Contributing Analysts: David Vellante and George Gilbert
Premise
IT management practices must evolve to bring mature transaction systems and contemporary analytics capabilities together. The phrase “contemporary analytics” refers to Predictive Analytics (PA) and modern Decision Management (DM) systems that, when leveraged with existing Systems of Record (SoR), form a coherent, agile and new operating model we refer to as Systems of Intelligence (SoI). Systems of Intelligence, we believe, will form the backbone of true data-driven organizations and confer significant competitive advantage to organizations that master the processes, skills and technical acumen necessary to bring these systems to life. The following parameters are fundamental to this capability:
- Existing systems of record (e.g. transaction systems) must be leveraged and evolved by applying modern analytics disciplines, processes and technologies to extend such systems;
- These systems must have the ability to handle multiple, disparate data sources and blend them into a coherent data model;
- Analytics must be operationalized and embedded within systems of record such that both humans and machines can take action;
- Infrastructure choices are relevant and emerging systems of intelligence (e.g. those leveraging transaction systems) will likely run on “True” private cloud infrastructure that can support the agility requirements of SoI.
Importantly, Systems of Intelligence are not a bespoke analytics capability, managed by data scientists and lines of business. Rather they represent a fundamental transformation of existing Systems of Record, applying agile methods, modern tooling and automation to support data-driven cultures and affect business outcomes in near real time.
Primer
There is much discussion around the concept of “Bimodal IT,” 1 a concept put forth by Gartner that refers to separate yet coherent management modes for information technology systems. Mode 1 is sequential and traditional, emphasizing stability and risk mitigation while Mode 2 focuses on agility, experimentation and speed. Systems of Record (SoR) are presumably included in Mode 1 where accuracy is fundamental while new areas such as predictive analytics and modern decision management are targeted for Mode 2.
Wikibon’s research shows the return on investment (ROI) from PA and modern DM come from implementing automated decision-making integrated inline with the systems of record (SoR). The term inline means the data on which the automated decisions are made is processed in real time at the same time as the transactional data is processed by the systems of record. We refer to this capability as Systems of Intelligence (SoI).
To achieve maximum ROI with SoI, we believe practitioners must apply a Mode 2-like mindset to the whole. Specifically, our research shows that applying sequential and traditional methods to the SoR component of SoI will create a weak link and limit results. Rather we believe that SoR staff must work hand-in-hand with data teams using a similar operating model to create systems of Intelligence. To be clear, we don’t believe this approach will sacrifice the compliance, security and governance edicts of the organization. Rather we are prescribing an approach that preserves the data quality aspects of SoR while at the same time increasing agility.
The ROI of integrating decision automation and predictive analytics as part of the SoR is an order of magnitude higher than traditional decision support systems (DSS) which really focused on making a handful of executives and professionals smarter. Wikibon uses the terminology Systems of Intelligence (SoI) to describe the integration of systems of record, predictive analytics and modern decision management.
There are two broad alternative strategies we’ve seen to successfully implement systems of intelligence:
- Add the decision management component to create a systems of intelligence platform within existing Systems of record; or
- Move all or part of the systems of record to be part of the modern DM systems.
Wikibon’s research shows the ROI of the former (creating SoI from the existing SoR) is almost always much greater with lower risk than converting the SoR onto another platform. The time to realize full value from systems of intelligence is between 2 to 5 years faster when leveraging the existing SoR.
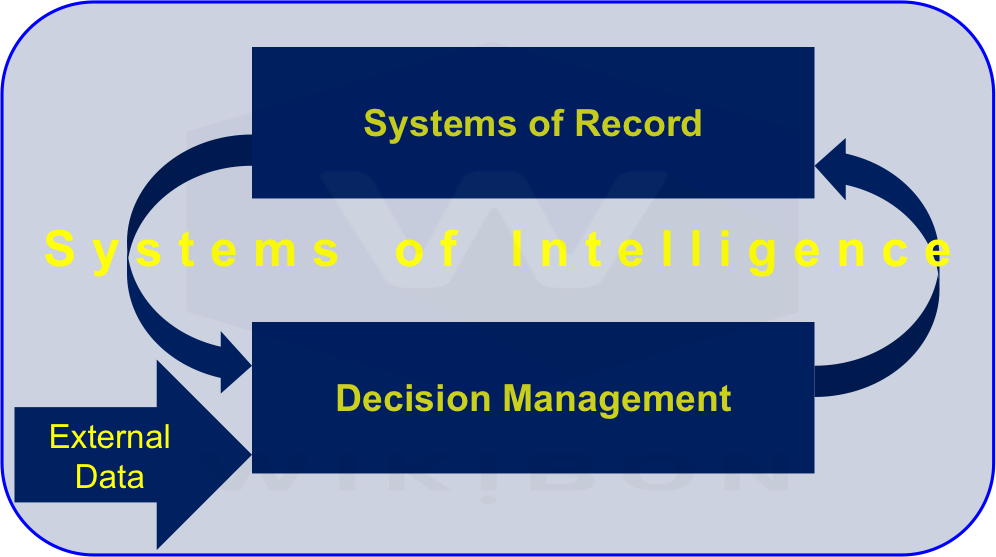
Source: © Wikibon, 2016
Wikibon’s research indicates that for SoI to be successful organizations must make significant investments in both PA and DM as well as the existing transaction SoR. Our research shows that successful SoR teams lowered the cycle time and cost of making incremental change, and operated with the same agility as the analytic team. Figure 1 shows a simplified diagram for Systems of Intelligence, which Wikibon believes should be the main focus of C-level executives and senior management.
Key Findings
- The value of leveraging transaction systems (SoR) to build a data-driven capability primarily comes from organizational productivity, not IT savings…
- Our models show that within five years, if implemented correctly, a 20,000 person organization following our prescribed recipe can do the same work with 3,000 fewer employees;
- Viewed more positively, those 3,000 employees, if retained, will generate an incremental revenue of $1B annually within five years;
- Organizations can choose to reduce headcount to save money, maintain headcount and avoid FTE costs or add headcount with greater productivity (e.g. revenue/employee) metrics;
- Revenue per employee will increase 35% in that timeframe;
- There is no free lunch – C-level executives that want to achieve such results should plan to increase IT spending as a percent of revenue. In our models, a firm with a $200M IT budget must increase IT spending by 12.5% annually (from 4% to 4.5% of revenue) in order to achieve such results. While there may be ways to cut elsewhere, we believe leading firms are increasing, not decreasing investments in IT.
Methodology and Data Sources
As part of this research, Wikibon developed a financial/economic model based on the Standard Wikibon Business Model, adjusted for a larger organization. The model assumes that the recommendations given in the “10 Strategic Steps to Implement Systems of Intelligence” section below are followed. The economic model was developed using the following inputs based on primary and secondary data sources:
- In-depth interviews with several dozen mid-to-large organizations implementing Systems of Intelligence in varying degrees of maturity to understand best practice and achievable results;
- We used these interviews to measure the people, process and technical adoption factors related to merging transactional and modern analytic systems;
- Organizational benchmark data developed over a ten-year period based on more than 300 interviews with leading organizations globally;
- Census data, department of commerce statistics and other publicly available data sources;
- Interviews with technology experts to determine the level of capability for existing and future technologies.
