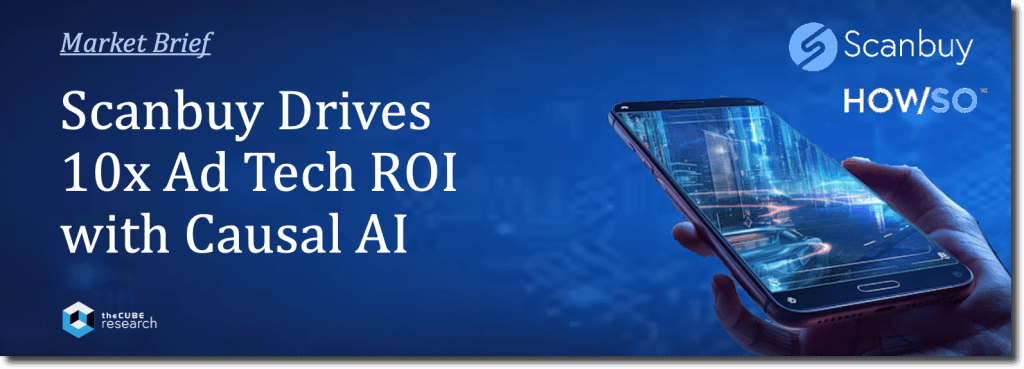
At theCUBE Research, we focus on keeping you informed about the latest developments shaping the future of AI and helping you prepare now rather than later.
This brief continues our research into the emerging impact of causal AI and explains why it is necessary and an inevitable ingredient in designing agentic AI systems.
This is the first of several market briefs that highlight the impact of causal AI in real-world use cases. It showcases how Scanbuy, a leader in mobile advertising, and its partner, Howso, a pioneer in AI understandability platforms, have set a new standard for trust, transparency, and AI-driven ROI in programmatic advertising.
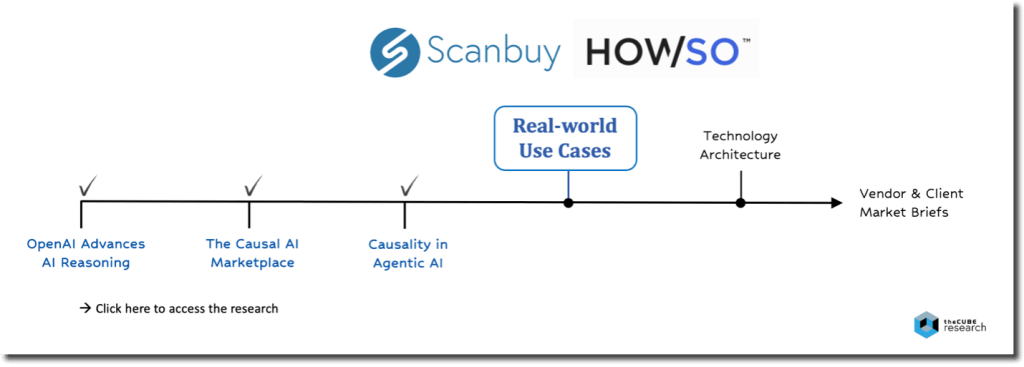
Causal AI Overview
While today’s generative AI is quite impressive, it is missing one of the most important ingredients in the business decision-making process: causality.
Causality will enable businesses to do more than create predictions, generate content, identify patterns, and isolate anomalies. They’ll also be able to play out countless scenarios to understand the consequences of various actions, explain the causal drivers of their business, and analytically problem-solve. That is, they’ll know WHAT to do, HOW to do it, and WHY certain actions are better than others to shape future outcomes. This is key because predicting something is less valuable than knowing why something happened and what can be done differently to improve an outcome.
Simply put, humans are causal by nature, so AI must also become causal by nature.
To solve problems, AI must understand “cause and effect” relationships and weigh the consequences of possible actions, just like humans do.
Furthermore, causal AI can understand more precise pathways to an outcome and tell you how variables interacted, their values, and how much they influenced it. This helps to open up the “black box” of today’s AI, providing greater trust, transparency, and explainability in AI recommendations and outcomes.
