Contributing Authors
Ralph Finos
George Gilbert
Peter Burris
Premise
Spark adoption is pretty certain, but different classes of users are taking different approaches to the adoption process.
New technology adoption never follows a straight and narrow path to broad usage. Rather, adoption twists and turns in response to an array of technological, social, and financial factors. The adoption of Apache Spark is no different. In the Fall of 2015, as part of our big data market forecasting effort, Wikibon surveyed over 300 members of the big data community to take their pulse on Spark interest, its benefits, and its weaknesses vis a vis Hadoop. The results show that the adoption of Apache Spark is assured and most influenced by both technology and political factors, including:
- Spark is better in crucial ways. As a technology, Spark is broadly recognized for its performance and streaming data advantages, even among Hadoop diehards.
- Data scientists are taking advantage of Spark’s benefits. The professionals most closely associated with big data project success or failure — data scientists — are ready to add Spark to their toolkit
- Spark is a pawn in emerging infrastructure battles. Even though Spark is viewed as superior in specific ways, not all believe that Spark is generally superior to Hadoop. The real debate about Spark pits infrastructure groups with Hadoop experience against those without. Hadoop experience today appears to be a marker for lower Spark enthusiasm among technology administrators

Of course, Spark and Hadoop functionality sometimes directly competes and sometimes is complementary. So, these comparisons of opinions should be interpreted more precisely as current and prospective user expectations about Spark and its ability or potential to replace at least some of the key analytic engines within Hadoop in a more satisfactory manner. In addition, there will be situations where users and prospects are considering running Spark without any Hadoop technology whatsoever.
To Know Spark Is To Like Spark
Of the 300 big data professionals that we surveyed, nearly 60% were using Hadoop and using or evaluating Spark. Of that group, about half thought Spark a superior or complementary option, a strikingly large number for a complex technology that, at the time, had been available as a 1.0 release or higher for less than 18 months (see Figure 1). Another third didn’t discern a real difference between the two technologies. Fewer than one-in-six believed Hadoop was better.
Multiple factors contributed to the generally favorable assessment of Spark by our community. Performance and functionality topped the list, which should be expected given that Spark was built to operate in-memory and supports non-batch processing modes, like streaming. But our community also indicated that their evaluations highlighted simplicity and better support for emerging, not-yet-defined classes of applications. To a degree, these responses reflect a bit of wishful thinking: over time, Spark may not remain a focal point of invention. Hadoop’s complexity is a response to the evolution of understanding about big data. As folks used Hadoop to solve new classes of problems for the first time, they discovered important technological limits, which compelled the open source community to extend the Hadoop toolkit, sometimes using discordant approaches. Spark, like other open source software, may go through a similar evolution. Similarly, as new big data application forms become better defined, new technologies will be built specifically to serve those applications, and those technologies may bypass Spark.
“What Is It, I’ll Take It”
As the disciplines of big data evolve, the high priests of big data — data scientists — are showing little technology bias in their use of tools to get the job done. Of course, this has positive and negative ramifications. On the positive front, data scientists appear focused on successful application of big data techniques, learning and applying evolving tools, and the pursuit of business returns. On the negative side, data scientists are littering the big data landscape with discarded tools and service contracts, avoiding accountability for failures, and minimizing collaborations with other internal groups that might slow down this project today, but may institute best practices that increase success rates of a greater number of future projects.
Figure 2 displays Hadoop vs. Spark Attitudes by Job Role. Generally, the pro-Spark attitudes of data scientists seem to stem from a few sources. First, they tend to regard Spark as easier to implement than Hadoop, a significant benefit to a professional focused on time-to-value. Second, Spark is believed to be relatively easier than Hadoop for business people to use; although that attitude likely is similar to believing that getting swarmed by zombie clowns is better than having a piano fall on your head. Third, data scientists are keenly aware of the performance advantages of Spark, noting, as one respondent did, “When it’s time sensitive, Spark produces better results.”