This firm level and macroeconomic data was used to develop “rules-of-thumb” assumptions for the economic model to include factors including:
- Average productivity per employee within various industries;
- Revenue per employee for public companies;
- IT spending as a percent of revenue by industry;
- FTE headcount for IT staff and corresponding average salaries as compared to regular FTEs;
- Percent of employees’ time spent actively using IT systems (applications) versus performing other tasks;
- The importance of IT over time as a contributor to revenue and productivity;
- Project ROI, IRR, NPV and breakeven period for a range of projects involving varying degrees of technology contribution with a specific emphasis on blending analytic and transaction systems.
Figure 2 below is a summary of the financial findings. The graphic shows the financial impact of building a modern analytics capability, in-line within the existing Systems of Intelligence framework. The bars show cumulative benefit over the five year period with key metrics called out. As indicated, with respect to headcount, organizations can choose to cut jobs and drive productivity up significantly, maintain the same FTE level and avoid new hires or aggressively hire and keep revenue per employee roughly flat but drive still drive incremental revenue. Note: In all three scenarios, revenue for the organization increases as a function of building out a data-driven culture.
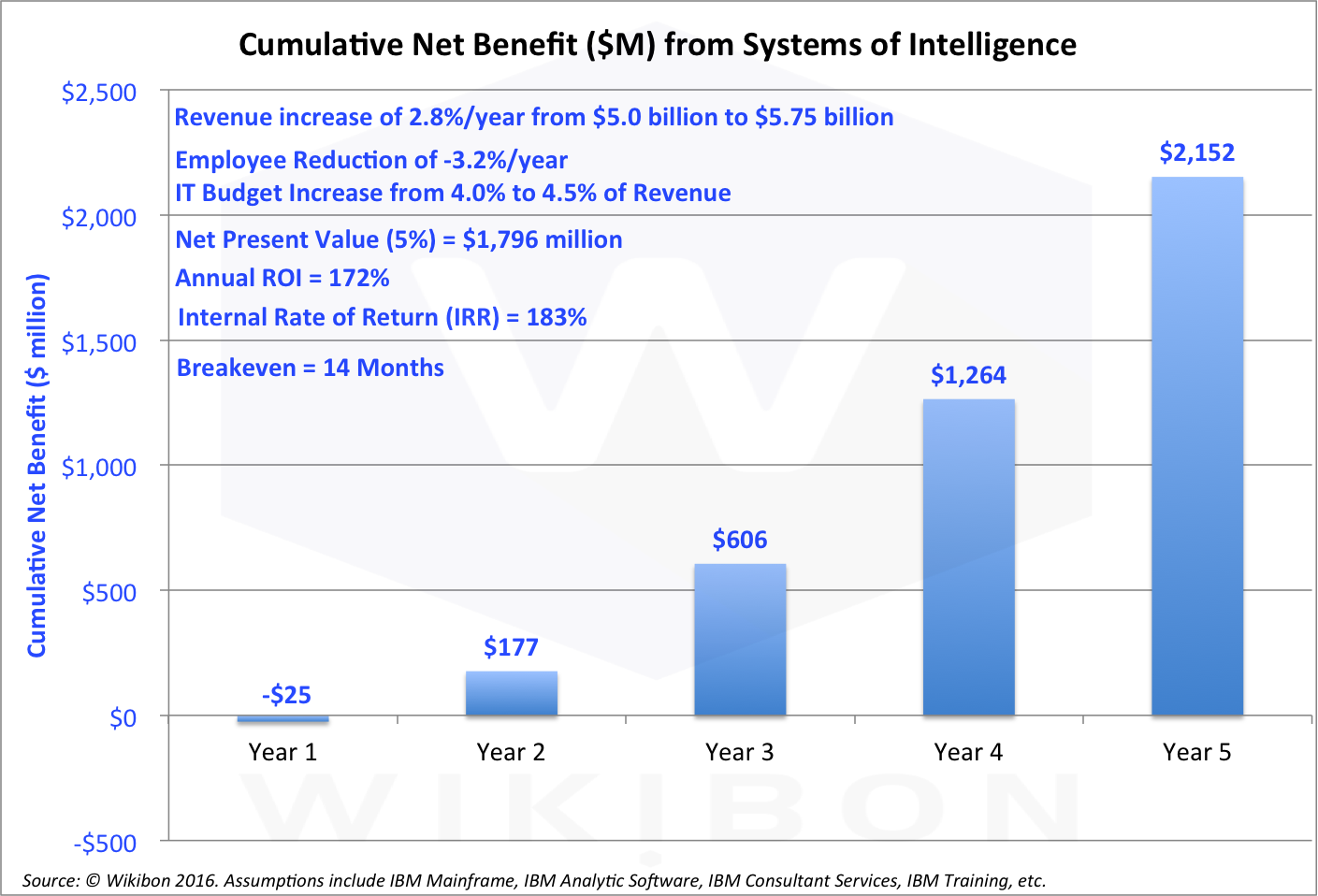
Source: © Wikibon, 2016. See Table 1 and Table 2 in the Footnotes below for details of the assumptions and calculations. See section “Business Case for Systems of Intelligence” below for a detailed description of the methodology.
- The business case in Figure 2 is very solid, with a breakeven of 14 months and a net present value of $1.8 billion.
- The projected benefits are in line with Wikibon findings from projects that have successfully combined systems of record and inline predictive analytics.
- Executive management should set objectives related to increasing automation within existing systems of record, with a sustainable and agile continuous improvement process. The combination of decision management (including predictive analytics) and systems of record will yield what Wikibon refers to as systems of intelligence (SoI).
- The business value of systems of intelligence is more than ten times higher than what’s achievable with traditional decision support systems, which generally provide insights for the few, versus the masses.
- The time to implement and realize value with systems of intelligence is between 2 and 5 years faster when created on existing systems of record.
- The existing systems of record need to be upgraded to become a True Private Cloud, and to reduce the cycle time of data movement from days/weeks to minutes/hours.
- The idea of Mode 1 in a bimodal model being traditional and sequential is too limiting in our model, and an obstacle to the implementation of systems of intelligence. In order to create systems of intelligence, systems of record need significant technology investments, and the application development tools and processes must be upgraded. This will enable SoR staff to work in close harmony with data scientists and line of business analysts to create systems of intelligence.
The Time Value of Data
Traditional data warehouses provide reports back to users in hours at the very best, but usually days or weeks. Data has to be moved from production systems to data warehouse systems through an ETL process (Extract, Transform, Load). The ETL process has to clean and reformat the data (often from a row-based format suitable for transaction processing to a column-based format suitable for queries). These jobs are serial, bandwidth intensive and long-running, usually outside prime business hours.
As Figure 3 below shows, the result is a decision support system that help executives, managers and professionals steer their organization by analyzing what happened days or weeks before. If you are steering a ship or a company, the value of the data is far, far less than real-time or near real-time data.
