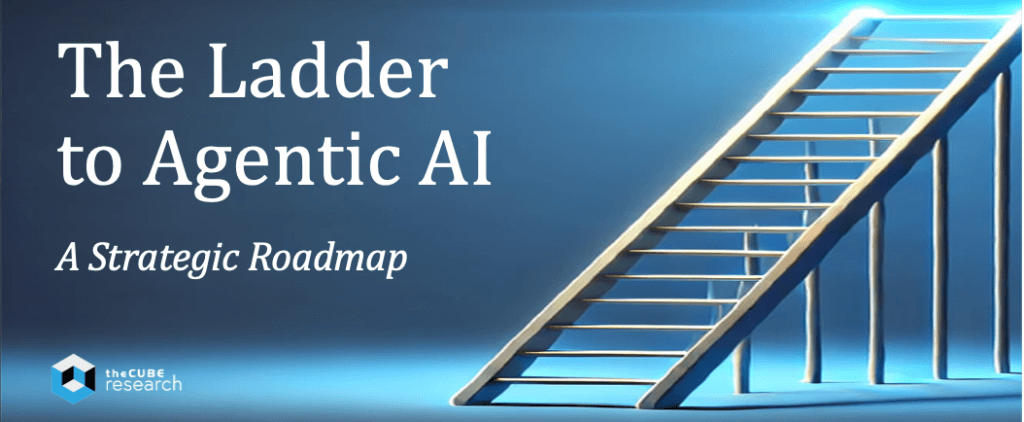
Here at theCUBE Research, we are dedicated to keeping you updated on the latest developments shaping the future of AI and helping you stay informed about emerging technologies, use cases, and real-world ROI.
We believe that combining agentic AI, new AI reasoning technologies, and domain-specific SLMs (where “S” stands for attributes like small, specialized, and sovereign) will accelerate ROI realization in the coming years.
In this research note, we’ll synthesize insights from numerous discussions with industry pioneers and experts already deriving value from agentic AI investments.
Our interviews clearly indicate that the rise of Agentic AI signifies a fundamental shift in AI, evolving from task-oriented automation to intelligent, goal-driven decision-making. Beginning in 2025, businesses will embrace AI agents that can learn, adapt, and autonomously perform tasks while operating within coordinated systems.
However, they also suggest that the pathway to achieving fully functional Agentic AI remains uncertain for many organizations. Our research indicates that 8 out of 10 enterprises plan to deploy agentic systems, yet only 1 in 3 understand the strategic roadmap that lies ahead.
We’ll outline a high-level strategic roadmap from our learnings, metaphorically called the “ladder to agentic AI.” This research will present a structured approach to developing Agentic AI, breaking it down into distinct phases, or “rungs,” on a conceptual ladder.
- Rung #0 — Foundational Fine-Tuning
- Rung #1 — Domain Knowledge
- Rung #2 — Explainable Decision Intelligence
- Rung #3 — Intelligence Actors
- Rung #4 — Agentic Teamwork
- Rung #5 — Act, Learn, Adapt
By ascending this ladder, organizations can progress from basic AI assistance to goal-driven AI systems that transform decision-making and how business processes adaptively operate.
Watch the Podcast
Join theCUBE Research’s George Gilbert and Scott Hebner as they outline the ladder to Agentic AI in a recent episode of the Next Frontiers of AI podcast.
Ground Floor – The Starting Point
Before we progress through each “rung” on the ladder to agentic AI, let’s first provide some perspective on the current state of the enterprise AI landscape.
After two years of increasing investment, Gen AI and LLMs have become well-established in most enterprises. Nearly half of the white-collar workers now use Gen AI to explore new ideas (42%), consolidate information (36%), and automate basic tasks (36%), according to an “AI in the Workplace” survey by Gallup. The widespread adoption within businesses is also supported by the Enterprise Technology Research (ETR) tracking surveys, finding that 74% have deployed Gen AI.
