Wikibon Contributing Analyst
Ralph Finos
Premise
Steady progress relative to last survey 18 months ago shows pilots and proofs of concept continue their conversion into production applications, with 41% reporting one production deployment, up 10%.
- Like all major enterprise technology adoption lifecycles, customers’ ability to deploy the software is gated by their ability to absorb and assimilate complex software.
- Gating factors to higher growth with on-premises Hadoop, specifically, are its high administrative overhead and specialized skills requirement.
Two implicit and major implications create a disconnect between 100% headline vendor growth and on-the-ground reality.
- Lots of unconsumed software piling up in “inventory” with customers – just like ERP and enterprise Internet infrastructure adoption bubbles in mid-to-late ‘90s; vendors likely pushing larger deals than can be deployed in the current year in order to make economics of direct sales work in an era of subscription software that features much less up-front revenue.
- Hidden challengers, especially as Hadoop moves into mainstream: Hadoop-as-a-Service and cloud native services from AWS, Azure, Google are easier to “consume” because of less demanding administrative and skills overhead.
Executive Summary
Headlines from vendors and research firms tout triple digit revenue growth numbers for Hadoop vendors and numbers near that level for many other participants in the Big Data analytics ecosystem. Wikibon’s survey results highlight a disconnect between these headlines and what’s happening on the ground. That disconnect represents a growing “inventory” of software accumulating with enterprise customers. At some point we may see an inventory “correction”, where they slow purchases so that deployment can begin to catch up.
Hadoop and Big Data analytics adoption parallels in many ways that of two hyper-growth software markets of the mid-to-late ’90s. ERP applications such as PeopleSoft and enterprise Internet infrastructure software such as Broadvision both saw similar growth. But enterprises couldn’t absorb software at those growth rates then and our survey results show that they can’t now, either.
The administrative and development skills and the operational processes to deploy and run new application architectures, then and now, can’t grow at triple digit rates. Fear of Y2K disruptions from legacy applications accelerated purchases of ERP software. And fear of missing out on the Web revolution propelled Internet infrastructure 15-20 years ago. Similarly, we believe fear of missing out on the Big Data analytics revolution is driving purchases now.
In addition to these demand-side issues, there are new supply-side ones as well. In the mid-to-late ’90s, enterprise software business models paid for enormously expensive direct sales forces, which could consume 50% or more of every dollar of revenue, by selling expensive, up-front licenses to software. The money that covered R&D and profits came from the annually renewable maintenance fees, which added up cumulatively across a growing installed base. With mostly open source software, there is very little up-front license revenue to cover those sales and marketing expenses. And vendors can’t recognize multi-year subscription revenue deals up front according to accounting principles. As a result, there is even greater pressure on vendors to sell large deals that cover far more than customers can absorb and deploy. In turn, vendors can at least bill customers for these purchases, with the cash covering some of their sales and marketing expenses, even if their reported profits are low or negative.
Methodology Summary
Wikibon conducted a web-based survey of 300 practitioners in US enterprises that had either deployed or were evaluating a Big Data analytics projects in Fall 2015. The survey is a follow-up to one conducted in Spring 2014 of the same size and respondent profile. Many of the questions were the same so that we could analyze progress over time. We defined Big Data analytics broadly to include technologies and data that traditional scale-up relational DBMS’s have trouble managing. For a full description of the methodology and profile of respondents, see the Methodology and Demographics section at the end of the document.
Review of Findings
(Where possible, we describe the findings as percentage changes relative to the Spring 2014 survey).
Attitude toward Big Data analytics: 6% more of enterprises see Big Data analytics as a source of competitive advantage
Enterprises are convinced that Big Data Analytics will be fundamental to their business and a new source of competitive advantage (52.1%) as opposed to being primarily a complement to existing Data Warehouse and Business Intelligence workloads (43.2%). In Spring 2014, these two attitudes were shared equally by respondents.