Wikibon has conducted hundreds of in-depth interviews with community practitioners every year, as well as thousands of interviews on theCUBE. When senior executives are asked about the ROI for current data warehousing and big data projects, enthusiasm is tepid at best. In a recent survey we conducted, the ROI for big data projects was a paltry 50¢ for each $1 invested. Wikibon’s conclusion from these findings is that making a few people even smarter in an enterprise is not an efficient way to improve financial performance. Information is often held back for optimum value to an individual or small group. The benefit of better data delivered after the fact is not a game-changer.
Figure 3 depicts the diminishing value of data over time and underscores the positive organizational impact of bringing analytics to transaction systems inline (i.e. in real time). The graphic uses the analogy of trying to steer a ship by looking at where it’s been versus where it’s headed.
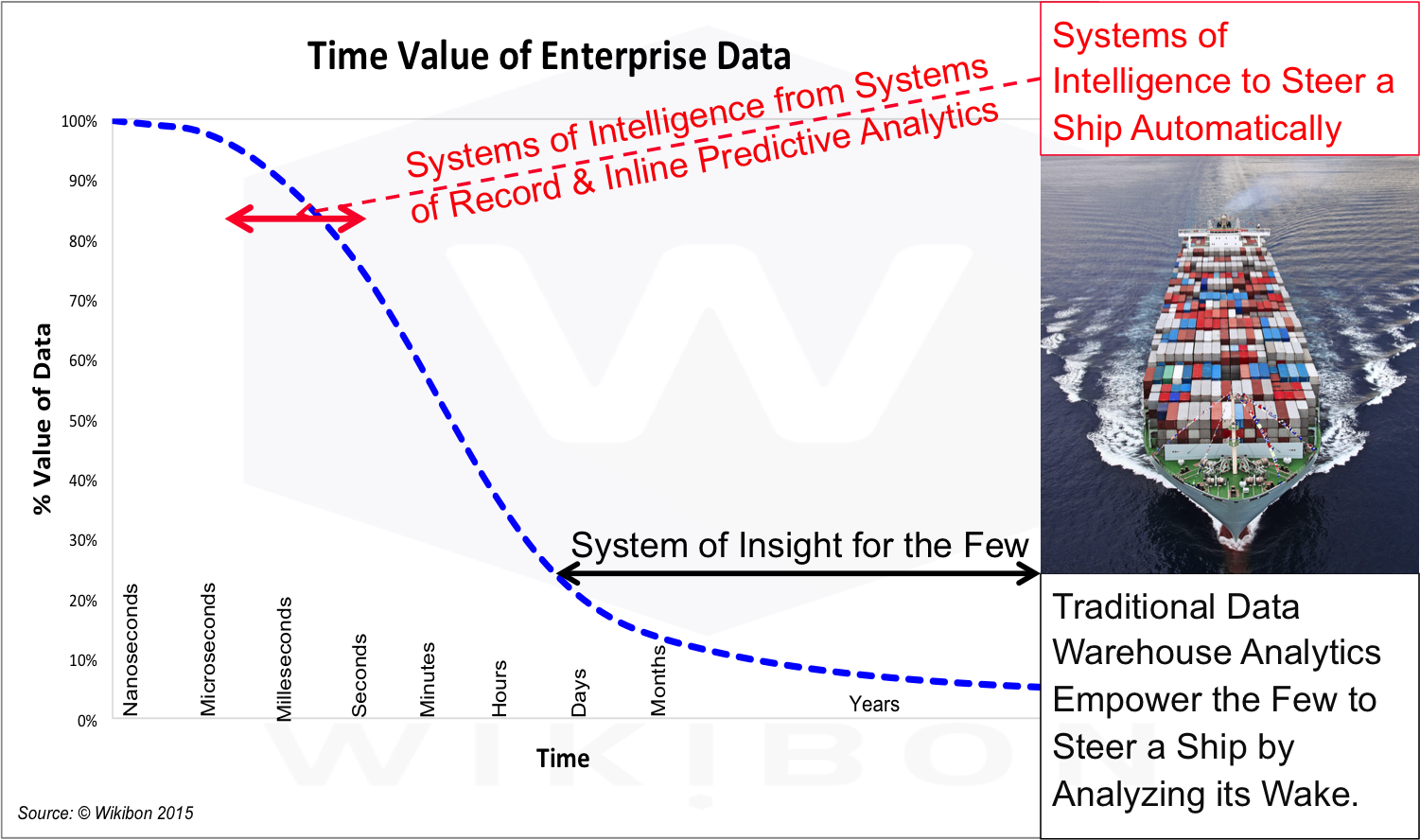
Source: © Wikibon, 2016
The top of Figure 3 shows that providing data in milliseconds can support real-time automated decision making. Our assertion is simple: Inline predictive analytics can help automate business process components in real-time, faster, more consistently and more accurately than human operators. And at a much lower cost. Early systems that bid in real-time for individualized advertisements have shown very high and enduring returns. Real-time fraud detection systems for credit-card and insurance companies have shown nine to ten figure returns. Wikibon research indicates that when ensuring insight is translated into operational automation, the benefits for the whole of the enterprise is a game-changer. Leaders in the Wikibon community stress that it’s the blending of analytic and transaction systems that yield competitive advantage and broadly applying so-called Mode 2 methods to managing both these systems is the right approach (rather than forking approaches).
Development Process for Systems of Intelligence
Figure 4 shows how all the enterprise IT processes combine to create system of intelligence. The top of Figure 4 shows the data coming in from billions of sensors feeding the internet of things, pumping data through edge machines into the cloud. This data is merged into mega-datacenters, filtered through data aggregators and made available as real-time streams. The data contains insights for enterprises about potential disruptions, threats and of course, business opportunities. A selection of these data flows may come through enterprise real-time streaming systems, i.e. data on the location of enterprise assets, personnel and customers. The data streams initially will go to data lakes and the right-side of the triangle as part of the predictive analytic research phase. When a production model has been created, selected external data streams will be incorporated into real-time streaming systems and integrated into the systems of record. This is where we believe competitive advantage will be most noticeable.
Also arriving on the left-hand side of the charts is enterprise data from personnel, partners and customers. These are fed into the systems of record to determine what products are ordered, what components are purchased, produce bills and statements, pay invoices, cut paychecks and support myriad operational business processes. Some of the systems of record are outsourced to the cloud through Software-as-a-Service (SaaS), such as payroll, email, HR and CRM systems. Core systems can be written and supported by the enterprise and are often based on integrated packages from IBM, SAP, Oracle and many others. Many are highly modified by enterprises to support specific differentiating business processes. These systems are usually transactional and support thousands of micro-decisions made by customers, employees, suppliers and partners.