Notably, fewer than 15% are using AI for decision intelligence, adaptive workflow automation, or to help solve complex problems. As a result, quantified ROI has proven elusive, appearing more implicit than explicit, leading to a continual shift in ROI expectations for the future. Overall, just 18% reported a high measurable value, according to a recent Data & AI Leadership Exchange survey.
Generative AI and LLMs have democratized the benefits of AI for everyone. We are currently witnessing a notable shift in focus toward utilizing Gen AI to cultivate higher-value use cases centered on decision intelligence and more autonomous workflows that aim to equip workers with new “superpowers.”
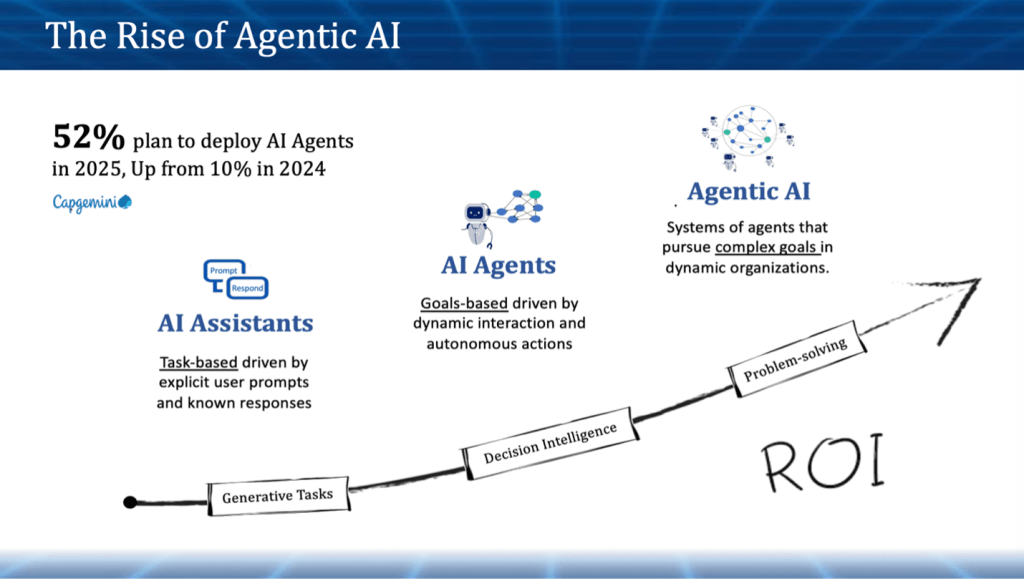
This shift will require a reevaluation of the technological landscape, as the current technologies most enterprises use will be insufficient to deliver the potential value of agentic use cases. Companies are increasingly aware that LLMs alone risk becoming incubators for real-world issues and are simply insufficient for producing the high ROI use cases they seek, particularly those associated with business-critical decisions.
Consequently, we anticipate a shift as many enterprises enhance and complement LLMs with more advanced model ecosystems and software architectures. Rather than relying solely on LLMs, multi-model AI architectures will become vital in driving a new generation of agentic AI use cases.
In 2024, the consensus view from various studies evaluated by theCUBE Research suggests that only 10-20% of enterprises have deployed such architectures. For example, the current adoption rates of domain-optimized knowledge models and AI reasoning technologies will need to accelerate to create the underlying model ecosystem to fuel AI agents.