We believe AI systems will rapidly mature in the years ahead by infusing progressive degrees of causal reasoning capabilities. These systems will be much better equipped to deliver on the high ROI promise of Agentic AI, which you can read about here.
Learn more about the concepts and benefits of causal AI here.
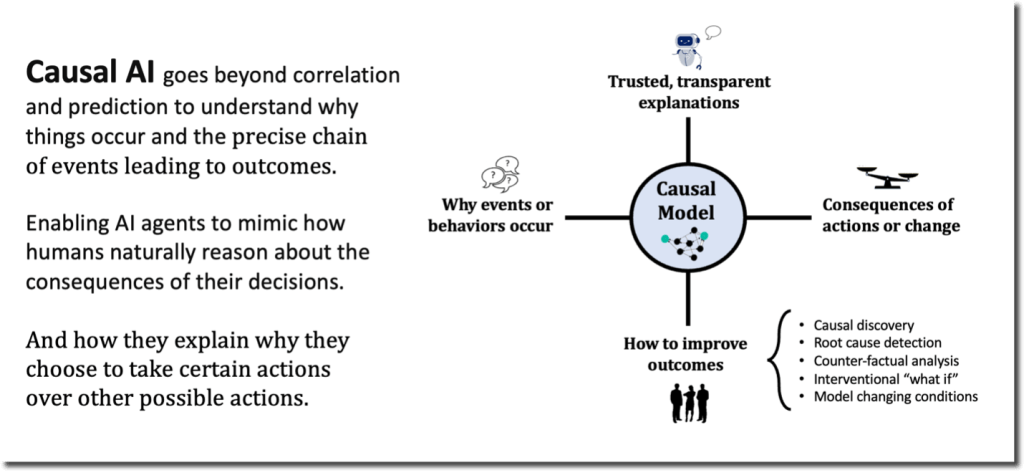
Ad Tech ROI
This causal AI use case explores how Scanbuy, a leader in mobile advertising and marketing solutions for over 300 of the world’s top brands, has partnered with Howso to usher in a new era of trust in AI-driven advertising. As a result, it achieved a 10x ROI in mobile advertising campaign performance:
- 10x improvement in campaign performance without any additional spending
- 900K lookalike dataset with similar performance to that of a deterministic dataset
- Greater transparency into the influences and attributes that driveo effective campaigns
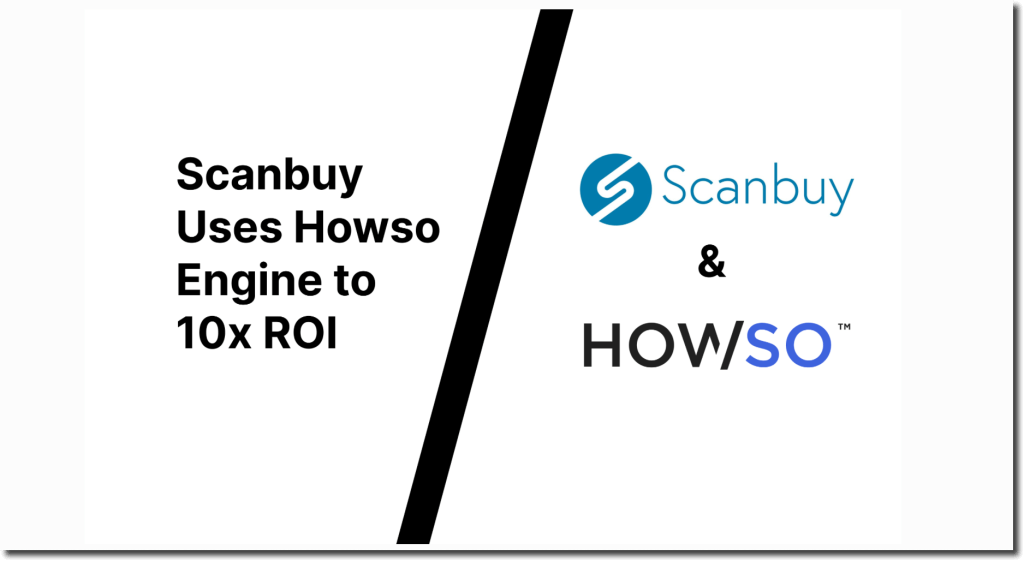
Delivering Trust in Ad Tech
Trust has become the currency of success in the evolving landscape of programmatic advertising. As AI-driven models increasingly dictate advertising strategies, the need for transparency and accountability has never been more critical.