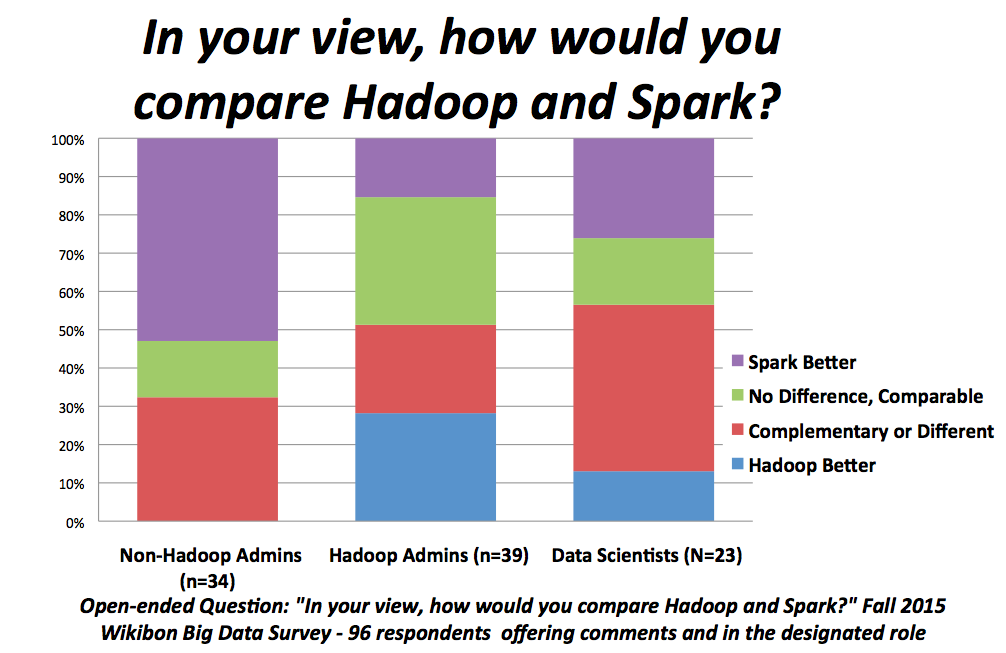
Source: Wikibon, 2016
“Got a Bet There, I’ll Meet It”
The largest gap that popped out of our Spark attitude data was between big data infrastructure professionals who identified themselves as Hadoop admins and those that said they were not. As Figure 2 shows, only 15% of Hadoop admins believe that Spark is better, whereas over 50% of big data infrastructure pros that aren’t administering Hadoop believe that Spark is better. Why the difference? And what does it mean?
Our data doesn’t definitively reveal the reason for the difference, but in our conversations with community members we think three factors are at play. First, some portion of the non-Hadoop admins are, in fact, Spark admins; not surprisingly, they would indicate a preference for Spark. Second, and on the flip side, Hadoop admins are standing behind the value of their experience by expressing a preference for Hadoop. But beyond the two obvious answers, our research suggests there’s another group weighing in: data warehouse admins, normally associated with traditional IT groups, that now are self-identifying as big data professionals and seek to leapfrog Hadoop admin groups, many of which carry shadow IT credentials, with Spark. The emergence of Spark, then, is likely to set off a political battle among the various business functions that are laying claim to management of analytics capabilities, including IT, marketing, and other groups that are developing big data competencies as part of their functional mission.
Action Item
Immature big data technologies are being applied to a wide variety of business problems. As should be expected, the big data approach taken is dependent on the composition of the regime taking the approach. CIOs should encourage this diversity — even competition — among these groups, but keep a close watch out for overspending due to inexperience and cultural acceptance of systemic failure to deliver results.
Methodology
In its Fall 2015 survey of 300 Big Data practitioners, Wikibon asked Big Data users “In your view, how would you compare Hadoop and Spark?” The respondents selected for this comparison self-identified as their primary Big Data responsibility:
- IT Admin – A datacenter professional who manages infrastructure/hardware associated with Hadoop and NoSQL database deployments that support Big Data Analytics projects
- Data Scientist – Advanced analytics professional conducting sophisticated analytics and develops predictive models/algorithms on large volumes of “messy” data
Response sample size for each group:
- IT Admins in Hadoop environments (n=39)
- Big Data Scientists in Hadoop environments (n=23)
- IT Admins in non-Hadoop environments (n=34)
Some respondents did not substantively answer the open-ended question, so their data was excluded from the analysis. Survey respondents could also self-identify as Application Developers, Business Analysts, or Business Users as their primary role. Practitioners in these job roles did not show the diversity of responses seen among the three groups chosen for our analysis.