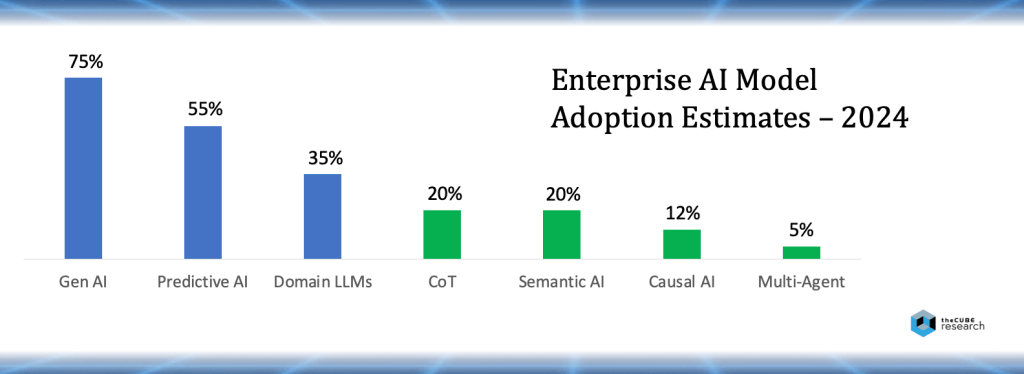
GenAI and LLMs have undoubtedly created a “gateway” to AI and laid a crucial foundation for future agentic AI applications. However, the real competition will shift towards developing integrated AI model ecosystems that address the limitations of today’s AI and unlock countless new opportunities through AI decision intelligence and dynamic agent collaboration.
Companies that recognize this change early—by concentrating on building value on these GenAI platforms—will pave the way for AI innovation and ROI. That is what the ladder to agentic AI represents.
Rung #0 – Foundational Fine-Tuning
At the base of the ladder lies foundational AI, which includes large language models (LLMs) and generative AI. These models form the backbone of modern AI capabilities, enabling automation, prediction, and content generation.
Within this “rung,” organizations should standardize on one or more pre-trained models, such as OpenAI’s GPT, Google’s Gemini, and/or Anthropic’s Claude, to provide general-purpose AI capabilities and act as a “gateway” into the global “web” of AI knowledge. This foundation will enable agents to process unstructured data, analyze information, generate responses, and automate tasks.
Organizations should utilize Retrieval-Augmented Generation (RAG) to align LLMs with their business by integrating real-time, proprietary data into AI-generated responses. Consider this as implementing the unique language of your business. RAG enhancements will improve contextual accuracy by retrieving relevant internal knowledge and ensuring responses align with business terminology and compliance requirements. Citing sources and using role-based prompts can also make responses clearer. Think of the LLM foundation as the cognitive core. Additional AI models refine their goal-setting, decision intelligence, reasoning, autonomy, and adaptability to drive real-world decision-making automation.
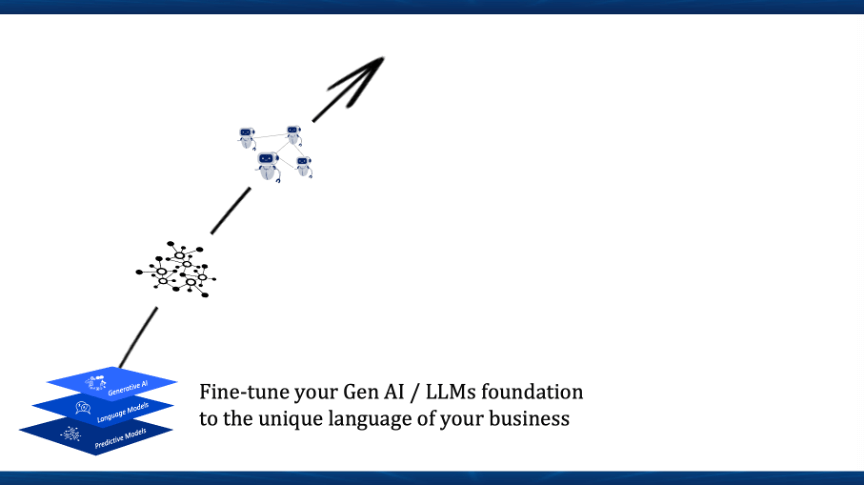
Rung #1: Domain Knowledge
To move beyond foundational AI, organizations must climb the first rung of the ladder by integrating domain intelligence into their AI models. This strategy will strengthen LLMs by providing the added depth of knowledge required to understand the specific language, workflows, and nuances of a business process
Organizations should start by refining their models with domain-specific data. They should train their models on proprietary datasets to improve their understanding of industry-specific terminology, regulations, and workflows. For example, a bank can enhance an AI model using regulatory compliance documents and transaction data to strengthen fraud detection and risk management.