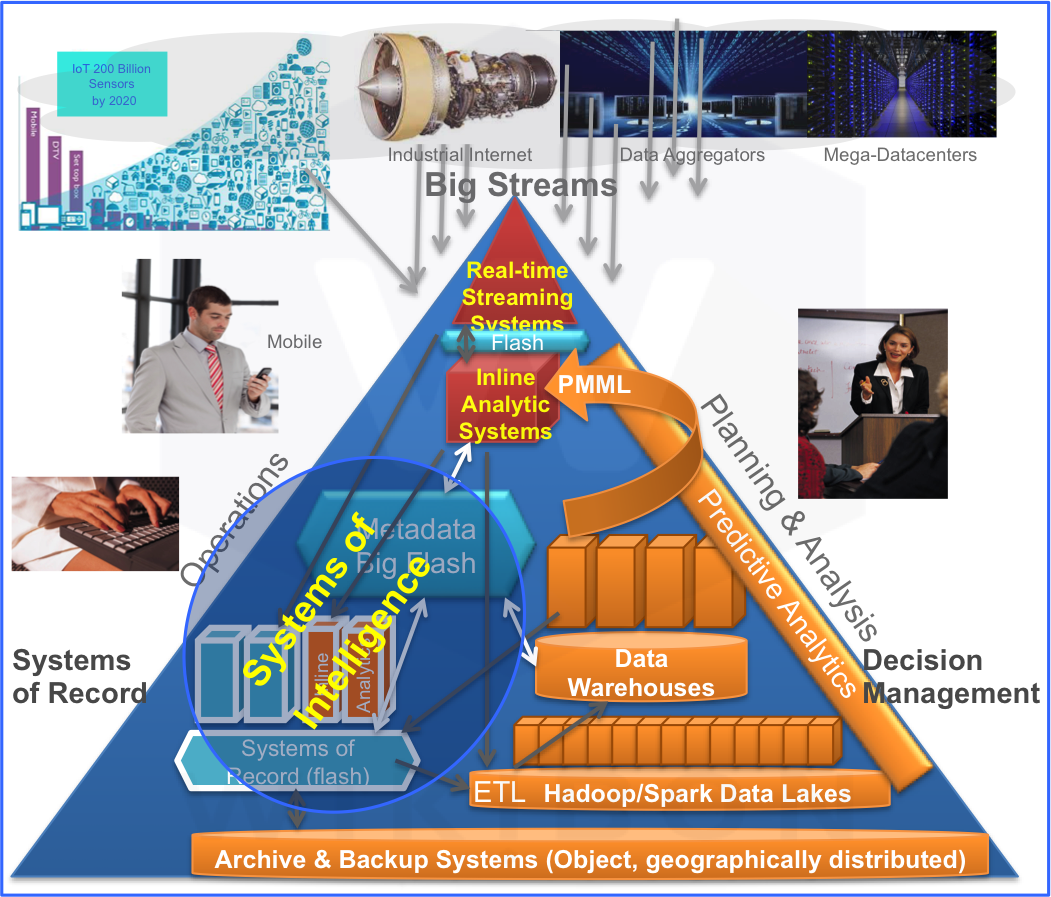
Source: © Wikibon, 2016
The systems of record on the bottom left of the triangle support data flowing into data lakes and traditional data warehouses. The movement of some data to the data warehouse can be very significantly increased by the use of consistent snapshot copies using upgraded flash storage on systems of record. Large volumes of historical data are held in the data warehouse, and external and less structured data held in the data lakes, driven by Hadoop and Spark open source technologies.
Spark is a particularly interesting new technology, because does not rely on a specific underlying data store (Hadoop utilizes HDFS). Spark can analyze data in-place without requiring movement. Spark has been enabled on most platforms, including z/OS mainframes and Unix/Linux. Models can be developed within the SoR, and Spark can be used to combine the results of analysis across multiple platforms within the SoR.
The analysts and data scientists will use this data together with predictive analytic tools used to create and test models against current and historic data. This is a strongly iterative process, requiring quick turnaround and significant resources. These models are developed using the Predictive Model Markup Language Resources (PMML) – see PMML Note2 in the Footnotes below for more details. PMML allows a rapid transfer of models from development into production, using toolsets from companies such as Zementis. This is illustrated in Figure 4 by the arrow with PMML from analysis to inline analytic systems.
The inline analytic systems run either within or very close to the systems of record. Importantly, these processes must be completed in milliseconds. As such multiple fast processors, high bandwidth, proximity between analytics and transaction data and a single coherent process are prerequisites to this approach. This level of integration enables production systems of intelligence to be implemented directly on the system of record, as shown in Figure 4 above.
The bottom line of Figure 4 is there is so much data flowing through organizations that has historically been used for reporting purposes. Organizations can leverage this set of hardened assets (without ripping and replacing) to build a new model of automated decision-making that is actionable by many more constituents (including machines) versus only a few people. Moreover, this new approach “completes a virtuous circle” where analytic insights are fed back in real time to transaction systems and continuously improved over time.
Technologies exist today to enable this capability and they will only improve over time.
Infrastructure Requirements for Systems of Intelligence
Supporting these systems of record are large integrated databases from enterprise companies such as IBM, Microsoft and Oracle, and from open-source tools such as MySQL. These systems of record need to be secure and demonstrate compliance with government regulations.
The hardware and software infrastructure platforms that support database transactional systems for systems of record include IBM z mainframes, large Unix systems, Linux systems and Microsoft Windows Server systems. The infrastructure is housed in enterprise data-centers, outsourced to mega-datacenters, or runs on public cloud Infrastructure-as-a-Service (IaaS).
All-flash Strategy
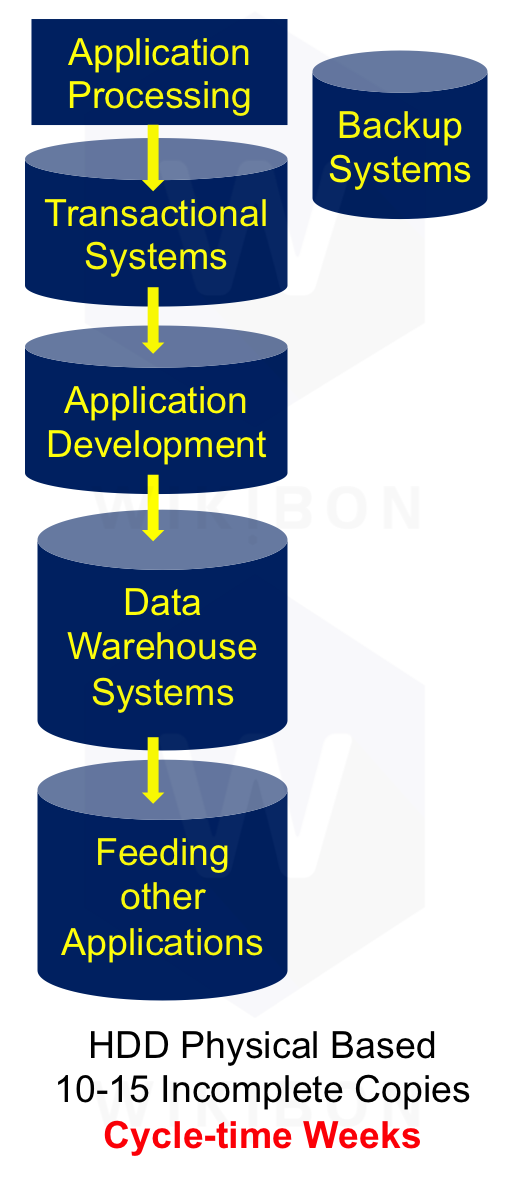
Source: © Wikibon 2015
A key step in enabling systems of intelligence is to reduce the elapsed time for IO, and to severely reduce the time to move data from one application/database to another. Figure 5 shows the traditional workflow with magnetic disks (HDDs). Because of the severe limitation of HDDs, the same copy of data is very difficult to share. Snapshots can be made quickly from HDD data (for example to provide a consistent recovery point). But using that snapshot to share the data with developers or other application users is not possible because of the low number of IOPS each individual hard disk can support. Data on disk optimized for one use (e.g., systems of record database transactions) is not able to be shared with IT applications optimized for data warehousing or other applications. Additionally, that data cannot be shared with application development because mixing production data with application data would impact performance on the production systems. The result is practitioners make multiple copies of the same data within the data center. About 10-15 copies of the same data is the number found in a typical data center, and it can be much higher. These copies (clones) usually have to be made at night or weekends, so as not to interfere with production. The copies (especially for application developers) are incomplete copies of the production data, usually made weeks later. Figure 6 shows the same data workflows in an all-flash datacenter environment. The same data can be shared across all the different uses of the data, because the underlying flash technology can handle hundreds of thousands of IOs, instead of the just 100 or 200 IOs that a single HDD can manage.