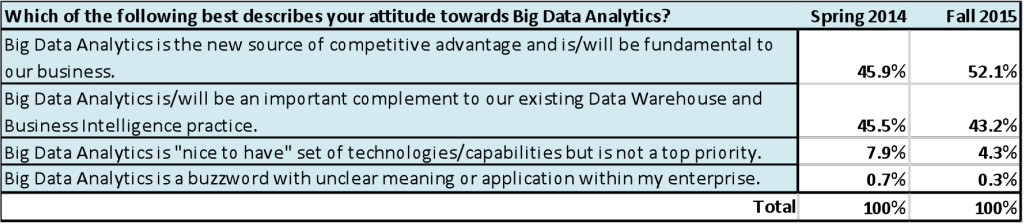
Source: Wikibon 2015
State of Big Data Analytics Deployments: 10% more have at least one production application
Pilots and proofs-of-concept (POC) continue a steady maturation into production. Enterprises are moving from the Evaluation phase of deployment (41% in Spring 2014, 32% in Fall 2015) to deployment of at least one Production application (31% in Spring 2014, 41% in Fall 2015). The shift shows that enterprises are making consistent progress in Big Data Analytics adoption.

Source: Wikibon 2015
Big Data analytics project results: 4% more enterprises report “success” compared to 18 months ago.
Enterprises reported slightly more “success” in Spring 2015 (44.6%) than in Fall 2014 (40.6%). While they are in various stages of Big Data Analytics adoption (Evaluation, Proof of Concept, Production), nearly all (98%) report at least partial value being realized and a sense that they are heading in the right direction.
Consistent with our hypothesis, large enterprises (>5,000 employees), which are more likely to have sufficient technical skills, are 12% are more likely than small-to-mid-size companies to report success (50.7% to 39%).
Assessment of results varied significantly by role. Technical-oriented staff (Infrastructure Admins and Big Data Scientists) were more likely to declare “success” (54.1%) than business staff (Business Analysts and Users) (32.6%). We attribute this 22% difference to the likelihood that technical staff are more likely to see an operational cluster as success. Business staff are more likely to see a usable and trusted repository that has actionable analysis as success. Clearly there is room for growth among business users.
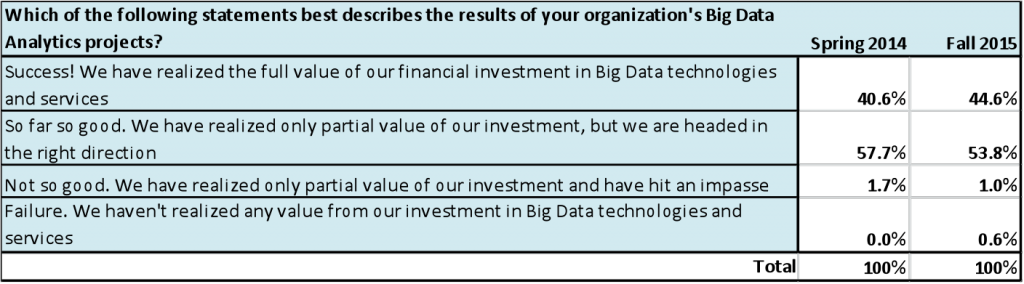
Source: Wikibon 2015
Primary use cases for Big Data analytics: IT operations support and ETL each reach above 50%
IT often deploys new technology to support their own use cases in order to acquire the skills to support broader production deployments. Big Data analytics appears to be following this pattern.
The most prevalent application centers on IT operations support, with well over half citing it (multiple choices were allowed). In addition, 70%+ of these applications are in production.
This choice appears similar to early adoption am0ng large Internet service companies. They used Hadoop to analyze log files and clickstreams for systems management and improving their applications’ features. Splunk’s popularity as an out-of-the-box application that delivers similar capabilities is likely a relevant correlation to this result.
ETL was also an application for a slight majority of respondents. This result is consistent with Hadoop’s “chasm-crossing” application as a data lake that also offloaded ETL processing from data warehouses.
Deployment status of hybrid operational analytic applications: 15% more enterprises are in production than 18 months ago
The incidence of the use of Big Analytics for operational/transactional production applications increased in Fall 2015 (66.3%) vs. Spring 2014 (51.6%). By comparison, those who haven’t deployed an application but are planning to in the next six months dropped 13% to 32%.
Enterprises made marked progress getting at least one of these applications into production over the past 18 months. The fact that the combination of transactional and analytic capabilities was the distinguishing feature of such a large increase in deployment suggests this new class of applications will see wide adoption over time. Many of these applications will fit into what Wikibon research identifies as Systems of Intelligence.

Source: Wikibon 2015
Challenges with supporting operational Big Data analytic applications: near real-time integration and overall performance
While the number of operational Big Data analytic applications in production might be growing, confidence in getting all the pieces to work together properly remains a work-in-progress. The focus is on getting the basic integration and operational performance working smoothly.