Another crucial component is the use of knowledge graphs, which organize data to illustrate semantic relationships between entities. This helps AI agents grasp context and meaning. This capability is vital when managing complex interdependencies, such as legal contracts, where AI must understand the relationships among vendors, regulations, and contract clauses to provide relevant insights. Graph-RAG can also retrieve relevant data into a knowledge graph, mapping relationships between entities to offer more context-aware and logically coherent responses, improve explainability, and reduce inaccuracies.
By developing an ecosystem of specialized domain knowledge models around foundational LLMs, organizations can unlock the real-world expertise and knowledge within their structures. AI agents that understand the context and significance of entities in a use case will become more valuable, adaptable, and trustworthy. Consequently, this will pave the way for integrating decision-making capabilities within the agentic AI model ecosystem.
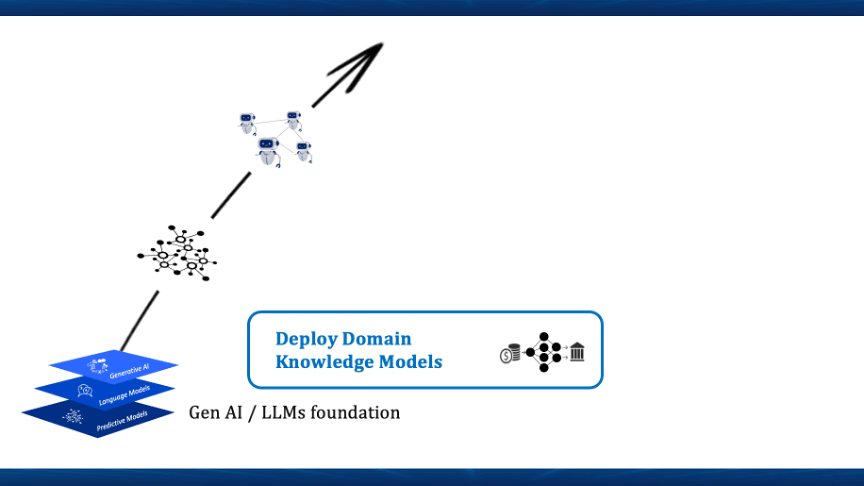
Rung #2: Explainable Decision Intelligence
Integrating explainable decision intelligence is the next crucial step in the journey toward AI agents that can truly assist humans in planning and problem-solving. This development will enable AI agents to explore countless scenarios, understand the ramifications of various decisions, tackle complex problems, and gain clearer insights into the optimal pathways for achieving a goal.
Additionally, it will promote a greater degree of trust in allowing AI agents to operate autonomously when human intervention is impractical, costly, or unnecessary. With the real-time decision intelligence provided by AI reasoning, AI agents will be capable of understanding not only WHAT to do but also HOW to do it and WHY certain actions are preferable to others. This is essential for the advancement of agentic AI systems.
Generally, organizations can incrementally enhance their reasoning capabilities by incorporating models into the ecosystem using:
- Chain-of-Thought: Also known as self-taught reasoning, to dissect problems into logical steps that are dynamically assessed and refined to arrive at a solution for a given question.
- Semantic Reasoning: to interpret the underlying context, relationships, and concepts in data to process the knowledge required to complete tasks. This can be accomplished by integrating knowledge graphs or with neural symbolic AI that incorporates graphs within AI models.
- Causal Reasoning: AI that comprehends how and why events occur, the consequences of actions, and the causal mechanisms leading to outcomes. By incorporating Causal AI models into the AI model ecosystem, the agents can grasp cause-and-effect relationships and the exact sequence of events that result in an outcome. These technologies also facilitate counterfactual reasoning, simulating “what-if” scenarios to effectively identify the optimal pathway to a desired result.