Logical copies are made by creating an application consistent snapshot of the data. The logical copies of data are managed by metadata, held in protected DRAM and Flash. The data in Figure 6 assumes all-flash storage is installed as a prerequisite for systems of record, and a metadata flash repository is highlighted for managing a catalog of all the logical copies, the delta-data and the mapping to physical copies (as well as controlling provenance of data and maintaining compliance data). The benefits of an all-flash strategy for systems of intelligence include:
- The potential for a 4 x reduction from compression and de-duplication;
- The potential for a 6 x reduction in cost from data sharing and copy elimination (it may take 18-24 months to achieve this level of sharing;
- As the 4x and 6x reductions from the two elements above are multiplicative, the potential is a 24x reduction in the raw storage required, which can eventually save storage costs;
- An ability to dramatically increase the productivity of DBAs and storage specialists, as storage constraints become a thing of the past;
- A key step (along with true private cloud) for laying the groundwork for moving to a DevOps model;
- Much faster response time for all current system of record applications, increasing end-user productivity and customer satisfaction;
- Ability to update key data warehouse tables in near-real time, and produce reports/alerts in near-real-time to assist current business processes;
- Ability to reduce the cycle-time of data moving through an enterprise from days/weeks for physical copies to seconds/minutes/hours for logical copies;
- The amount of new functionality that can be programmed to improve end-user productivity can be increased by a factor of six times (6x) (see Note3 below, and Step 3 of Wikibon research entitled “Driving BusinessValue for Oracle Environments with an All-Flash Strategy“);
- Ability to deploy new applications – i.e. Systems of Record mixed with predictive inline analytics without impacting production performance;
- Ability to increase the size and complexity of the predictive analytics production model and increase the levels of automation to the line of business processes.
True Private Cloud
Wikibon projects that systems of intelligence will increasingly run as on-premise or hosted “True” private clouds, and on public SaaS or IaaS clouds. The true private clouds achieve public cloud levels of cost and agility by integrating processor, networking and storage, outsourcing hardware & software maintenance to the vendor, and supporting orchestration, automation and self-service capabilities. IBM z mainframes, Linux/Unix systems and Windows systems have or are expected to have true private cloud and hosted true private cloud offerings in 2016, which are projected to offer similar or better cost and agility when compared to public clouds.
True all-flash private clouds will support enterprise IT retaining systems of record on their current platform, and focussing on improving business processes by integrating automated decision execution using predictive analytics into the existing systems of record.
10 Strategic Steps to Implement Systems of Intelligence
The following ten strategic steps to implement SoI are a summary of the actions that should be initiated and monitored by senior executives:
- Keep core systems of record on current platform(s):
- Understand the cost, elapsed-time and risk to convert core systems from one platform to another is very high, and strongly resist calls to convert systems of record in whole or in parts;
- Ensure that the ability to integrate inline predictive analytics is not compromised by other infrastructure decisions;
- Prepare to implement predictive analytics and the ability to process the data streams inline, and execute the decisions within the current Systems of Record.
- Improve infrastructure of existing systems of record:
- Migrate to 100% all-flash storage to improve IO times and reduce IO processing time, preparation for additional IO load from predictive analytical processing;
- Migrate to True Private Clouds (see Wikibon research for definition of “True” Private Cloud) to ensure that the system of record equipment and support costs are competitive;
- Enable data sharing via space-efficient snapshots using the flash storage to drastically reduce data promulgation and IT cycle times;
- Utilize Spark technology on these data shared snapshots of production data to enable direct analysis (including in-memory processing) of the data on the SoR (Spark is supported on z/OS mainframes and Unix/Linux Systems), and if necessary combine it with the Spark results from other systems/platforms;
- Move away from administration silos for server, storage and network, and move towards a DevOps Model.
- Improve Productivity of System of Record Application Developers:
- Enable much faster data access by publishing complete copies of production databases and code bases from shared data on flash to improve programmer productivity, shorten development cycles and improve code quality (see Step 2 of Wikibon research entitled “Driving Business Value for Oracle Environments with an All-Flash Strategy“;
- Update development tools and platforms on-premise or in the cloud to shorten development and maintenance cycles;
- Train system of record developers on the basics of predictive analytics, PMML standards, and how to implement and update inline predictive analytics on systems of record;
- Introduce a strong incentive plan to reduce time-to-value of new and improved analytic algorithms from months to days/hours (e.g. by utilizing PMML Standards).
- Place Analysts/Data Scientists Close to the Line of Business:
- Introduce a strong incentive plan for managers and professional to move from insight to inline predictive analytics models and algorithms that can implemented within the Systems of Record;
- Ensure a cooperative and close relationship between analysts, data scientists and system of record developers, with common incentive plans rewarding final integration and production.
- Understand Potential Data Sources inside and outside Organization:
- Data from the Internet of things;
- Data from social, mobile & industrial Internet;
- Understand the potential to add sensors and security/video feeds within the enterprise (warehouses, trucks, etc.);
- Understand the potential availability of data from data aggregators (e.g., data about supply chains within an industry).
- Position Location of Data Sources to Minimize Cost & Elapsed-time to move Data:
- Ensure that all the important data sources are available and can be moved fast enough to include in real-time inline analytics;
- If or when external data sources become a cost or time to move issue, investigate potentially locating systems of record in a mega-datacenter close to cloud services & external data sources.
