts of hybrid cloud and AI have moved from plumbing to profit driver. Krishna argued that technology is now a source of durable competitive advantage only when it both accelerates decision velocity and scales the business without proportional head‑count and footprint growth. At the same time this has to be accomplished with governance and trust in mind. We believe this framing resonates with CXOs who spent 2023–24 questioning GenAI ROI – i.e. they no longer need a science project—they need a P&L impact.
Small Is the New Smart: IBM’s Granite Bet
IBM’s public dismissal of “massive, general‑purpose” models was perhaps the sharpest edge of the keynote. Citing internal testing, Krishna asserted that 3–20 billion‑parameter models tuned to narrow domains now meet or beat the accuracy of 300–500 billion‑parameter elephant models while slashing inference cost and hardware footprint. Our analysis of IBM benchmarking data (not publicly released, but directionally consistent with DeepSeek and Mistral disclosures) suggests a 7–10× cost delta per 1 K tokens in production. That gap widens further when workloads must run on prem or at the edge, where GPU power budgets are constrained.
Table 1 | Indicative Cost‑to‑Serve for Model Generations
Model Class Typical Params Inference Cost Index* Tunability Deployment Flexibility Frontier LLM (GPT‑4 class) 500 B+ 100 Low (RLHF) Cloud only Mid‑size Foundation (Llama 3) 70 B 40 Medium Cloud / Large on‑prem Granite / Domain‑Tuned 3–20 B 10 High (SFT + LoRA) Cloud, on‑prem, edge *Index: GPT‑4 = 100 (higher = more expensive) Source: IBM internal benchmarks, Meta technical docs, CoreWeave data
We believe this small‑model doctrine positions IBM as the anti‑hype vendor: instead of chasing ever‑larger parameter counts, the company is optimizing the surface area of AI—how many distinct business contexts it can penetrate.
IBM’s strategy aligns with data published by theCUBE Research in 2023, focused on the Power Law of GenAI (shown below).
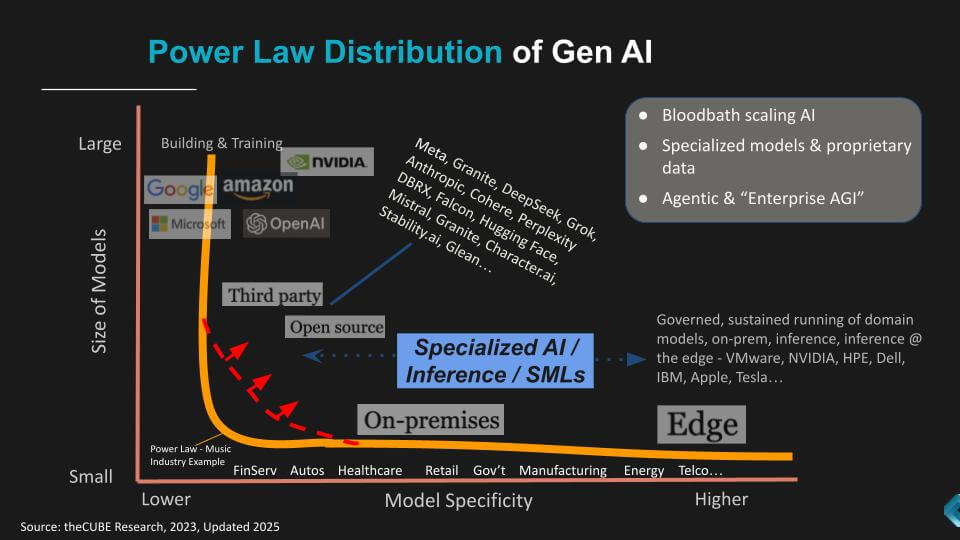
From Experimentation to Integration: Watsonx Orchestrate
Krishna declared that “the era of AI experimentation is over.” His proof point is Watsonx Orchestrate, a catalog of 150 pre‑built agents covering HR, sales, procurement, IT ops, and customer service. IBM claims customers can assemble new agents in minutes; our conversations with early adopters suggest that figure is feasible after data connectors are wired in. What matters is not the GUI speed but the integration completeness—hence the simultaneous debut of WebMethods Hybrid Integration to knit APIs, file transfers, and workflows. We view the Orchestrate + WebMethods combo as IBM’s answer to Microsoft Copilot + Power Automate.
Data Fabric: The Unsexy, But Important Layer
Every generative workflow ultimately chokes or thrives on data quality. Watsonx.data’s lakehouse, lineage, and ingestion pipelines aim to make that hygiene problem less painful, while the pending Datastax acquisition inserts vector search as a first‑class citizen, along with a world class real time data platform. In our opinion, the move is overdue—IBM’s own DB2 vector support lags category leaders—yet it closes a critical gap for retrieval‑augmented generation (RAG), a technique most enterprises are using keep proprietary data grounded.