- Multi-modal Reasoning: AI systems in which multiple agents or AI reasoning models share knowledge, coordinate actions to achieve a common goal, and learn interactively from human feedback as well as the consequences of their decisions.
Integrating decision intelligence and genuine explainability will inevitably become essential for enabling AI agents to make actionable and trustworthy recommendations. Most importantly, they must be explainable so that humans can trust them.
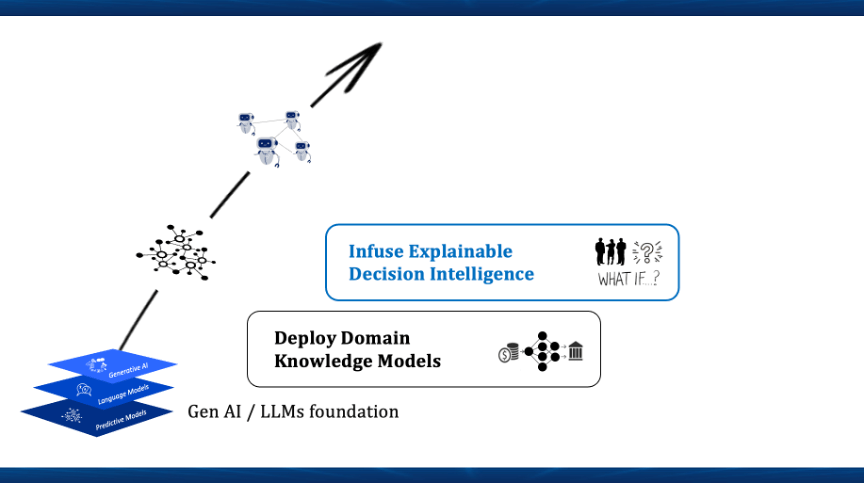
Rung #3: Intelligent Actors
Once an organization has developed a fundamental AI model ecosystem that provides domain knowledge, decision intelligence, and explainability, the next step is to create AI agents that utilize those models. Organizations can build custom AI agents tailored for specific use cases or purchase pre-built agents from AI marketplaces and modify them to suit their unique needs. The goal is to establish a portfolio of agents that can operate independently or connect to collaborate within an agentic system.
This involves using a platform designed for creating AI agents, such as Sema4, IBM Watsonx, and Microsoft’s CoPilot Studio. These platforms will help define the agent’s purpose, ensure it connects to relevant AI models for advanced decision-making, and incorporate human oversight when needed. They also facilitate continuous testing and learning mechanisms to enhance the agent’s performance over time, enabling it to adapt to changing business needs and conditions.
Alternatively, organizations can acquire pre-built AI agents from platforms such as OpenAI’s GPT Store, Hugging Face, or numerous enterprise AI agentic providers. These agents come with pre-configured functionalities and can be fine-tuned using proprietary data to improve their contextual accuracy. This process involves aligning the agent with specific business use cases and linking it to internal knowledge graphs and reasoning models.
By developing or acquiring AI agents that utilize a well-organized AI model ecosystem, businesses can deploy them incrementally to address specific use cases or integrate them into agentic AI systems to tackle more complex organizational challenges. The key is selecting the right platform to build custom agents and manage their lifecycles as they adapt and improve.