- Provide Best-of-breed Analytic Tools to Analysts/Data Scientists:
- Enable access to on-premise and cloud-based analytic tools and platforms and all data sources;
- Training to migrate Systems of Insight to Inline Analytics to be implemented on Systems of Record.
- Grow/Evolve the Capabilities of Analysts/Data Scientists:
- Encourage enhancement from simple scoring models to clustering models, decision trees, neural network models, naïve Bayesian classifiers, random forest models etc.;
- Implement a strong incentive plan to move from systems of personal insight to inline predictive analytics and algorithms that can implemented within the systems of record;
- Create and close relationship with system of record developers and shared incentive plans;
- Implement Standards (e.g., PMML) to Facilitate Direct Transfer from Model Creation to Model Deployment:
- Strong Incentive Plan to Reduce time-to-value of new and improved analytic algorithms from months to days/hours (e.g. by Utilizing PMML Standards to move Models and Model updates to System of Record Developers).
- Gain success from one business process in one line of business and rapidly expand to additional business processes and additional lines of business.
- C-level executives should take particular care to ensure that data models are not hijacked by senior executives for their sole use, and should ensure the projects to improve SoR to automate business processes are funded and completed.
Wikibon strongly believe that these steps are a pre-requisite to achieving a sustainable return on investment from big data and analytics. The next section gives a method for quantification of this strategy.
Business Case for Systems of Intelligence
For this business case we used IBM’s technology portfolio as a reference model to build support the processes laid out in Figure 4. IBM mainframes are the classic gold standard for large systems of record. As well, IBM has been the largest big data vendor (in revenue terms) for the last two years with the broadest portfolio of predictive analytics in the industry. See “Big Data Vendor Revenue and Market Forecast 2013-2017” and “Big Data Vendor Revenue and Market Forecast 2011-2026” for details on IBM and other vendor shares.
We assumed these inline analytic systems run either within or very close to the systems of record (in this case we used an IBM z mainframe). For an analytics accelerator, we assumed a high-performance appliance using Netezza technology. This is supported by DB2 running in the mainframe, and using the MPP appliance to speed up processing without any additional coding.
Note: An alternative solution could have been running Linux within the mainframe. The z mainframe has a very fast processor (5.0GHz) and high speed memory bus interfaces between the processors.
The level of integration we assumed enables production systems of intelligence to be implemented directly on the system of record, as shown in Figure 4 above. PMML tools support the running of development and production code on many different platforms, including zOS.
The business case is developed on the previous assumptions and recommendations from earlier sections. The investment priorities for the initial incremental budget of $25 million dollars in the first year is as follows:
- Establish a team of business analysts and data scientists to work on the first project (assumed here to be a radical improvement in early fraud detection);
- Upgrade storage for z mainframe Systems of Record to 100% Flash;
- Upgrade z Mainframes to create a “True” Private Cloud;
- Upgrade the application development systems and processes for the systems of Record to improve application development productivity and time to deploy;
- Evaluate and deploy IBM Analytics & Decision Management Software;
- Deploy Spark on z/OS, in conjunction with Rocket Software’s Mainframe Data Service for Apache Spark (MDSS) (MDSS can render virtually all z/OS data stores, including DB2, IMS, VSAM, ADABAS, etc. into Spark RDDs for in-memory analysis without copying the source);
- Decide on the development platform(s) for inline predictive analytics platform (z/OS mainframe, Power systems, public cloud systems, etc.)
- Deploy PMML tools and methodologies;
- Develop initial fraud models for testing migration from development models to production;
- Test and select data sources from within and outside the enterprise;
- Establish a methodology and processes for updating the development model and migrating to production.
Figure 7 gives a summary of the key metrics in the business case, derived from Table 1 in the footnotes below. The detail shows a very robust case for investment in this approach. It shows significant benefits from reducing headcount and increasing revenue (or maintaining headcount and more dramatically increasing revenue). The returns are orders of magnitude better than the case for better and faster reports for executives and professionals to take action. The harsh truth is that reports and sophisticated charts are used more as weapons to protect and advance than a shared resource for the advancement of the company as a whole. By focusing on each line of business and making incremental change which automates the business, the changes are permanent, can be improved iteratively, and are owned by the line of business. The key metrics in this case are:
- An initial investment in year 1 of $25 million from a IT budget increase of 0.5%;
- A net present value (NPV) of $1.8 billion over 5 years;
- The key benefits are a projected revenue increase of 15% five years out.
- This assumes a reduction in employees of 3.2%/year;
- If headcount stayed flat, the model would show a 35% revenue increase at the end of the period;
- Note: Organizations can choose to cut headcount costs or more dramatically increase revenue if market demand exists;
- An internal rate of return (IRR) of 183%, including an overall investment in IT of $209 million;
- A breakeven of 14 months.
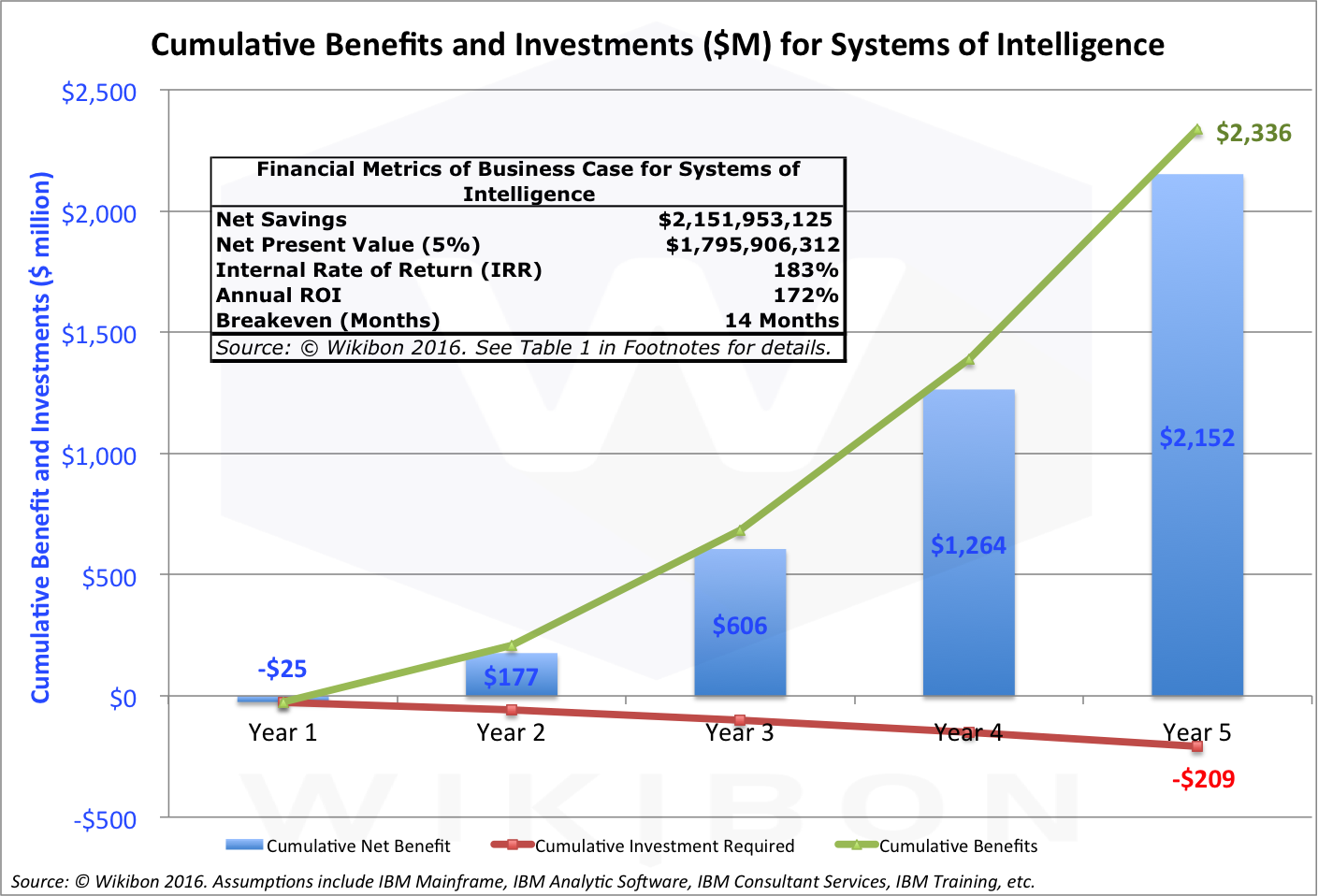
Source: © Wikibon, 2016. See Table 1 and Table 2 in the Footnotes below for details of the assumptions and calculations.