IT practitioners and business staff see a range of challenges that register in the range of 40-50% (multiple choices were allowed). These include integrating analytics into operational applications in near real-time or getting the data from operational applications into the analytics; maintaining application performance with large data volumes, high volumes of reads/writes, and large numbers of concurrent users.
Incorporating new data sources and tuning algorithms are less critical challenges today.

Source: Wikibon 2015
Hadoop Usage, Experience, and Plans
61% or 182 of respondents in our sample reported that their enterprise used Hadoop.
Administrative overhead: the number of administrators per cluster dropped by half as customers grew from a single cluster to 3 or more clusters.
Customers with one cluster reported an average of 3.5 administrators while those with greater than 2 reported that number declined to 1.4 because they were better able to leverage scarce skills. Overall, respondents reported that there were 2.2 admins per cluster.

Source: Wikibon 2015
Software deployed on Hadoop clusters
Respondents reported an average of nearly 3 software tools on their Hadoop clusters. Cloudera Manager was cited most often, at 32.4%, but Spark was mentioned almost as frequently, at 29.7%. HBase registered 18.7% with Hive coming in just below at 18.1%. It’s likely that most respondents didn’t count MapReduce as a software tool since it registered rather low.
Planning to use Hadoop in production in next 12 months
The overwhelming number of respondents reported that they were using Hadoop in production now or planned to within the next 12 months.
Top applications (multiple choice)
Unsurprisingly, customer analytics applications such as churn and campaigns collectively ranked above 50%. The initial chasm-crossing use-case of ELT registered above 50%. Fraud detection came in at 37%.
Long-term plans for Hadoop
While recognizing the importance of Big Data Analytics for business success, respondents are not generally ready to embrace Hadoop as a replacement for their data warehouses. 45.6% of respondents expressed their Hadoop strategy in terms of Hadoop and traditional data warehousing technologies playing equally important roles and both getting investments. 31.2% expressed a similar sentiment, but were positioning Hadoop for less mission critical applications. 13.2%, however, were positioning Hadoop as replacing traditional data warehouse technology – either by capturing new spending (6.6%) or by actually transferring some current traditional data warehouse spending to Hadoop instead.
Larger companies then had a more aggressive stance towards Hadoop deployment relative to data warehouses than did small enterprises, which were more likely to see both Hadoop and traditional data warehousing having “equally important roles”.
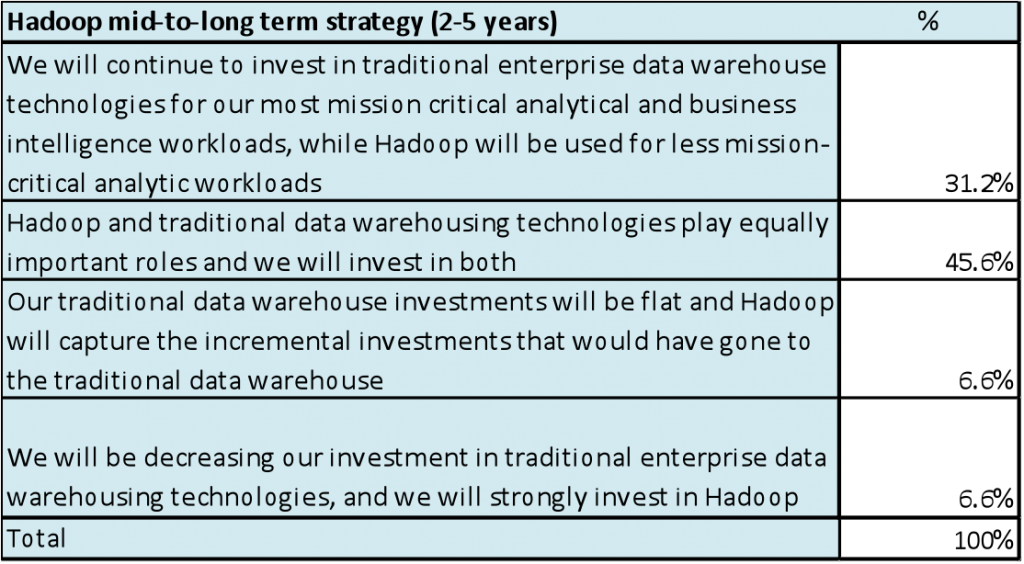
Source: Wikibon 2015
Larger (>10,000 employees) enterprises (58.8%) were more likely to embrace an “equally important role” strategy than smaller enterprises, with a “steady as you go” strategy embraced by40.5%. Smaller enterprises tended to position Hadoop more for “less mission critical analytics workloads” at 45% – a more cautious role – than did larger enterprises at 31.4%.