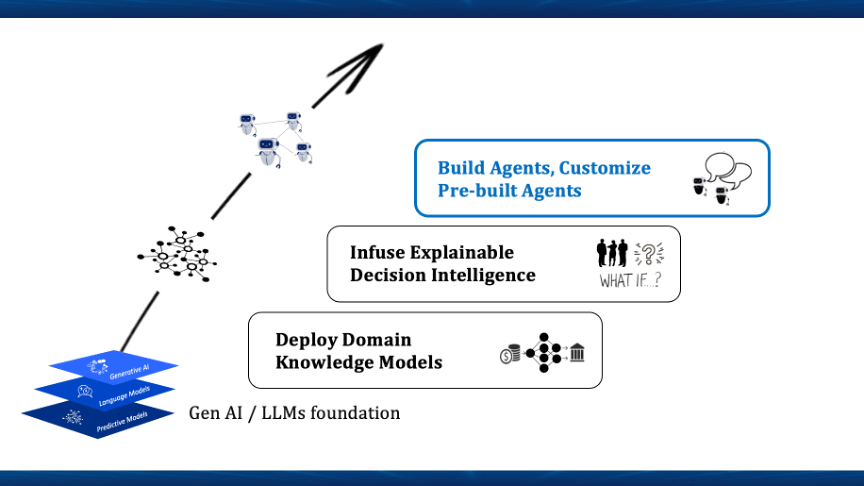
Rung #4: Agentic Systems – Teamwork
While individual AI agents provide significant value to organizations through their decision-making capabilities, even greater advantages will emerge from implementing Agentic AI systems, which assist in achieving more complex organizational goals that individual agents cannot accomplish alone.
Agentic AI systems consist of individual AI agents working together within an interconnected framework to optimize business objectives as a cohesive unit. The agents are interlinked, allowing them to dynamically interact and learn from one another, enabling them to adapt their behaviors to changing conditions. This requires organizations to empower AI agents to communicate, share insights, and allocate tasks efficiently to meet workflow goals.
A more advanced concept in Agentic AI systems is swarm intelligence, where AI agents operate collectively as a decentralized network. In this model, agents self-organize, utilizing real-time data and feedback from each other to enhance performance. Swarm intelligence promotes distributed problem-solving, adaptability, and resilience, allowing systems to function efficiently even in complex and ever-changing environments.
Businesses typically need to implement orchestration platforms, which often vary from AI agent-building platforms. These platforms enable and oversee multi-agent collaboration, decision orchestration, and hierarchical goal management alignment. Solutions like Multi-Agent Decision-Making (MADM) platforms and IBM Watson Orchestrate govern how AI agents work together, ensuring their decisions align with broader enterprise objectives. These frameworks offer structured workflows that balance autonomy and coordination to prevent conflicting AI decisions. Additionally, these platforms enforce goal alignment to ensure that each individual AI agent contributes to enterprise-wide priorities, such as revenue growth or customer retention, guided by metric trees.
When designing agentic systems, considering production-level factors is essential, as this is where orchestration becomes more dynamic and adaptive.
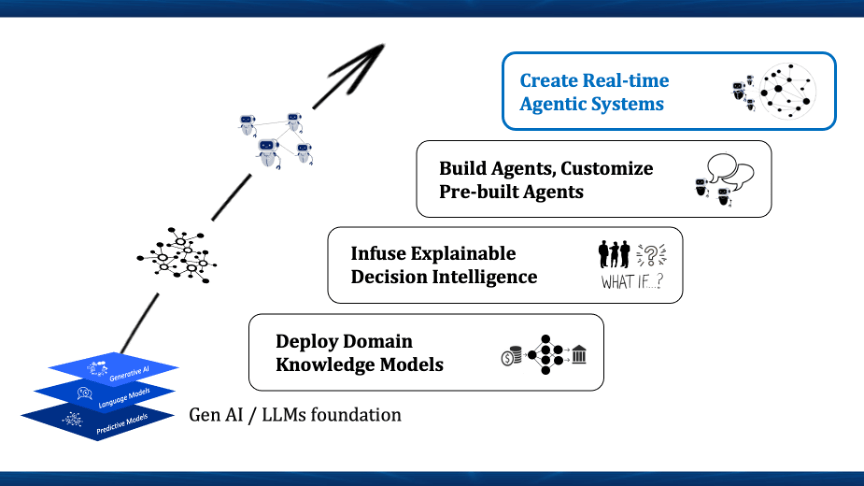
Rung #5: Learning Loop – Act, Learn, Adapt
Organizations should empower AI agents to continuously learn, adapt, and enhance their capabilities by exchanging insights and refining their decision-making in a collaborative environment. This necessitates the creation of a learning loop fueled by multi-agent collaboration, where AI agents interact, share knowledge, and collectively optimize workflows effectiveness.