The recent discourse among industry leaders highlights a pervasive trust deficit in AI systems, exacerbated by opaque algorithms and inconsistent data practices.
Programmatic marketers everywhere are grappling with myriad challenges, from the deprecation of third-party cookies to the complexities of AI-driven decision-making.
For example, as advertisers scale their target audiences, only about 10% come from deterministic datasets, while 90% are probabilistic. These probabilistic audiences are typically generated via “black box” predictive AI models, such as LLMs, which cannot explain themselves and are prone to biases and inaccuracies.
As a result, agency executives are expressing a lack of understanding and trust in AI, fearing that poor data inputs will lead to suboptimal outputs, increased fraud, and new regulatory compliance challenges. It’s not just agencies that are concerned; brands are increasingly aware of the trust gap, as are other actors in the ad tech value chain.
As Marc Le Maitre, Scanbuy’s Chief Technology Officer, stated,
“We operate in a data enrichment sector that is increasingly regulated, such as with the Digital Services Act in Europe, and we need to find new ways to bring AI transparency and explainability to the industry. If we don’t, using AI in advertising will deliver diminishing returns.”
Causality in Programmatic Advertising
The call for transparency is loud and clear; however, the path to achieving it remains elusive. This is why Scanbuy partnered with Howso, a pioneer in making AI understandable, to employ causal AI to help programmatic advertisers:
- Understand the causal drivers of effective advertising
- Generate lookalike audiences that are open and auditable
- Make real-time adjustments and model hypotheticals (what if)
- Provide meaningful information about ad creation
- Simplify regulatory compliance reporting
Drawing inspiration from the trusted On-Board Diagnostics (OBD) systems in vehicles, which self-report faults and allow for deep inspection, Scanbuy has adopted a similar approach to employ causal AI as the “check engine light” in the ad tech space. By leveraging the Howso instance-based learning theory alongside its causal AI capabilities, Scanbuy has provided advertisers with a transparent, auditable model for monitoring trust metrics in AI-driven campaigns.
Unlike traditional black-box models in today’s LLMs, Howso‘s approach delivers unparalleled transparency. It also enables real-time adjustments and hypotheticals to ensure that AI models remain aligned with evolving consent and regulatory requirements. Howso‘s perfect attribution traces decisions back to the source data, enabling complete audibility and fostering a culture of accountability and trust.
For example, with new counterfactual analysis capabilities, advertisers can explore “what if” scenarios, gaining insights into decision-making paths and potential outcomes. They can also enable real-time adaptability to advertising models by fine-tuning “on the fly” while ensuring compliance with the latest changes in regulatory guidelines and consumer consent. As a result, Scanbuy can probabilistically scale target audiences by up to 10x without degrading engagement rates, which typically experience up to 50% decay when using “black box” AI models that lack causality and full transparency.
The net outcome is that causal AI techniques helped to drive a breakthrough 10x ROI by achieving the same deterministic audience campaign success rate with a 10x larger audience, with no additional incremental campaign spending.
More strategically, Marc Le Maitre sees a broader industry-wide impact:
“We’re using LLMs and gen AI to explain the outcomes based on the causal models at the atomic level with almost no hallucination. The combination is fantastic. Causal AI is what we are flying our flag on. It’s exactly what the industry needs. It can tell us what caused an ad to be effective or rejected by their target audiences and help us determine if the advertising model is transparent enough to create benchmarks for audibility.”
This makes a ton of practical sense. For example, the European Digital Services Act says that when serving ads, you must show what caused this ad to be served to its target audience, what meaningful information was used to create it, and that you must give the recipient the opportunity to make changes to that ad when appropriate. There is no framework today on how to do that, which is becoming a critical need for digital advertising players. Causal AI may represent the keys to demonstrating how auditable programmatic advertising can be across the algorithmic and decision-making process. Causal AI offers new levels of AI model integrity.