Satisfaction with Hadoop
Satisfaction levels were relatively high – with 10.4% stating they were only “Somewhat satisfied” and only 1 respondent reporting being “Somewhat Dissatisfied”. 95% reported a Net Recommender score of >80%.

Source: Wikibon 2015
Open source vs. commercial Hadoop distributions: commercial adoption grows with production deployments
Between Spring 2014 and Fall 2015, there was a very significant trend away from reliance on free Hadoop distributions to paid subscriptions. As Big Data Analytics becomes more integrated into operational applications, enterprises are becoming more reliant on vendors who can provide quality tools and support for these key systems of intelligence. 72% of companies with >10,000 employees are using paid distributions vs. 64% of those <10,000. However, both groups are moving from free distributions to paid distributions at a similar rate.
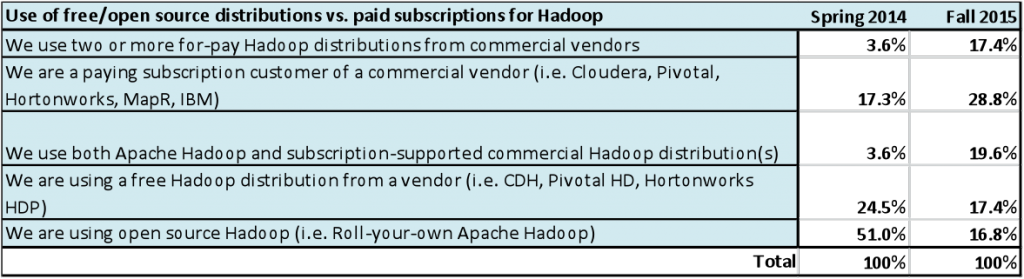
Source: Wikibon 2015
Spark plans and experience relative to Hadoop: Spark’s rollout in production applications is well behind but carries high expectations
Spark is still in early days with only 6.9% of enterprises having at least 1 Spark deployment in production. 74.0%, however, are evaluating or have Spark in a pilot/proof-of-concept. Respondents were very optimistic about Spark’s place in their future plans. 78% said they expect Spark to substitute for some of the new workloads that they would have put on Hadoop processing engines such as Hive. Fully 20% said they expect for Spark to substitute for a significant amount of the new workloads that they would otherwise have put on Hadoop engines.
Part of the optimism around Spark is probably because it is still in its honeymoon phase. The inevitable teething problems that come with production and scale will likely crop up more frequently in the future.

Source: Wikibon 2015
Unsurprisingly, over half of respondents reported using Spark’s SQL libraries, with Streaming coming in just slightly behind it. That there is so much use of all 4 libraries indicates that many applications really are leveraging the increasing integration among the libraries.
Plans for public cloud
There is significant use of Public Cloud for Big Data Analytics – 74% of respondents say they are doing some production work in the cloud. Equal parts are using Hadoop as well as native services. We defined native services using AWS examples such as Data Pipeline, Kinesis, DynamoDB, Redshift and their counterparts on Google’s Cloud Platform and Microsoft’s Azure. Some users reported using both methods. While the question was formulated slightly differently in Spring 2014, it appears that overall public cloud use increased by 5% in our 2015 study.

Source: Wikibon 2015
Hadoop isn’t a product but a rapidly evolving, innovative ecosystem. The trade-off is that there is a relatively high administrative overhead in the form of new and specialized skills. Part of our hypothesis is that as Hadoop deployments move into the mainstream, smaller and mid-size enterprises (<5,000 employees) will be more inclined to deploy it in public clouds. Not only do we expect the share of cloud-deployed Hadoop to grow, but also the share of native cloud services.
Large enterprise Hadoop users tend to be doing more production work in the Public Cloud than others and have equal rates of native public cloud services use vs. non-Hadoop users. We believe large enterprises have the skills to take them further along the path to production deployments, whether on-premises or in the cloud. Over time we expect small and mid-size enterprises to deploy a higher share of their workloads to the public cloud because it is less operationally demanding.
Users of public cloud cite the fact that data is already in the cloud (58.0%) and/or that Public Cloud offers operational simplicity (53.8%). 44.5% believe that their provider can give them a better set of tools to more easily build end-to-end applications.