A crucial step involves utilizing multi-agent reinforcement learning (MARL), where AI agents learn from their own experiences and others in a shared environment. Frameworks such as LlamaIndex or AutoGen provide the foundation for training AI agents to make collaborative decisions.
More advanced techniques are rapidly emerging in the market, such as imitation learning, which enhances the learning process by enabling AI agents to observe and emulate expert human behavior, learning from the outcomes they see and the consequences of previous actions or decisions. Additionally, those utilizing semantic and causal reasoning models can use Graph-RAG and Causal RAG, which improve AI decision-making by integrating structured knowledge and cause-and-effect relationships into multi-agent learning processes.
These techniques enable AI agents to understand the effects of their decisions instead of depending only on correlations. The true power of agentic AI systems will derive from their real-time learning loops, allowing AI agents to continuously improve their strategies in response to changing conditions and promoting self-enhancement based on real-world results.
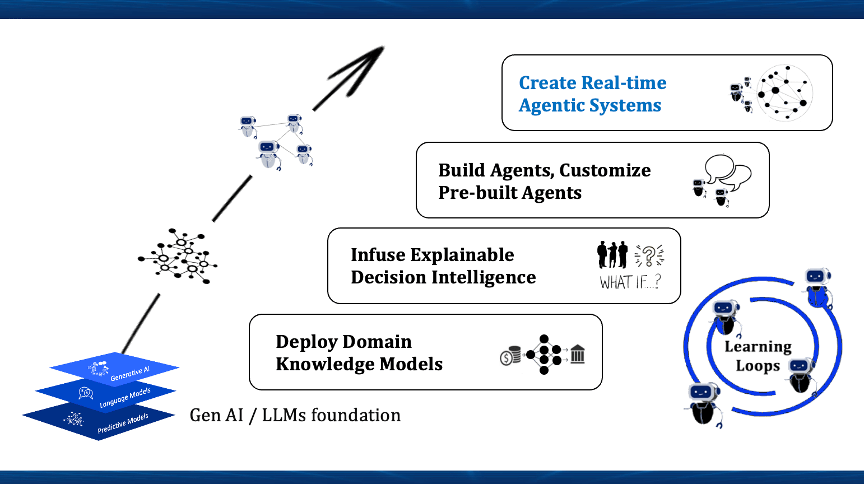
What To Do and When
The shift towards Agentic AI will not occur overnight. Enterprises must methodically ascend the ladder, integrating capabilities step by step. While LLMs and generative AI provide a necessary foundation, the ultimate aim is a network of intelligent, goal-oriented AI agents that autonomously execute business processes while learning and improving over time.
This will necessitate greater domain knowledge, decision-making intelligence, explainability, and multi-agent orchestration frameworks.
As 2025 unfolds, businesses proactively investing in the Agentic AI roadmap will gain a significant advantage by enhancing AI decision-making, automating adaptive workflows, and even creating efficiencies through talent scaling and autonomous operations. The next stage in the ROI journey is sure to follow, progressing through the development of AI agents and agentic capabilities systems.
Perhaps the time is now to start preparing for the journey that lies ahead:
- Building competency in agentic AI and AI decision-intelligence
- Evaluate use cases and how to get started incrementally
- Engage with the ecosystem of pioneering vendors and experts
- Experiment with the technology and build a talent pool
As always, contact us if we can help you on this journey by booking a briefing here or messaging me on LinkedIn.
Finally, we’d recommend watching the following podcasts:
Six Predictions for AI in 2025
Making AI decisions Explainable with Causal AI
Thanks for reading. Feedback is always appreciated.