Marc’s view:
“If you don’t do this, you end up with model collapse or dementia.
His experience is that Causal AI enables meaningful reinforcement learning with humans in the loop to improve ad effectiveness by understanding causal drivers of action and understanding the pathways to better outcomes. When a user sees an advertisement they don’t like, the causal model can understand why and rebuild itself to be better.
“This is transformational if applied correctly. Creating transparent and explainable open-lookalike advertising models will transform the industry and likely reduce regulatory friction. It will democratize the industry at its core.”
The innovation at Scanbuy, in partnership with HowSo, promises to set new standard for transparency and trust in programmatic advertising by embracing causal AI. Just as Onboard diagnostics (OBD) systems have become a trusted standard in automotive diagnostics, Howso‘s approach to AI offers advertisers a reliable, transparent framework for navigating the complexities of modern ad tech. As the industry moves forward, those who prioritize trust and transparency will lead the way in delivering meaningful, compliant advertising experiences.
Based on their success, Scanbuy is now working with the IAB Tech Lab to position its approach to create industry standards for open and auditable AI advertising. This initiative would ease the complex regulatory landscape and foster greater trust among consumers and advertisers.
Read the full Scanbuy use case and download the Howso Causal AI starter kit to learn more.
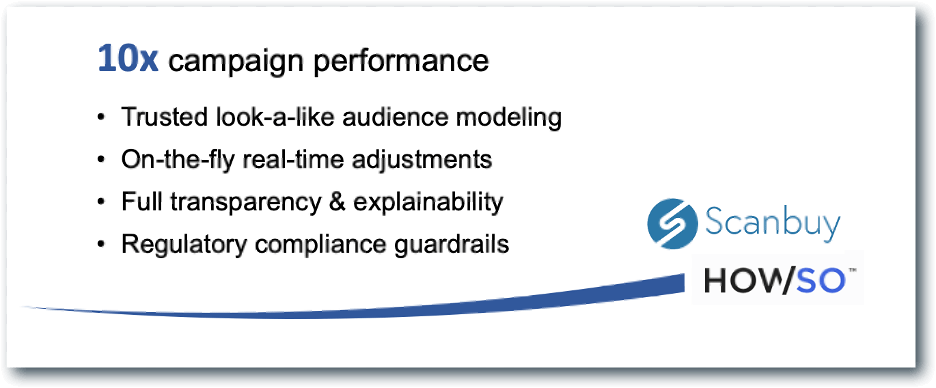
The Causal AI Marketplace
The emerging Causal AI marketplace aims to address the limitations of current LLMs and generative AI solutions. This is particularly crucial for high ROI use cases that rely on root cause detection, decision intelligence, problem-solving, and autonomous action. AI professionals working on these cases recognize that integrating causality is essential for modeling the dynamic business environment and enhancing AI trust and explainability.
The marketplace is speaking louder and louder.
A survey of 400 senior AI professionals provided by Databricks showed that among AI pioneering companies, 56% were already using or experimenting with Causal AI. In addition, among the total population of the survey, Causal AI was ranked as the #1 AI technology “not using, but plan to next year.” The study reported that 16% are already actively using causal methods, 33% are in the experimental stage, and 25% plan to adopt. Overall, 7 in 10 will adopt Causal AI techniques by 2026.