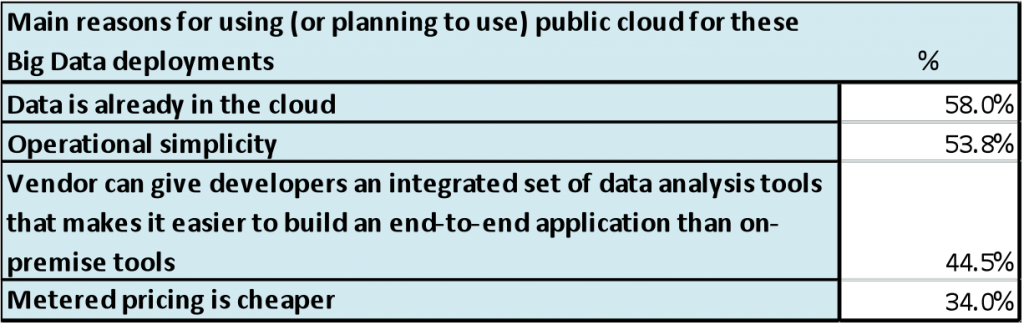
Source: Wikibon 2015
Disconnect between IT and business users over current and future public cloud plans
Business Analysts and users (36.1%) report a higher level of use of native services than Infrastructure Admins and Data Scientists (30.6%). This gap, though not large, probably comes from the fact that the business side of organizations are making use of public clouds while the IT department doesn’t even know about it. We expect this gap to widen.
Technical staff are more likely (22.4%) to report they don’t or will not use public cloud for their Big Data Analytics projects. Business staff, on the other hand, are more open to using Public Cloud (only 13.6% say they don’t or will not be using public cloud. This may be a bias towards “do-it-ourselves” on the part of technical staff.
Methodology and Demographics
Wikibon conducted a web-based survey of 303 Big Data analytics practitioners in the US in Fall 2015. Survey respondents were asked to characterize their understanding of Big Data analytics at the outset of the survey. Those who responded they were at least “somewhat familiar” or “very familiar” with Big Data analytics, as defined below, were asked to proceed with the survey.
For the purpose of this study, we defined Big Data analytics projects as those that:
- Leverage non-traditional data management tools and technologies such as Hadoop, NoSQL, or MPP analytic databases, and/or …
- Involve the analysis of multi-structured and/or unstructured data such as clickstream, text, log file, and social media data
- Big Data projects, for the purpose of this survey, do not include projects solely involving the use of relational databases or otherwise “traditional data management technologies” to collect, process, store and analyze structured data associated with legacy systems such as CRM and ERP applications
The survey further asked respondents to identify which industry they worked in, their role in the enterprise generally and role specific to Big Data analytics projects, employee count and annual revenue of their enterprise. Wikibon obtained a broad distribution of enterprise types, led by IT technology providers at 21%,manufacturing at 18%, healthcare at 14%, banking & finance at 11%, and retail at 10%.
The median company size was between $100 million and $500 million in annual revenue with between 1,000 and 5,000 employees. 23% had between 5,000-9,999 employees and 14% had over 10,000.
Respondents’ level of responsibility ranged from managers to c-level executive. Respondents were also asked to identify their roles related to Big Data analytics projects by selecting one of the following personas:
- Business users (i.e. a line-of-business professional who uses dashboards and other visualizations to understand Big Data) were 19%.
- Business analysts (i.e. a departmental power-user who conducts analysis of various Big Data sets with tools such as Excel and SPSS) were 24%.
- Application developers (i.e. a developer who builds applications that leverage Big Data analytics such as predictive models and algorithms 13%.
- Data scientists (i.e. an advanced analytics professional who conducts sophisticated analytics and develops predictive models/algorithms on large volumes of “messy” Big Data) were 16%.
- Infrastructure administrators (i.e. a datacenter professional who manages infrastructure and hardware associated with Hadoop, NoSQL database and other technologies that support Big Data analytics projects) were 28%.
Based on the respondent profile and their understanding of Big Data analytics, it is clear that the resulting analysis represents the state of Big Data analytics among relatively early adopters. This is an inevitable result of studying this topic. With Big Data analytics technologies and approaches still relatively immature, those enterprises and practitioners that are evaluating or have deployed Big Data analytics projects are by definition early adopters. This is an important piece of information to keep in mind when considering the results of the survey.
Wikibon compared respondent data from its Spring 2014 research with the Fall 2015 results on a number of questions. We drew our 2015 sample lists from the same sources as we used in 2014, so we believe the differences in responses between the two years are fairly reflective of changes in attitudes, plans, and experiences of Big Data users over the 18 month period.