Figure 8 below show the improvement in application value contributed by it as a result of this project. Wikibon has developed a method of measuring the contribution of IT applications to the business. Application value is measured as the contribution of the application to the business. This is a simplified version of a detailed methodology.
Wikibon deploys user surveys when working with enterprises to determine how much time they are actively using IT, and how productive they are using it. By using it evaluate a whole portfolio of applications, the results of this analysis can guide application investment decisions.
When applied to systems of record, the key assumption in this analysis is that while users of the system are using IT, they are as productive as all the other components of their work (meetings, phone calls, etc). The other assumption made is that users spend about 15% of their time actively using IT (have an application just open does not count – it is time actively using it). Some platforms can give good metrics on usage to help improve the data.
Application Value is given by the formula “Application Value = Number of Users x Revenue per User x % of time using IT – IT Budget used for Applications” The revenue/user is a measure of the value a user gives to the organization, and is typically 3 to 4 times the loaded salary.
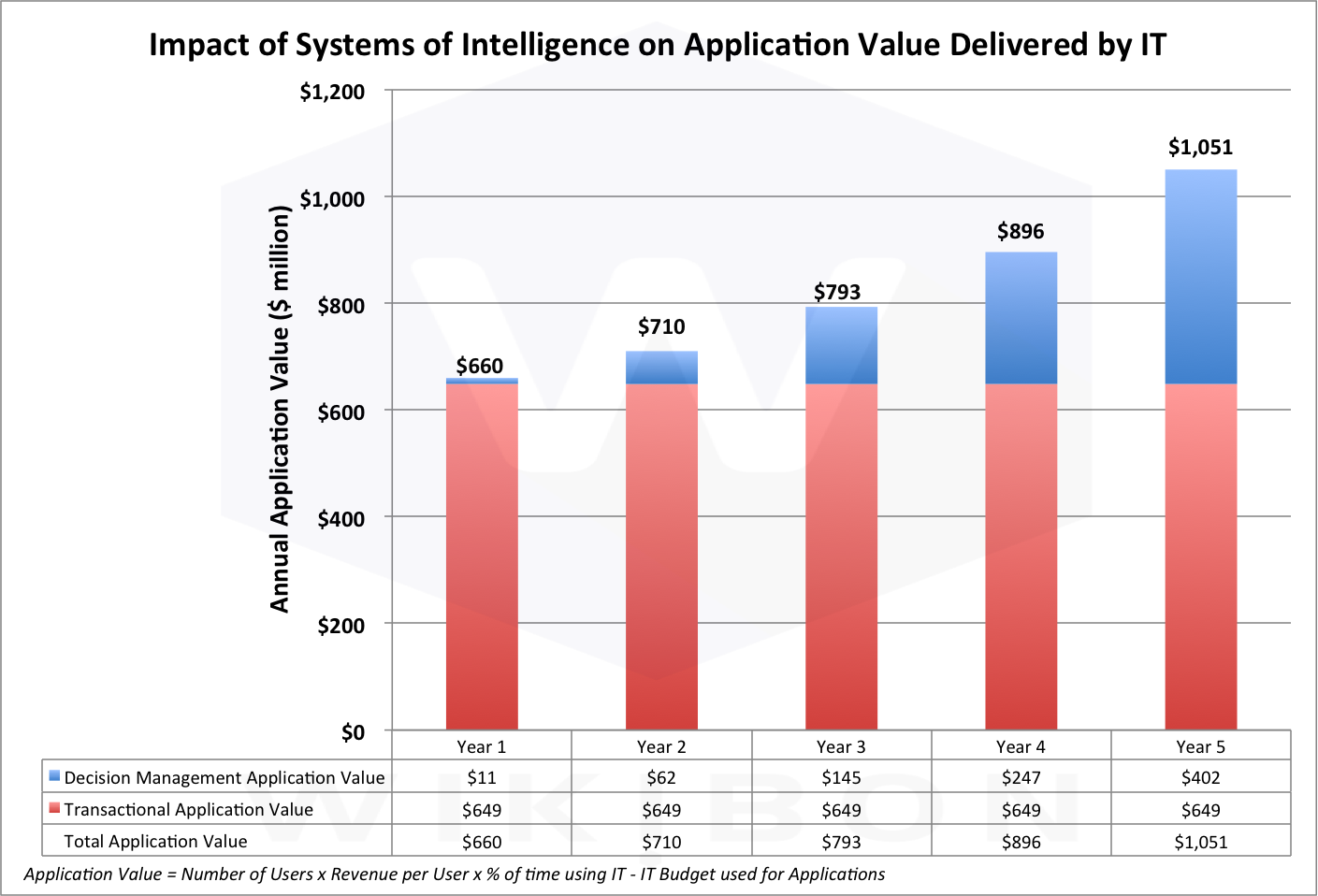
Source: © Wikibon, 2016. See Table 1 and Table 2 in the Footnotes below for details of the assumptions and calculations.
In Figure 6, the red columns show the application value delivered by the existing systems of record, and it is assumed that there is little change. The major change is adding the inline predictive analytics, which have the ability to significantly increase the productivity of the end-users as a whole. The additional contribution to application value of the system of intelligence is shown by the blue bars. It shows that the systems of record are delivering 59% more value in a year at the end of the five year period.
The bottom line is that systems of intelligence in this rapidly changing business landscape are vital to enabling enterprises to disrupt or to avoid being disrupted.