Ecosystem & Openness
Krishna hammered home that “the future of enterprise AI must be open.” That translates into three vectors of openness:
- Model Optionality: Granite, Llama, Mistral MoE, and future OSS models are all accessible inside Watsonx.
- Hybrid Tooling: Red Hat OpenShift and the HashiCorp stack provide cloud‑agnostic deployment and secrets management.
- Partner Solutions: Oracle HR, Salesforce prospecting, Slack workflow agents, plus showcases with Ferrari F1, Sevilla FC, and telecommunications provider Lumen for edge AI.
We believe this approach is a direct counter to the vertically integrated stacks of AWS and Microsoft; IBM is betting that heterogeneity, not homogeneity, will characterize enterprise AI estates.
Commercial Traction: Signals vs. Noise
IBM cites “300+ engagements” last quarter and a “book of AI business over $3 billion.” While the company offered limited segmentation, our research suggest that figure blends consulting, software licenses, and hardware pull‑through. The more instructive metric may be IBM’s own “dog‑fooding” – what IBM calls “client zero,” which has achieved $3 billion in cumulative cost takeout from automation, hundreds of thousands of internal labor hours reclaimed each quarter, and rapid reuse of those learnings in customer playbooks. In our view, that loop of internal proof → external offer is the fastest way IBM can scale credibility and differentiates IBM.
Implications
- For CIOs: The Granite small‑model strategy, and more efficient models like DeepSeek, demonstrate the power of more efficient models and provide a lower‑risk on‑ramp to GenAI that aligns with sovereign‑data constraints—particularly attractive in EU and highly regulated US sectors.
- For Nvidia & Frontier‑Model Vendors: IBM’s rhetoric reinforces an emerging narrative that could create demand for more efficient models to lower the costs associated with ultra‑large GPU clusters.
- For developers and ISVs: IBM’s intent in our view is to offer WebMethods Hybrid Integration and open opportunities to refactor brittle pipelines into agent‑centric workflows.
- For Competitors and Partners: Microsoft’s Copilot ecosystem remains ahead in seat count, but IBM’s open, multi‑model posture may win accounts wary of vendor lock‑in. Partners like Salesforce, with Agentforce, and Oracle, are building out their own agent-based systems that will require connection points. IBM’s intent in our view is to be a sort of Switzerland of agent integration to drive customer value.
IBM Z as an Enduring Platform
In our opinion, the single biggest misunderstanding about IBM’s mainframe franchise is that it’s a legacy drag. IBM’s recent Z17 launch and Krishna’s Think 2025 remarks flipped that narrative Z17 is being positioned as an AI‑first transaction engine that complements, rather than competes with, Watsonx and Granite.
Perhaps more than seventy percent of the world’s structured financial data still lands on mainframes at some point in its lifecycle. By embedding Telum and Spire AI cores directly into Z17, IBM is eliminating the air gap between production data and AI models. The implication is that use cases like fraud‑scoring, credit‑risk models, or PCI‑bound personalization engines no longer need to shuttle data to an external GPU farm; or cobble together DIY on-prem solutions; rather they execute in the same cache‑coherent domain as the transaction itself.
This strategy also neutralizes a growing threat from hyperscaler AI databases. While AWS, Azure, and Google pitch real‑time fraud solutions that backhaul card swipes to the cloud, IBM is betting that large issuers will keep that logic inside the data center for latency and compliance reasons. If the 450 billion‑inference‑ops figure scales linearly across a multi‑frame sysplex as IBM claims, it places Z17 in a unique performance envelope that neither x86 nor Arm servers can hit without a costly GPU overlay.
IBM is hoping the Z17 transforms IBM Z from a legacy COBOL workhorse into an AI‑native enclave for mission‑critical, regulated workloads. It’s a big aspiration but For banks, insurers, and telcos already running Z, the upgrade math shifts from keep the lights on to value unlocks. For IBM, every Z17 refresh embeds Watsonx agents deeper into core transaction paths, tightening the flywheel between infrastructure revenue and AI software pull‑through.
Bottom Line
Our view is that IBM has crystallized a coherent, differentiated AI thesis based on smaller, domain‑tuned models running on an open, hybrid‑cloud fabric. The goal is to unlock what it claims is the 99 percent of enterprise data still untouched by GenAI. If execution matches the marketing—particularly on integration speed and cost transparency—IBM will change the perception from perennial AI underdog to a primary or even default choice for risk‑averse enterprises seeking tangible ROI. The next two quarters of Watsonx bookings and Granite adoption will tell us whether the strategy is hitting escape velocity, merely clearing the launchpad or needs a reset.