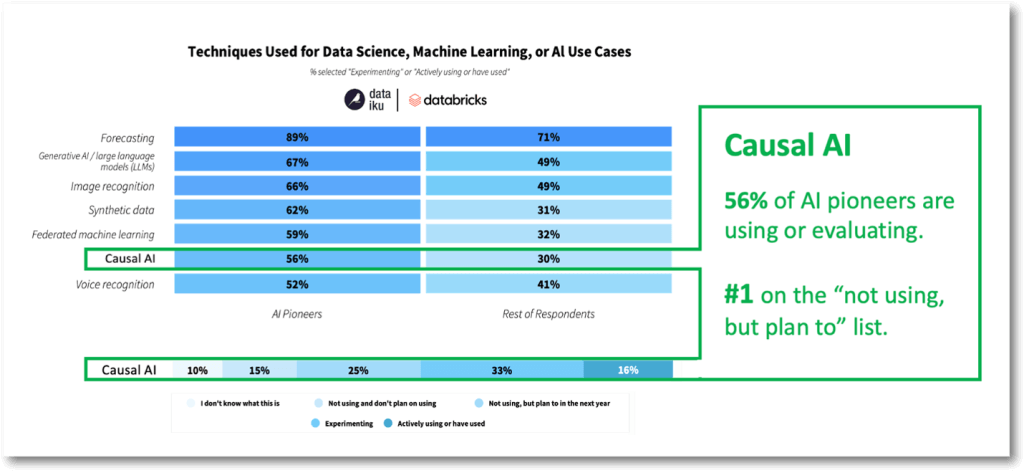
The resulting commercial marketplace is somewhat ill-formed, but we expect it to solidify and rapidly expand from here. The consensus view of six independent market studies complied by theCube Research indicates a projected 41% CAGR through 2030 to around a $1 billion marketplace.
Our view is that when assessing this marketplace, we must consider the following:
- In-house engineers are adopting and customizing open-source causal AI libraries.
- App vendors are building causal AI methods into their products and services.
- AI heavyweights like Meta, Google, and OpenAI are active in causal AI research.
For these reasons, we believe the aggregate market impact of causal AI on business will be far greater than the $1B marketplace indicated by today’s studies. In fact, we would not be surprised to see a similar dynamic to how generative AI well outperformed its initial market growth projections and became the darling of the AI world.
You can read more about the emerging Causal AI Marketplace here.
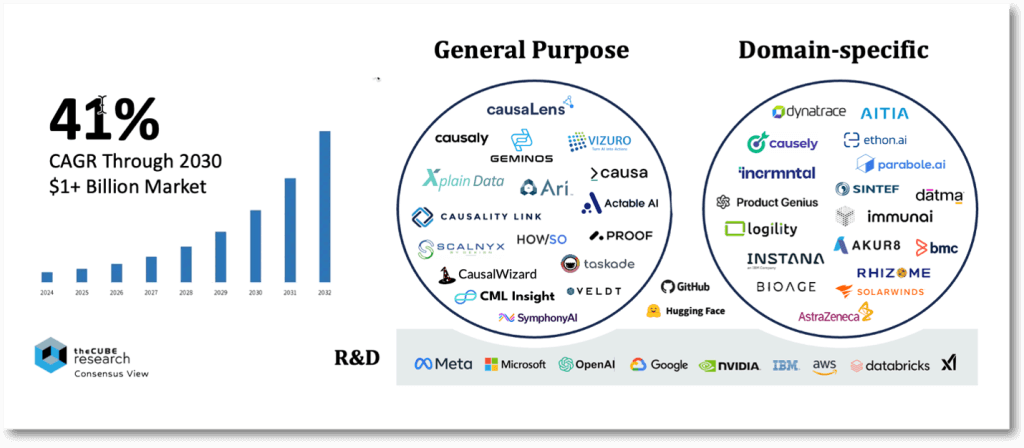
What to Do and When
The Scanbuy use case illustrates one of the countless ways in which causal AI will reshape how businesses solve complex problems. Stay tuned for a series of market briefs that will explore additional high-ROI use cases.
Our view here at theCUBE Research is that as the world of Agentic AI matures, causality will become an inevitable part of future-state AI models, as the limitations of correlative-based AI will eventually hinder innovation.
Perhaps the time is now to start preparing. We’d recommend you:
- Build competency in both agentic and causal AI
- Evaluate the impact of causality on your use cases
- Download the Howso causal AI starter kit
- Experiment with the technology and build talent
We also recommend checking out the following research:
Thanks for reading. Feedback is always appreciated.
As always, contact us if we can help you on this journey by booking a briefing here or messaging me on LinkedIn.