Action Item:
C-level executives, senior IT executives and line of business executives should focus on moving decision management from making a few people smart to automating the enterprise and making the whole enterprise more productive. The first key in achieving this objective is a significant investment to speed up systems of record and to improve the ability of developers to make changes to systems of record in hours/days. The second key is to run production inline predictive analytic systems on or very closely integrated with the systems of record. This strategy holds whether the system of record is currently running on-premise, as a SaaS, or in a public IaaS cloud. The development of predictive analytics can be completed on the platform most appropriate for development, using PMML to ensure ease of migration from development to production.
By incentivizing all levels of management and professionals to adopt this approach, organizations will be able achieve large and sustainable returns on investment. All other strategies, especially those requiring conversion of systems of record to another platform or another package, will almost certainly add years to the project and add enormous business risk.
Footnotes:
Table 1 is the detailed model based on the Wikibon Standard Large Business Model, for an enterprise with revenues of $5 billion, and 20,000 employees. The figures in blue are assumptions used in the model. Table 2 below has more details on these key assumptions. The timescale is a strategic 5-year view.
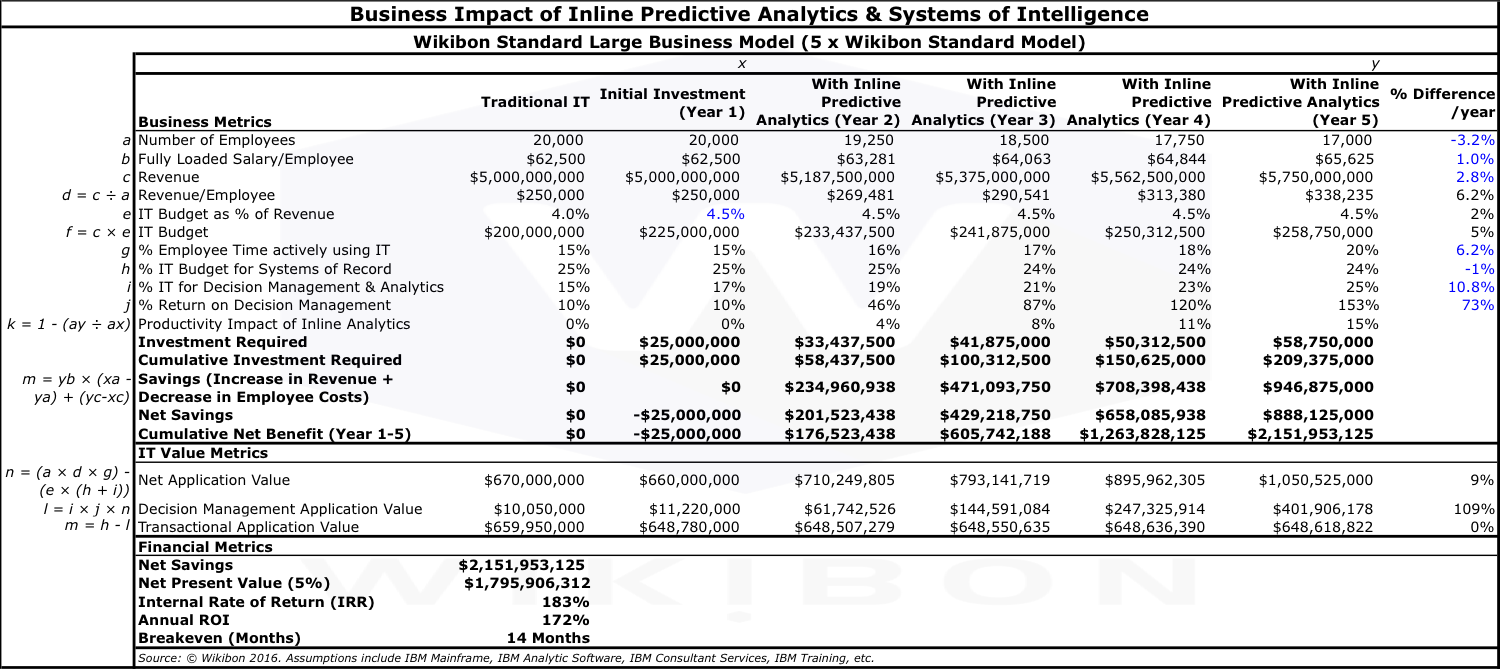
Source: © Wikibon, 2016
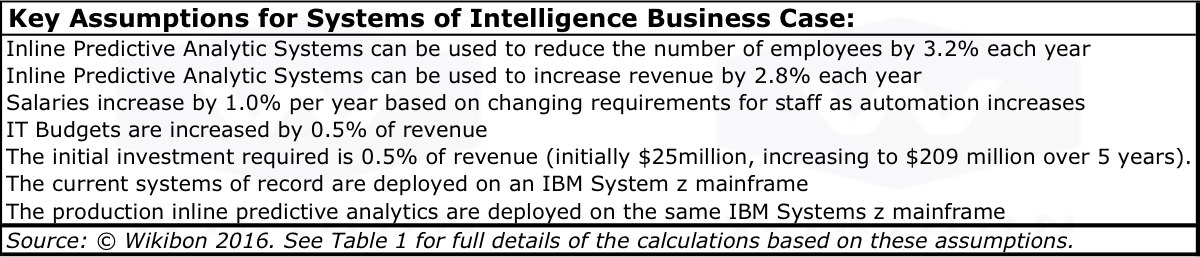
Source: © Wikibon 2016. See Table 1 above for other assumptions and calculations
Note1: Bimodal IT
The Gartner Bimodal IT definition is: “Bimodal IT is the practice of managing two separate, coherent modes of IT delivery, one focused on stability and the other on agility. Mode 1 is traditional and sequential, emphasizing safety and accuracy. Mode 2 is exploratory and nonlinear, emphasizing agility and speed. Source: Downloaded from http://www.gartner.com/it-glossary/bimodal on December 27 2015 at 11:14am.
Note2: PMML (Predictive Model Markup Language) has the following attributes:
- An open standard developed by the DMG (Data Mining Group);
- XML-based language used to define statistical and data mining models and share these between compliant applications;
- Avoids proprietary issues and incompatibilities when models are deployed;
- Supported by many leading commercial and open-source analytic tools;
- Data handling and transformations (pre-and post-processing) are a core component of the PMML standard;
- Allows for the clear separation of tasks: model development vs. model deployment within systems of record;
- Greatly reduces the need for custom code and proprietary model deployment solutions;
- Key enabler of reducing time-to-model-deployment from months to days/hours;
- Is integrated into toolsets by many vendors such as Zementis.
Note3: Assuming that the initial state was 80% maintenance and 20% new stuff, and conservatively assuming programmer productivity is doubled, the maintenance will go from 80% to 40%. The 20% new stuff will go to 60% new stuff (3x improvement), and during this 60% of time twice as much new stuff will be produced (3x x 2 = 6x improvement in new stuff produced). This improvement in productivity allows resources to be applied to developing Systems of Intelligence without new hires.
Updates:
January 5 2016: updates to include additional information on the role of Spark for analytics, and other small changes (df)